Scoring protein sequence alignments using deep learning
BIOINFORMATICS(2022)
摘要
Motivation: A high-quality sequence alignment (SA) is the most important input feature for accurate protein structure prediction. For a protein sequence, there are many methods to generate a SA. However, when given a choice of more than one SA for a protein sequence, there are no methods to predict which SA may lead to more accurate models without actually building the models. In this work, we describe a method to predict the quality of a protein's SA. Results: We created our own dataset by generating a variety of SAs for a set of 1351 representative proteins and investigated various deep learning architectures to predict the local distance difference test (IDDT) scores of distance maps predicted with SAs as the input. These IDDT scores serve as indicators of the quality of the SAs. Using two independent test datasets consisting of CASP13 and CASP14 targets, we show that our method is effective for scoring and ranking SAs when a pool of SAs is available for a protein sequence. With an example, we further discuss that SA selection using our method can lead to improved structure prediction.
更多查看译文
关键词
deep learning,protein,sequence
AI 理解论文
溯源树
样例
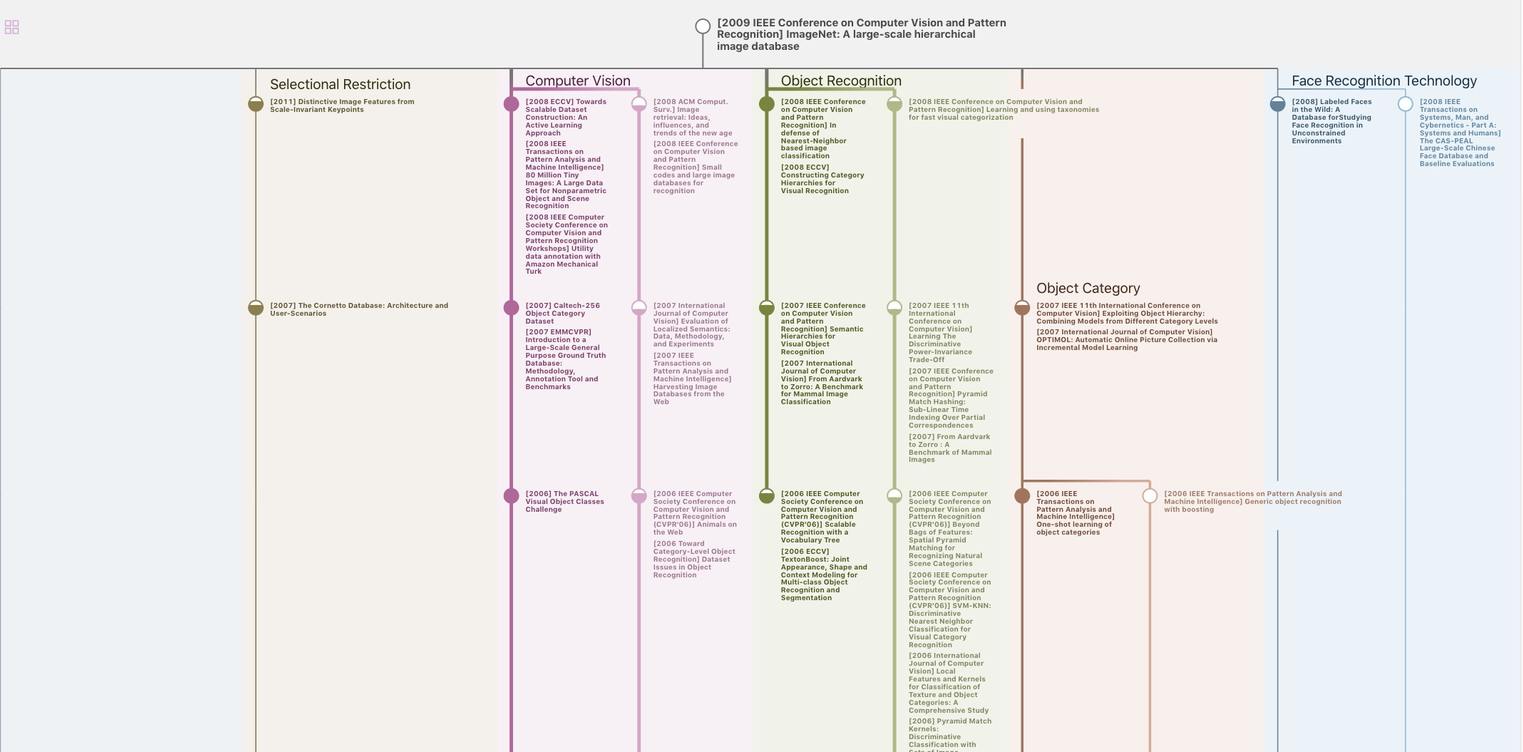
生成溯源树,研究论文发展脉络
Chat Paper
正在生成论文摘要