Linked machine learning classifiers improve species classification of fungi when using error-prone long-reads on extended metabarcodes
biorxiv(2021)
摘要
The increased usage of long-read sequencing for metabarcoding has not been matched with public databases suited for error-prone long-reads. We address this gap and present a proof-of-concept study for classifying fungal species using linked machine learning classifiers. We demonstrate its capability for accurate classification using labelled and unlabelled fungal sequencing datasets. We show the advantage of our approach for closely related species over current alignment and k-mer methods and suggest a confidence threshold of 0.85 to maximise accurate target species identification from complex samples of unknown composition. We suggest future use of this approach in medicine, agriculture, and biosecurity.
### Competing Interest Statement
The authors have declared no competing interest.
更多查看译文
关键词
species classification,classifiers,fungi,machine learning,error-prone,long-reads
AI 理解论文
溯源树
样例
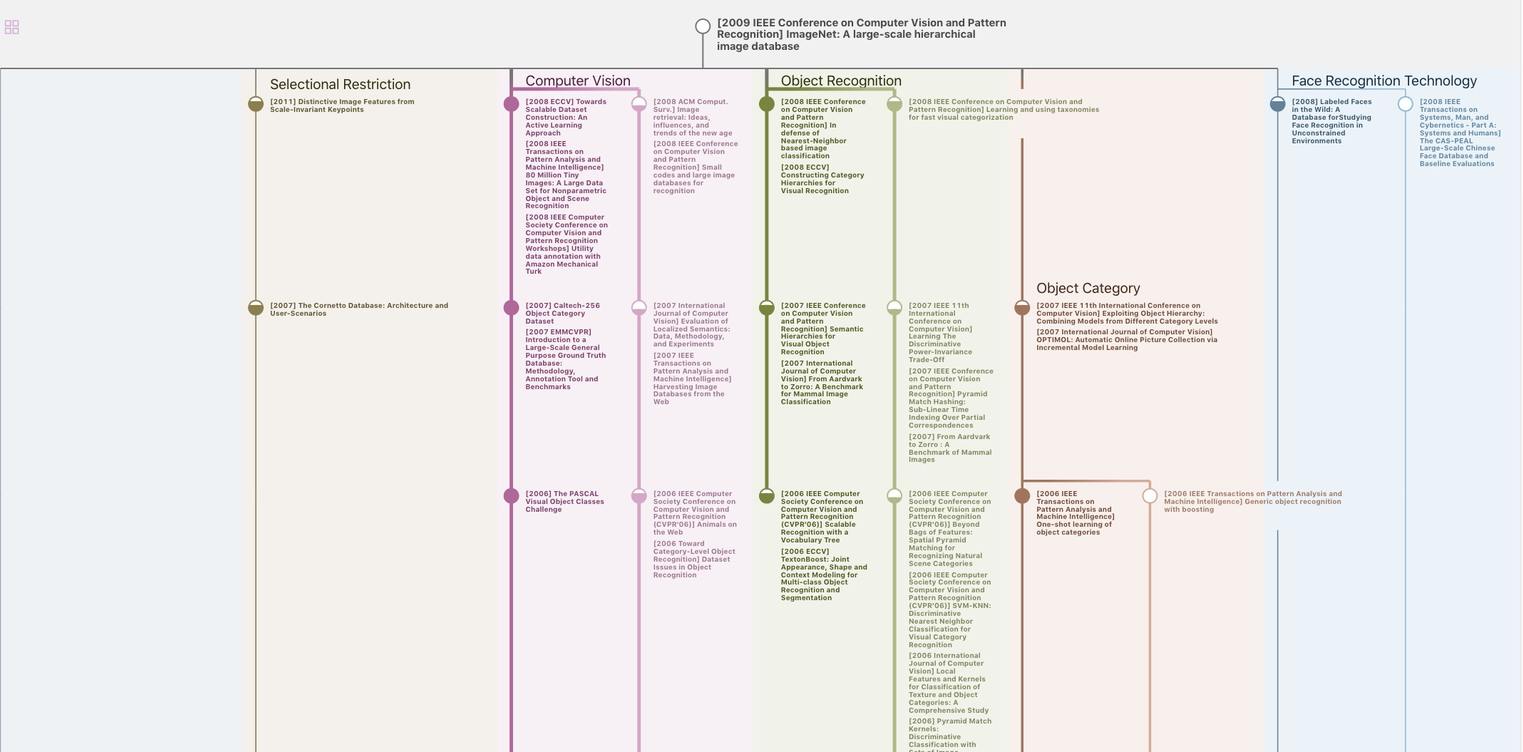
生成溯源树,研究论文发展脉络
Chat Paper
正在生成论文摘要