dsMTL - a computational framework for privacy-preserving, distributed multi-task machine learning
Bioinform.(2021)
摘要
Multitask learning allows the simultaneous learning of multiple ‘communicating’ algorithms. It is increasingly adopted for biomedical applications, such as the modeling of disease progression. As data protection regulations limit data sharing for such analyses, an implementation of multitask learning on geographically distributed data sources would be highly desirable. Here, we describe the development of dsMTL, a computational framework for privacy-preserving, distributed multi-task machine learning that includes three supervised and one unsupervised algorithms. dsMTL is implemented as a library for the R programming language and builds on the DataSHIELD platform that supports the federated analysis of sensitive individual-level data. We provide a comparative evaluation of dsMTL for the identification of biological signatures in distributed datasets using two case studies, and evaluate the computational performance of the supervised and unsupervised algorithms. dsMTL provides an easy- to-use framework for privacy-preserving, federated analysis of geographically distributed datasets, and has several application areas, including comorbidity modeling and translational research focused on the simultaneous prediction of different outcomes across datasets. dsMTL is available at (server-side package) and (client-side package).
### Competing Interest Statement
AML has received consultant fees from: Boehringer Ingelheim, Elsevier, Brainsway, Lundbeck Int. Neuroscience Foundation, Lundbeck A/S, The Wolfson Foundation, Bloomfield Holding Ltd, Shanghai Research Center for Brain Science, Thieme Verlag, Sage Therapeutics, v Behring Roentgen Stiftung, Fondation FondaMental, Janssen-Cilag GmbH, MedinCell, Brain Mind Institute, Agence Nationale de la Recherche, CISSN (Catania Internat. Summer School of Neuroscience), Daimler und Benz Stiftung, American Association for the Advancement of Science, Servier International. Additionally he has received speaker fees from: Italian Society of Biological Psychiatry, Merz-Stiftung, Forum Werkstatt Karlsruhe, Lundbeck SAS France, BAG Psychiatrie Oberbayern, Klinik fuer Psychiatrie und Psychotherapie Ingolstadt, med Update GmbH, Society of Biological Psychiatry, Siemens Healthineers, Biotest AG. All other authors have no potential conflicts of interest.
更多查看译文
关键词
machine learning,privacy-preserving,multi-task
AI 理解论文
溯源树
样例
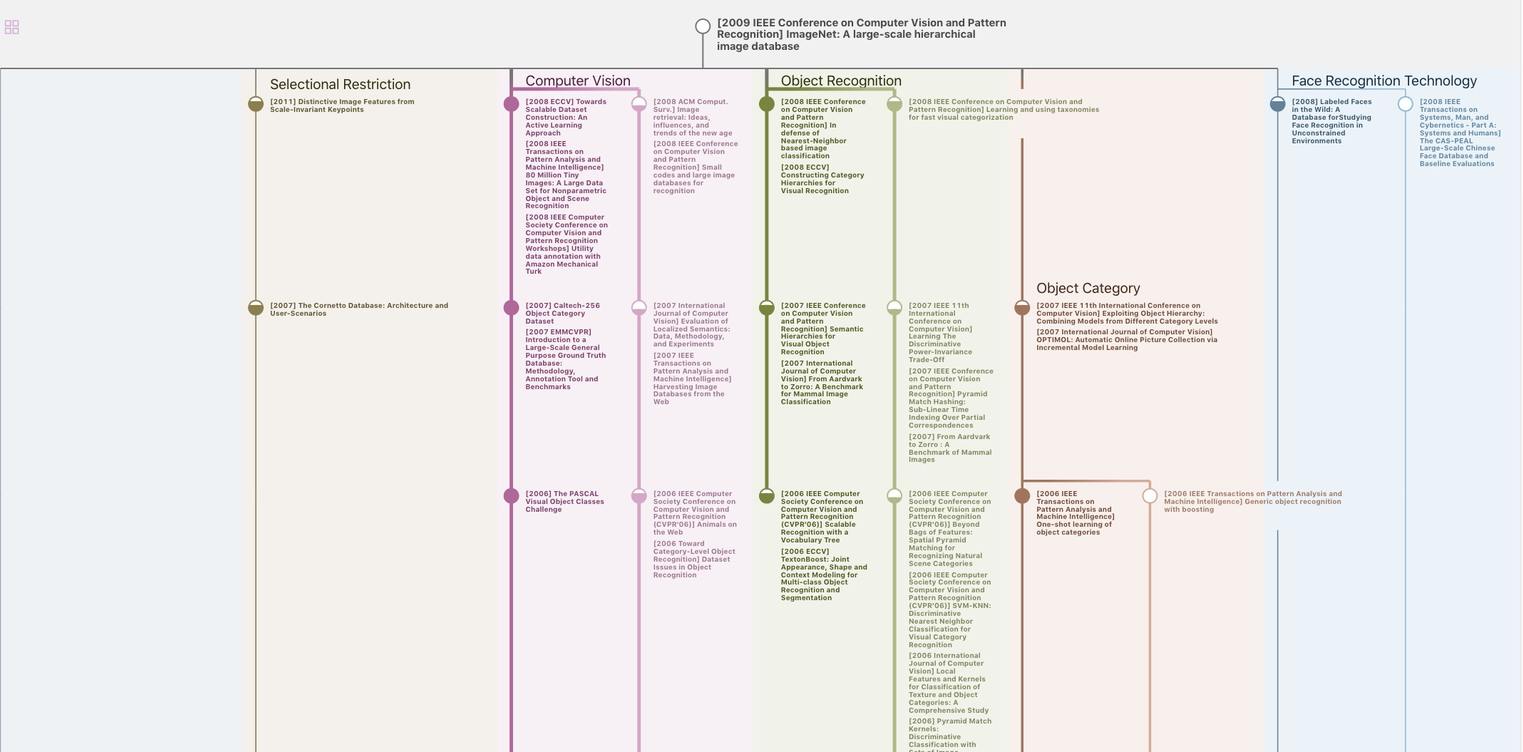
生成溯源树,研究论文发展脉络
Chat Paper
正在生成论文摘要