A General LSTM-based Deep Learning Method for Estimating Neuronal Models and Inferring Neural Circuitry
biorxiv(2021)
摘要
Computational neural models are essential tools for neuroscientists to study the functional roles of single neurons or neural circuits. With the recent advances in experimental techniques, there is a growing demand to build up neural models at single neuron or large-scale circuit levels. A long-standing challenge to build up such models lies in tuning the free parameters of the models to closely reproduce experimental recordings. There are many advanced machine-learning-based methods developed recently for parameter tuning, but many of them are task-specific or requires onerous manual interference. There lacks a general and fully-automated method since now. Here, we present a Long Short-Term Memory (LSTM)-based deep learning method, General Neural Estimator (GNE), to fully automate the parameter tuning procedure, which can be directly applied to both single neuronal models and large-scale neural circuits. We made comprehensive comparisons with many advanced methods, and GNE showed outstanding performance on both synthesized data and experimental data. Finally, we proposed a roadmap centered on GNE to help guide neuroscientists to computationally reconstruct single neurons and neural circuits, which might inspire future brain reconstruction techniques and corresponding experimental design. The code of our work will be publicly available upon acceptance of this paper.
### Competing Interest Statement
The authors have declared no competing interest.
更多查看译文
关键词
neuronal models,deep learning,deep learning method,lstm-based
AI 理解论文
溯源树
样例
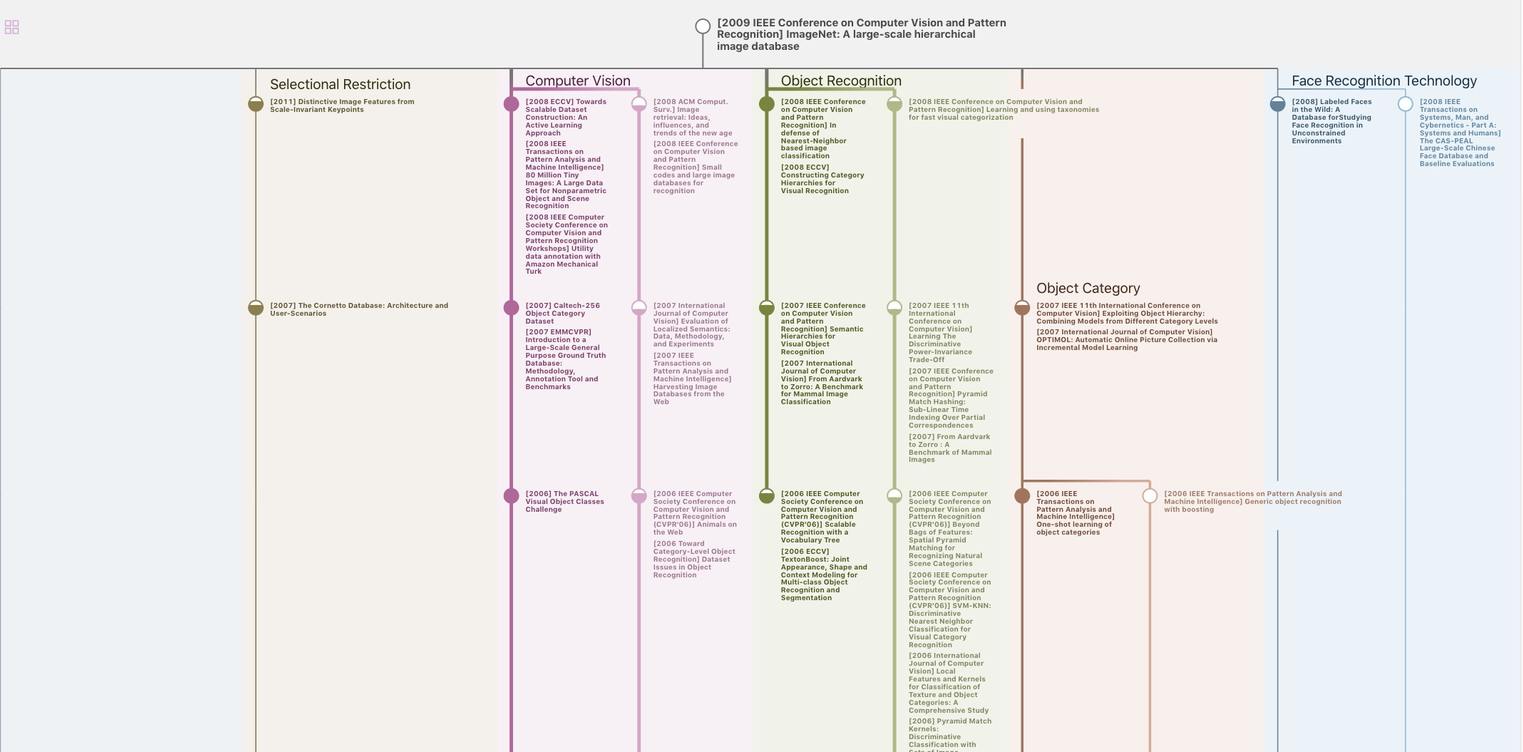
生成溯源树,研究论文发展脉络
Chat Paper
正在生成论文摘要