ME-VAE: Multi-Encoder Variational AutoEncoder for Controlling Multiple Transformational Features in Single Cell Image Analysis
biorxiv(2021)
摘要
Image-based cell phenotyping relies on quantitative measurements as encoded representations of cells; however, defining suitable representations that capture complex imaging features is challenged by the lack of robust methods to segment cells, identify subcellular compartments, and extract relevant features. Variational autoencoder (VAE) approaches produce encouraging results by mapping an image to a representative descriptor, and outperform classical hand-crafted features for morphology, intensity, and texture at differentiating data. Although VAEs show promising results for capturing morphological and organizational features in tissue, single cell image analyses based on VAEs often fail to identify biologically informative features due to uninformative technical variation. Herein, we propose a multi-encoder VAE (ME-VAE) in single cell image analysis using transformed images as a self-supervised signal to extract transform-invariant biologically meaningful features, including emergent features not obvious from prior knowledge. We show that the proposed architecture improves analysis by making distinct cell populations more separable compared to traditional VAEs and intensity measurements by enhancing phenotypic differences between cells and by improving correlations to other analytic modalities.
### Competing Interest Statement
The authors have declared no competing interest.
更多查看译文
关键词
single cell image analysis,multiple transformational features,me-vae,multi-encoder
AI 理解论文
溯源树
样例
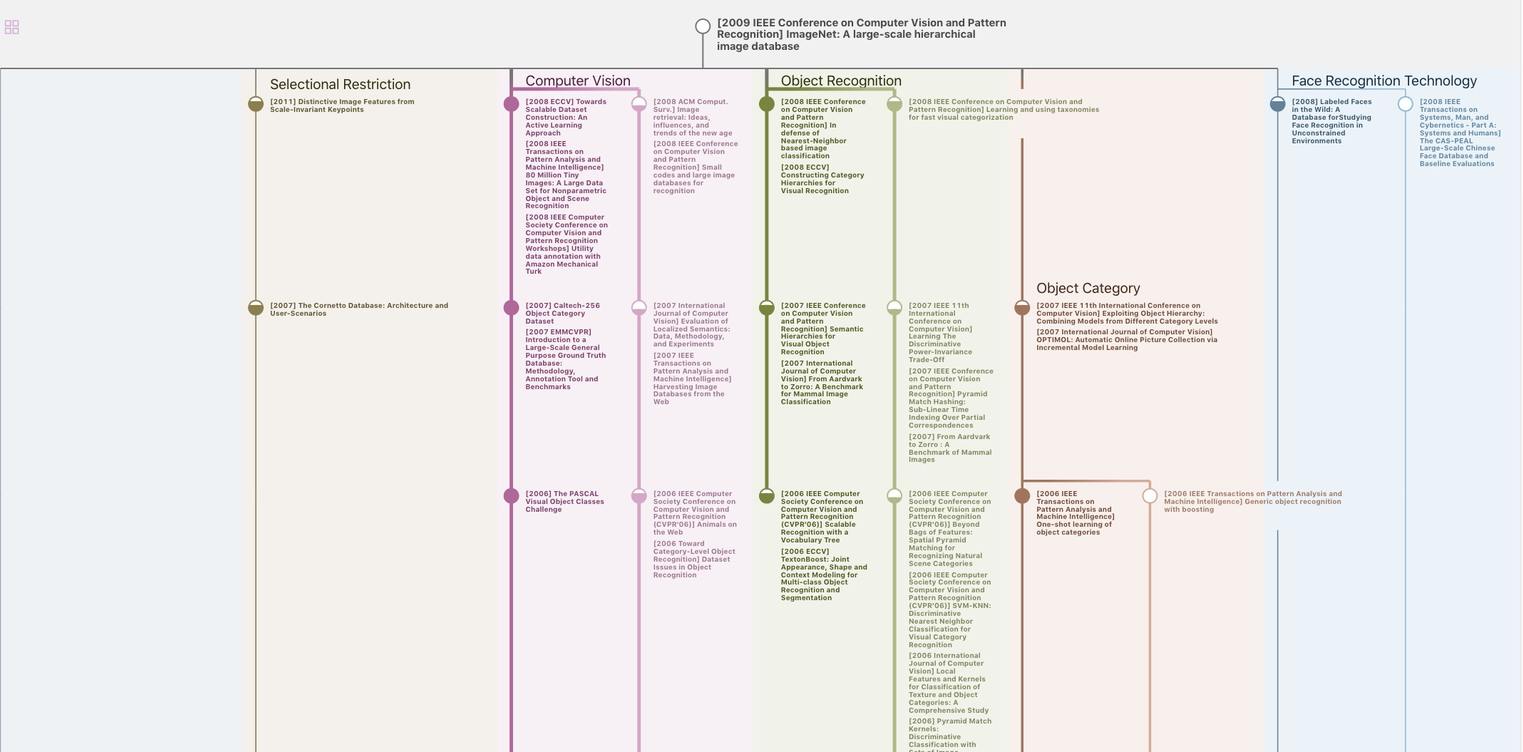
生成溯源树,研究论文发展脉络
Chat Paper
正在生成论文摘要