Deep learning-based segmentation and quantification of podocyte foot process morphology
bioRxiv (Cold Spring Harbor Laboratory)(2021)
摘要
The kidneys constantly filter enormous amounts of fluid, with almost complete retention of albumin and other macromolecules in the plasma. Diseases of podocytes at the kidney filtration barrier reduce the intrinsic permeability of the capillary wall resulting in albuminuria. However, direct quantitative assessment of the underlying morphological changes has previously not been possible. Here we developed a deep learning-based approach for segmentation of foot processes in images acquired with optical microscopy. Our method – Automatic Morphological Analysis of Podocytes (AMAP) – accurately segments foot processes and robustly quantifies their morphology. It also robustly determined morphometric parameters, at a Pearson correlation of r > 0.71 with a previously published semi-automated approach, across a large set of mouse tissue samples. The artificial intelligence algorithm wasWe applied the analysis to a set of human kidney disease conditions allowing comprehensive quantification of various underlying morphometric parameters. These results confirmed that when podocytes are injured, they take on a more simplified architecture and the slit diaphragm length is significantly shortened, resulting in a reduction in the filtration slit area and a loss of the buttress force of podocytes which increases the permeability of the glomerular basement membrane to albumin.
### Competing Interest Statement
The authors have declared no competing interest.
更多查看译文
AI 理解论文
溯源树
样例
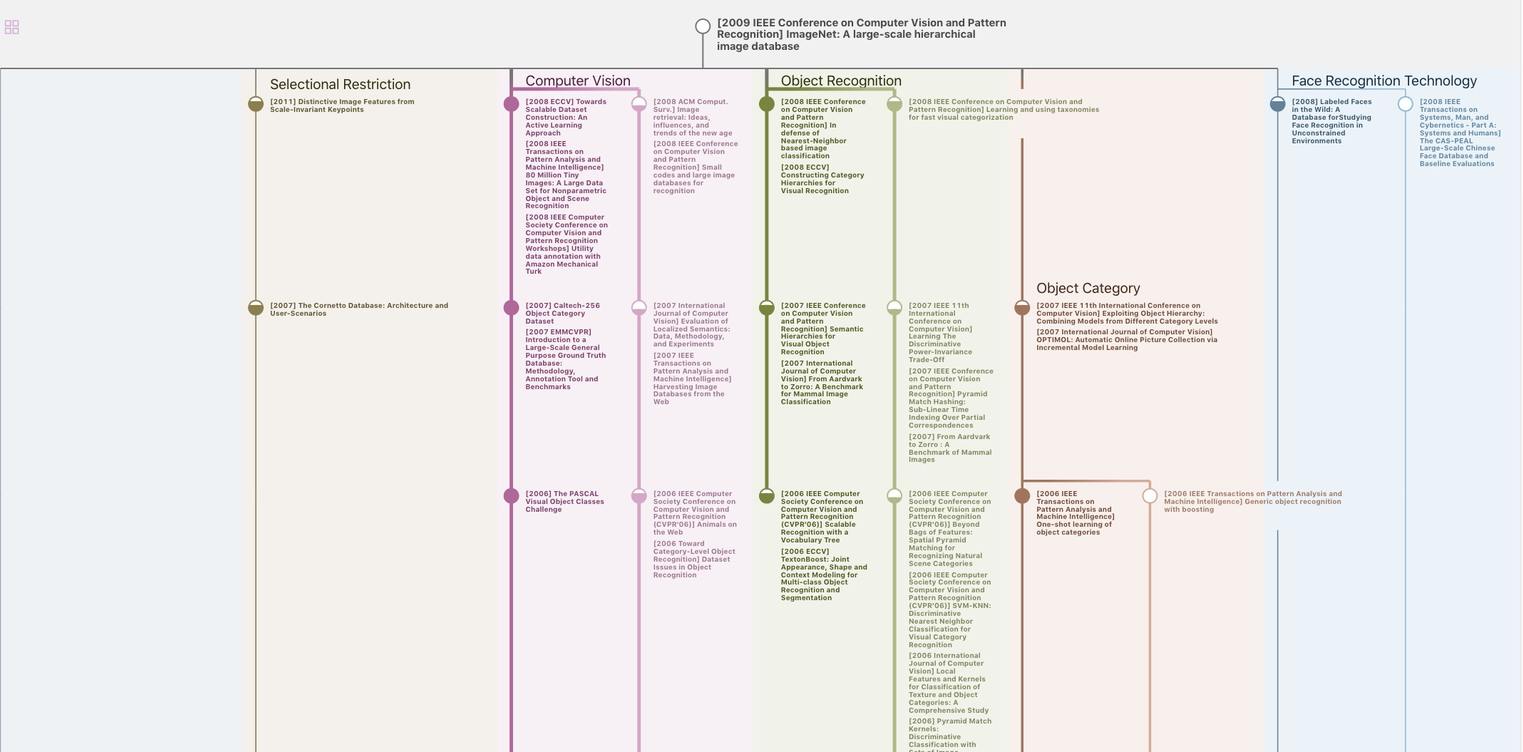
生成溯源树,研究论文发展脉络
Chat Paper
正在生成论文摘要