Batch effects removal for microbiome data via conditional quantile regression
biorxiv(2022)
摘要
Batch effects in microbiome data arise from differential processing of specimens and can lead to spurious findings and obscure true signals. Strategies designed for genomic data to mitigate batch effects usually fail to address the zero-inflated and over-dispersed microbiome data. Most strategies tailored for microbiome data are restricted to association testing or specialized study designs, failing to allow other analytic goals or general designs. Here, we develop the Conditional Quantile Regression (ConQuR) approach to remove microbiome batch effects using a two-part quantile regression model. ConQuR is a comprehensive method that accommodates the complex distributions of microbial read counts by non-parametric modeling, and it generates batch-removed zero-inflated read counts that can be used in and benefit usual subsequent analyses. We apply ConQuR to simulated and real microbiome datasets and demonstrate its advantages in removing batch effects while preserving the signals of interest.
更多查看译文
关键词
microbiome data,conditional quantile regression,batch effects removal
AI 理解论文
溯源树
样例
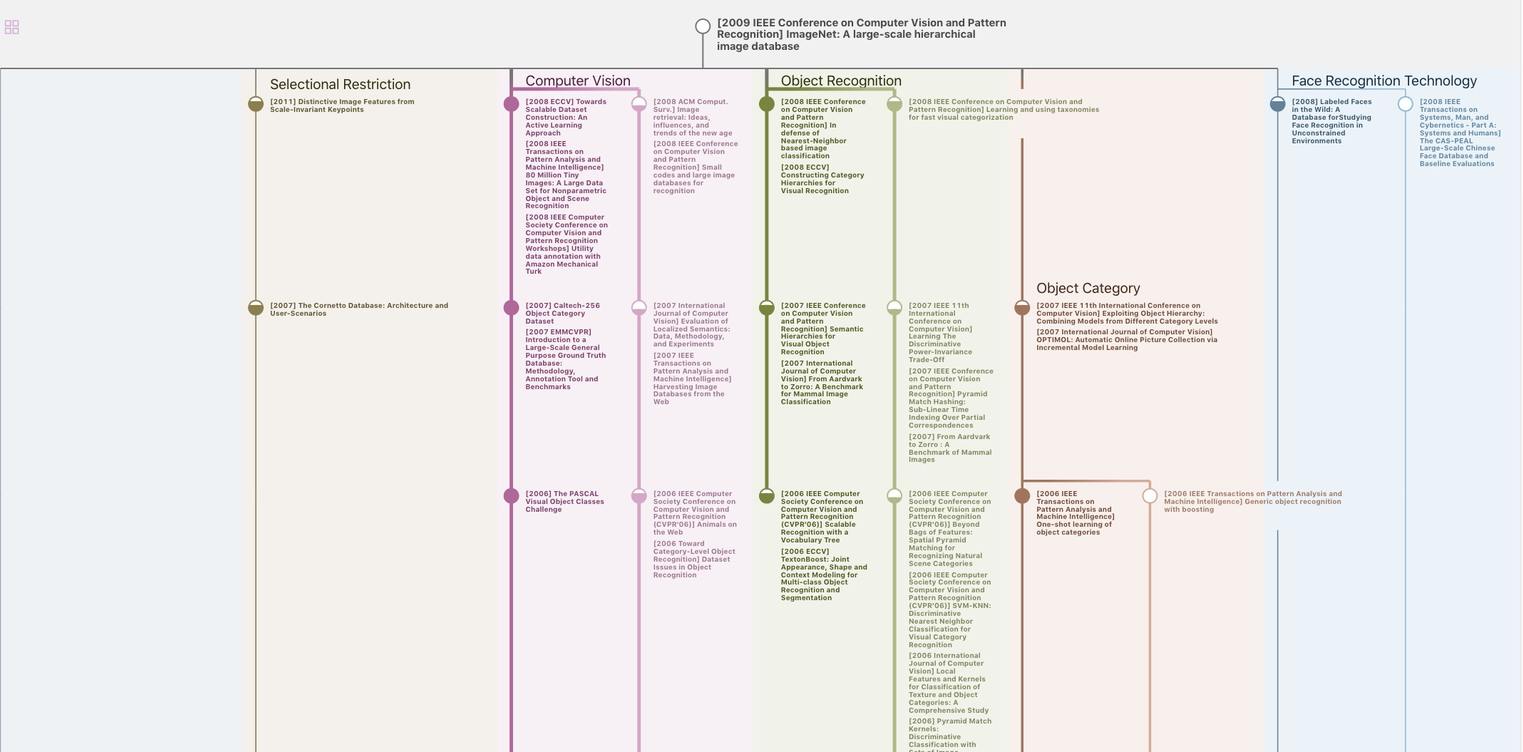
生成溯源树,研究论文发展脉络
Chat Paper
正在生成论文摘要