Accelerating Identification of Chromatin Accessibility from noisy ATAC-seq Data using Modern CPUs
biorxiv(2021)
摘要
Identifying accessible chromatin regions is a fundamental problem in epigenomics with ATAC-seq being a commonly used assay. Exponential rise in single cell ATAC-seq experiments has made it critical to accelerate processing of ATAC-seq data. ATAC-seq data can have a low signal-to-noise ratio for various reasons including low coverage or low cell count. To denoise and identify accessible chromatin regions from noisy ATAC-seq data, use of deep learning on 1D data – using large filter sizes, long tensor widths, and/or dilation - has recently been proposed. Here, we present ways to accelerate the end-to-end training performance of these deep learning based methods using CPUs. We evaluate our approach on the recently released AtacWorks toolkit. Compared to an Nvidia DGX-1 box with 8 V100 GPUs, we get up to 2.27× speedup using just 16 CPU sockets. To achieve this, we build an efficient 1D dilated convolution layer and demonstrate reduced precision (BFloat16) training.
### Competing Interest Statement
All authors are employees of Intel Corporation.
更多查看译文
关键词
chromatin accessibility,atac-seq
AI 理解论文
溯源树
样例
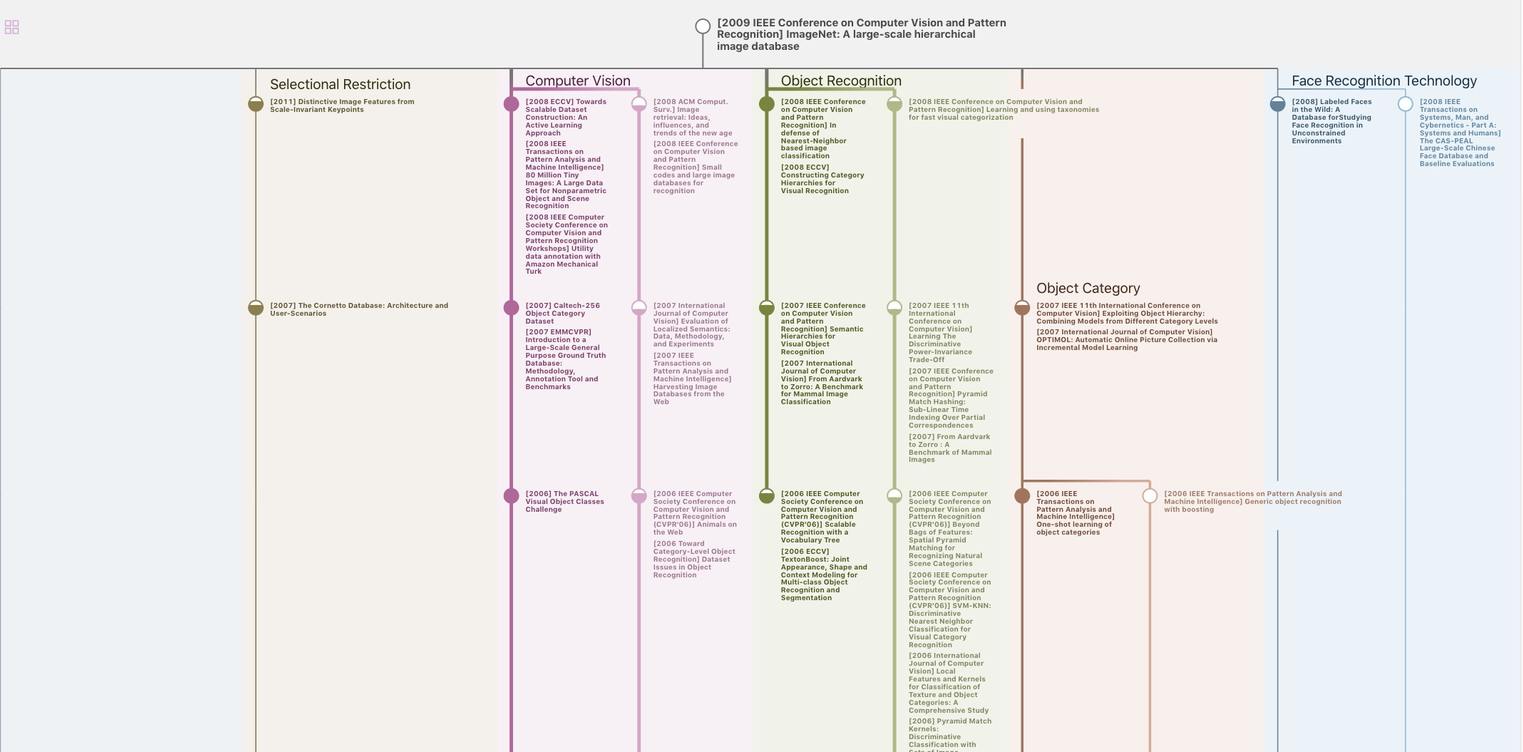
生成溯源树,研究论文发展脉络
Chat Paper
正在生成论文摘要