DrSim: Similarity Learning for Transcriptional Phenotypic Drug Discovery
Genomics, Proteomics & Bioinformatics(2022)
摘要
Transcriptional phenotypic drug discovery has achieved great success, and various compound perturbation-based data resources, such as connectivity map (CMap) and library of integrated network-based cellular signatures (LINCS), have been presented. Computational strategies fully mining these resources for phenotypic drug discovery have been proposed. Among them, the fundamental issue is to define the proper similarity between transcriptional profiles. Traditionally, such similarity has been defined in an unsupervised way. However, due to the high dimensionality and the existence of high noise in high-throughput data, similarity defined in the traditional way lacks robustness and has limited performance. To this end, we present DrSim, which is a learning-based framework that automatically infers similarity rather than defining it. We evaluated DrSim on publicly available in vitro and in vivo datasets in drug annotation and repositioning. The results indicated that DrSim outperforms the existing methods. In conclusion, by learning transcriptional similarity, DrSim facilitates the broad utility of high-throughput transcriptional perturbation data for phenotypic drug discovery. The source code and manual of DrSim are available at https://github.com/bm2-lab/DrSim/.
更多查看译文
关键词
Metric learning,Transcriptional profile similarity,Drug annotation,Drug repositioning,LINCS
AI 理解论文
溯源树
样例
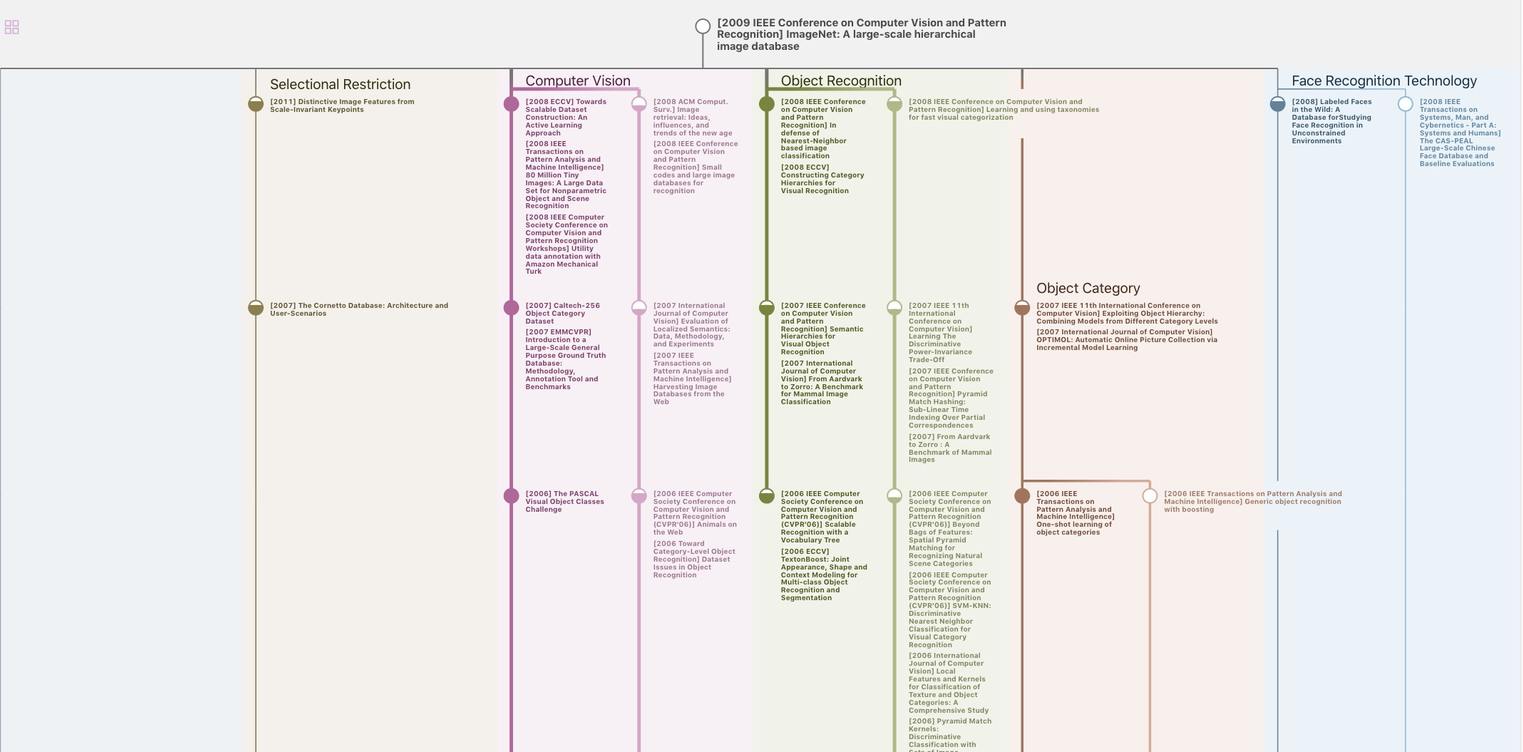
生成溯源树,研究论文发展脉络
Chat Paper
正在生成论文摘要