PyMVPD: A Toolbox for Multivariate Pattern Dependence
FRONTIERS IN NEUROINFORMATICS(2022)
摘要
Cognitive tasks engage multiple brain regions. Studying how these regions interact is key to understand the neural bases of cognition. Standard approaches to model the interactions between brain regions rely on univariate statistical dependence. However, newly developed methods can capture multivariate dependence. Multivariate pattern dependence (MVPD) is a powerful and flexible approach that trains and tests multivariate models of the interactions between brain regions using independent data. In this article, we introduce PyMVPD: an open source toolbox for multivariate pattern dependence. The toolbox includes linear regression models and artificial neural network models of the interactions between regions. It is designed to be easily customizable. We demonstrate example applications of PyMVPD using well-studied seed regions such as the fusiform face area (FFA) and the parahippocampal place area (PPA). Next, we compare the performance of different model architectures. Overall, artificial neural networks outperform linear regression. Importantly, the best performing architecture is region-dependent: MVPD subdivides cortex in distinct, contiguous regions whose interaction with FFA and PPA is best captured by different models.
更多查看译文
关键词
multivariate pattern dependence, connectivity, fMRI, deep networks, toolbox
AI 理解论文
溯源树
样例
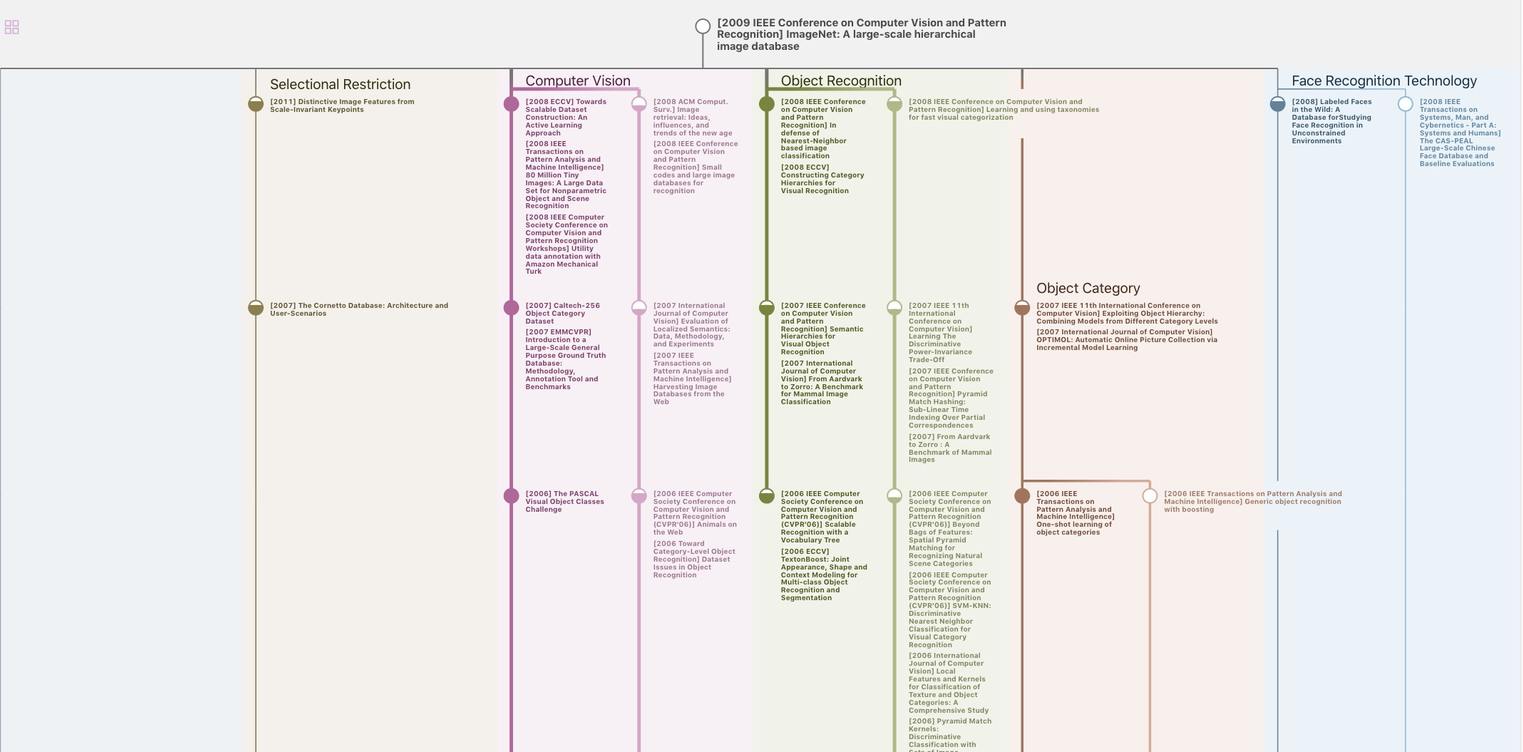
生成溯源树,研究论文发展脉络
Chat Paper
正在生成论文摘要