Going Beyond the Point Neuron: Active Dendrites and Sparse Representations for Continual Learning
biorxiv(2021)
摘要
Biological neurons integrate their inputs on dendrites using a diverse range of non-linear functions. However the majority of artificial neural networks (ANNs) ignore biological neurons’ structural complexity and instead use simplified point neurons. Can dendritic properties add value to ANNs? In this paper we investigate this question in the context of continual learning, an area where ANNs suffer from catastrophic forgetting (i.e., ANNs are unable to learn new information without erasing what they previously learned). We propose that dendritic properties can help neurons learn context-specific patterns and invoke highly sparse context-specific subnetworks. Within a continual learning scenario, these task-specific subnetworks interfere minimally with each other and, as a result, the network remembers previous tasks significantly better than standard ANNs. We then show that by combining dendritic networks with Synaptic Intelligence (a biologically motivated method for complex weights) we can achieve significant resilience to catastrophic forgetting, more than either technique can achieve on its own. Our neuron model is directly inspired by the biophysics of sustained depolarization following dendritic NMDA spikes. Our research sheds light on how biological properties of neurons can be used to solve scenarios that are typically impossible for traditional ANNs to solve.
### Competing Interest Statement
The authors have declared no competing interest.
更多查看译文
关键词
continual learning,active dendrites,sparse representations,point neuron
AI 理解论文
溯源树
样例
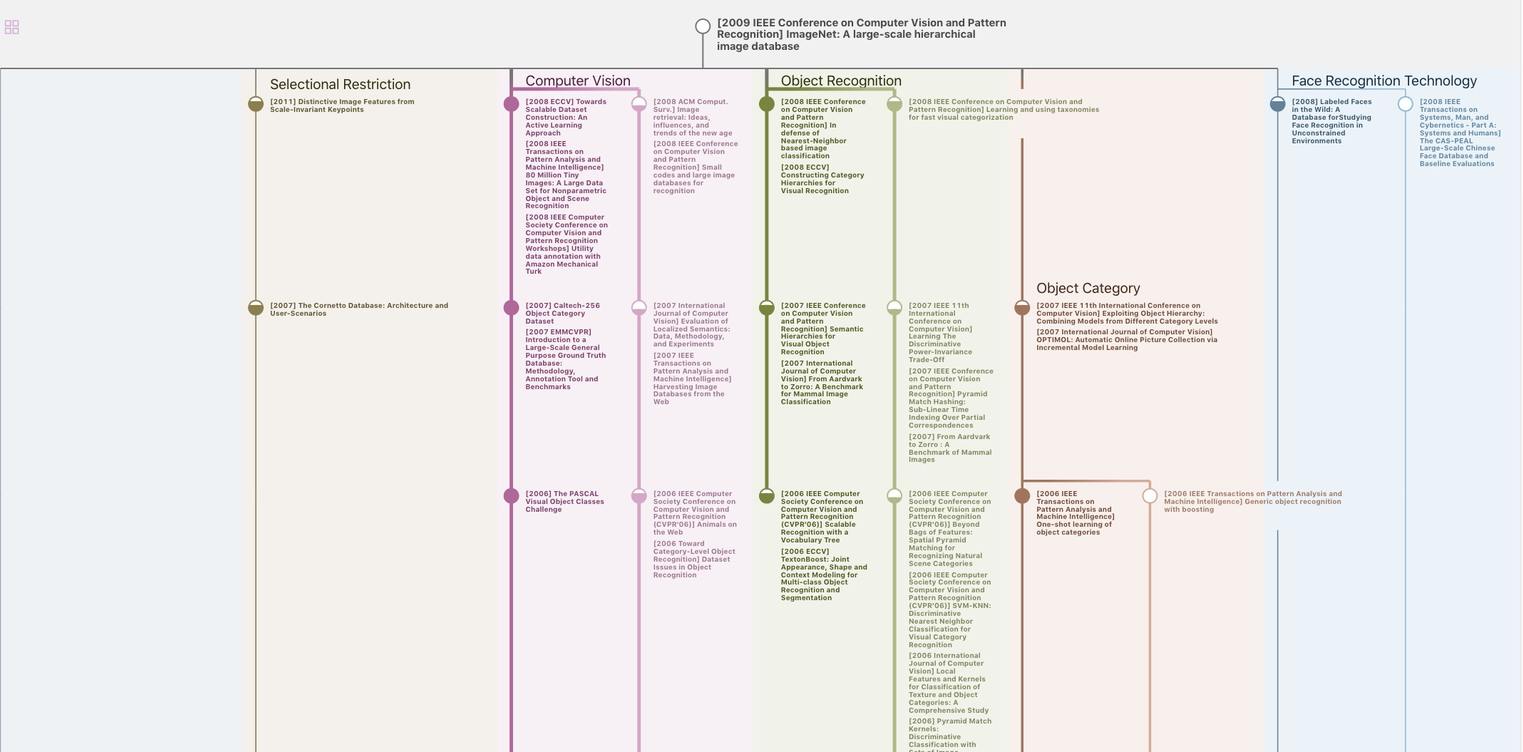
生成溯源树,研究论文发展脉络
Chat Paper
正在生成论文摘要