CliP: subclonal architecture reconstruction of cancer cells in DNA sequencing data using a penalized likelihood model
biorxiv(2021)
摘要
Subpopulations of tumor cells characterized by mutation profiles may confer differential fitness and consequently influence prognosis of cancers. Understanding subclonal architecture has the potential to provide biological insight in tumor evolution and advance precision cancer treatment. Recent methods comprehensively integrate single nucleotide variants (SNVs) and copy number aberrations (CNAs) to reconstruct subclonal architecture using whole-genome or whole-exome sequencing (WGS, WES) data from bulk tumor samples. However, the commonly used Bayesian methods require a large amount of computational resources, a prior knowledge of the number of subclones, and extensive post-processing. Regularized likelihood modeling approach, never explored for subclonal reconstruction, can inherently address these drawbacks. We therefore propose a model-based method, Clonal structure identification through pair-wise Penalization, or CliP, for clustering subclonal mutations without prior knowledge or post-processing. The CliP model is applicable to genomic regions with or without CNAs. CliP demonstrates high accuracy in subclonal reconstruction through extensive simulation studies. Utilizing the well-established regularized likelihood framework, CliP takes only 16 hours to process WGS data from 2,778 tumor samples in the ICGC-PCAWG study, and 38 hours to process WES data from 9,564 tumor samples in the TCGA study. In summary, a penalized likelihood framework for subclonal reconstruction will help address intrinsic drawbacks of existing methods and expand the scope of computational analysis for cancer evolution in large cancer genomic studies. The associated software tool is freely available at: .
### Competing Interest Statement
The authors have declared no competing interest.
更多查看译文
AI 理解论文
溯源树
样例
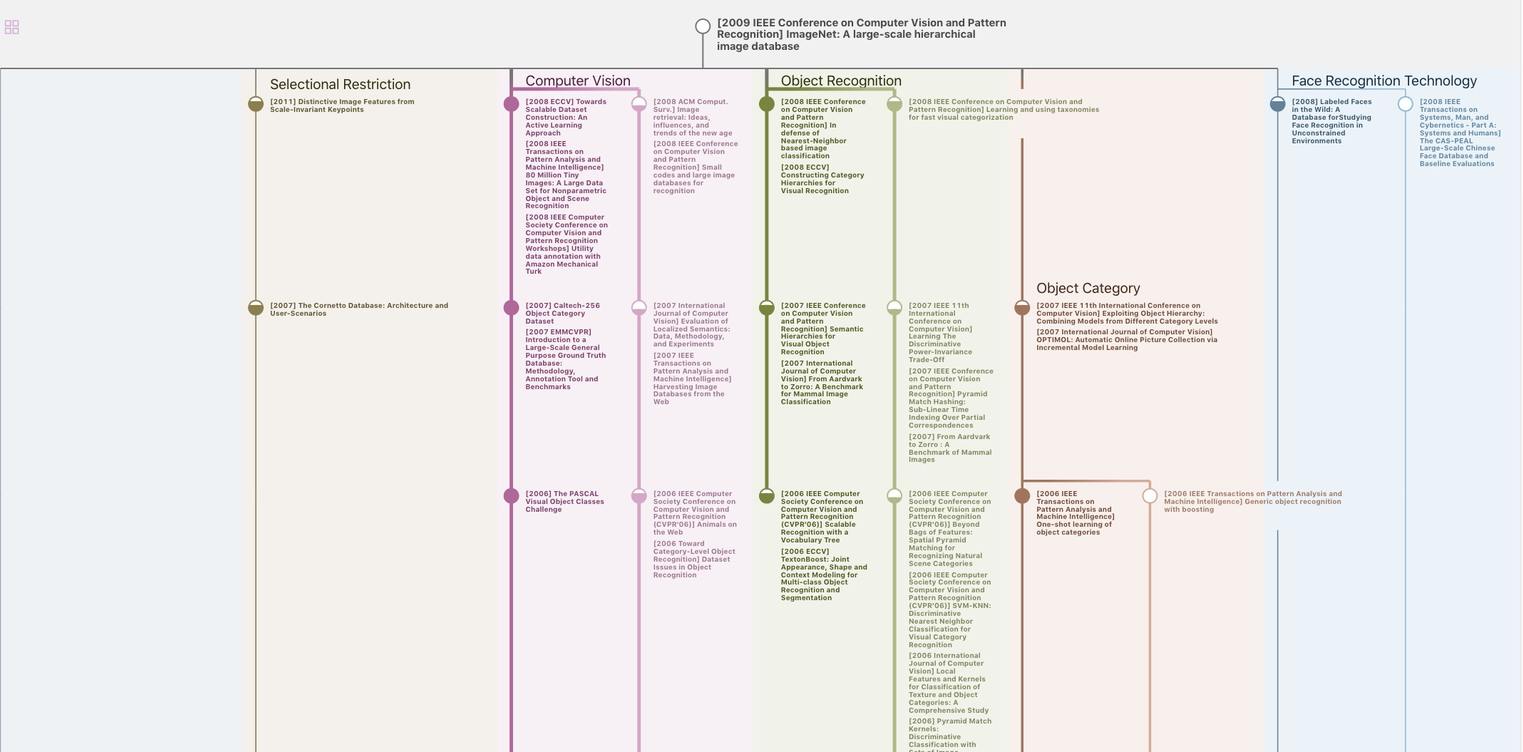
生成溯源树,研究论文发展脉络
Chat Paper
正在生成论文摘要