Effective expression analysis using gene interaction matrices and convolutional neural networks
biorxiv(2021)
摘要
Artificial intelligence recently experienced a renaissance with the advancement of convolutional neural networks (CNNs). CNNs require spatially meaningful matrices ( e.g ., image data) with recurring patterns, limiting its applicability to high-throughput omics data. We present GIM, a simple, CNN-ready framework for omics data to detect both individual and network-level entities of biological importance. Using gene expression data, we show that GIM-CNNs can outperform comparable neural networks in performance and their design facilitates network-level interpretability. GIM-CNNs provide a means to discover novel disease-relevant factors beyond individual genes and their expression, factors that are likely missed by standard differential gene expression approaches.
### Competing Interest Statement
The authors have declared no competing interest.
更多查看译文
关键词
effective expression analysis,gene interaction matrices,neural networks
AI 理解论文
溯源树
样例
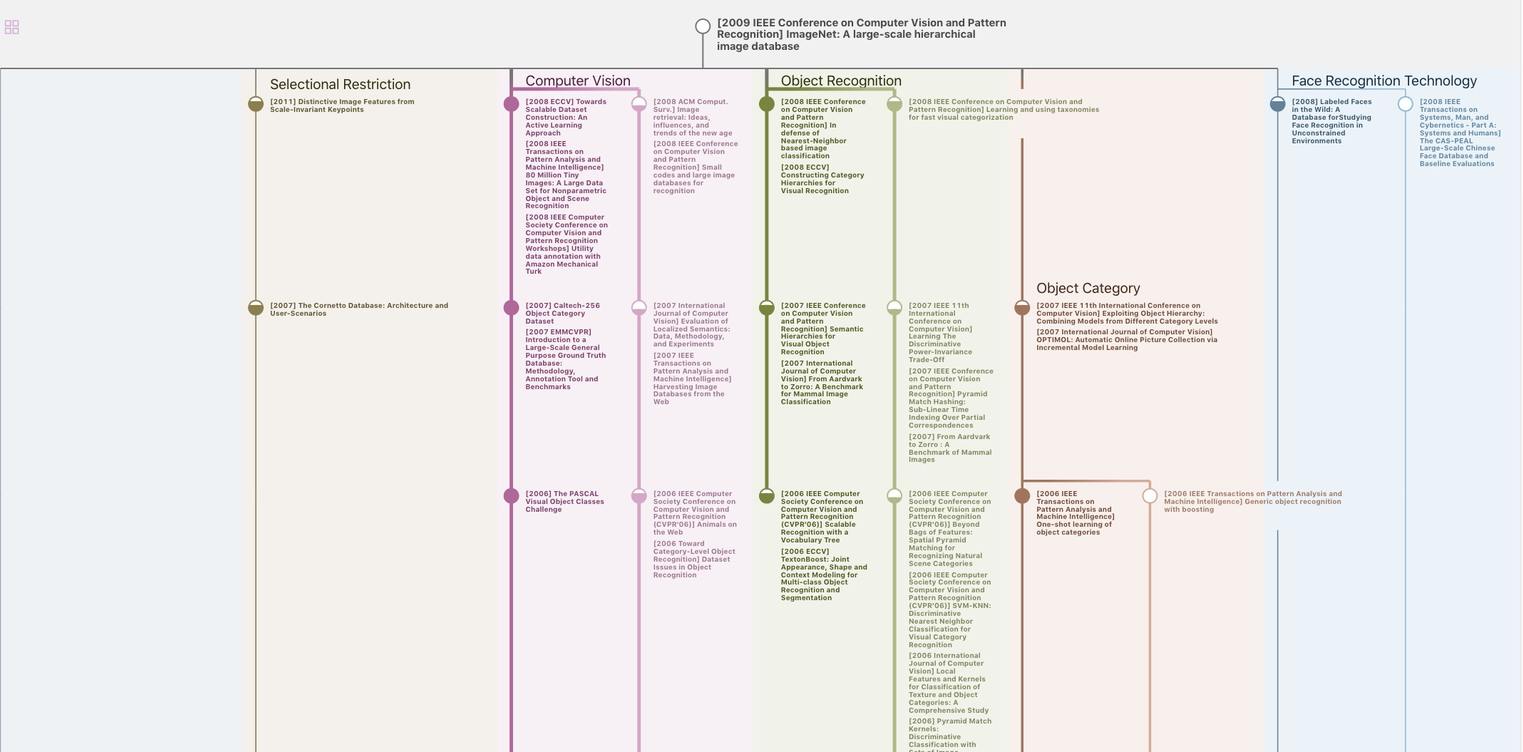
生成溯源树,研究论文发展脉络
Chat Paper
正在生成论文摘要