The Organelle in the Ointment: cryptic mitochondria account for many unknown sequences in cross-species microbiome comparisons
biorxiv(2023)
摘要
The genomes of mitochondria and chloroplasts contain ribosomal RNA (rRNA) genes, reflecting their evolutionary ancestry as free-living bacteria prior to endosymbiosis. In microbiome studies of animals, plants, or other eukaryotic hosts, these organellar rRNAs are often amplified. If identified, they can be discarded, merely reducing sequencing depth. However, incorrectly annotated mitochondrial reads may compromise statistical analysis by distorting relative abundances of free-living microbes. We quantified this by reanalyzing 7,459 samples from seven 16S rRNA sequencing studies, including the microbiomes of 927 unique animal genera. We find that under-annotation of cryptic mitochondrial reads affects multiple of these large-scale cross-species microbiome comparisons, and can be severe in some samples. It also varies between host species, potentially biasing cross-species microbiome comparisons. We propose a straightforward solution: by supplementing existing taxonomies with diverse mitochondrial rRNA sequences, we resolve up to 97% of unique unclassified sequences in some entire studies as mitochondrial (14% averaged across all studies), without increasing false positive annotations in mitochondria-free mock communities. Overall, improved annotation decreases the proportion of unknown sequences by ≥10-fold in 2,262 of 7,459 samples (30%), including representatives from 5 of 7 studies examined. While standard DADA2 analyses are severely affected, the default positive filter in Deblur run through QIIME2 discards many divergent mitochondrial sequences, preventing bias in analysis, but also making analysis of these sequences more difficult. We recommend leveraging mitochondrial sequence diversity to better identify, remove and analyze mitochondrial rRNA gene sequences in microbiome studies.
### Competing Interest Statement
The authors have declared no competing interest.
更多查看译文
AI 理解论文
溯源树
样例
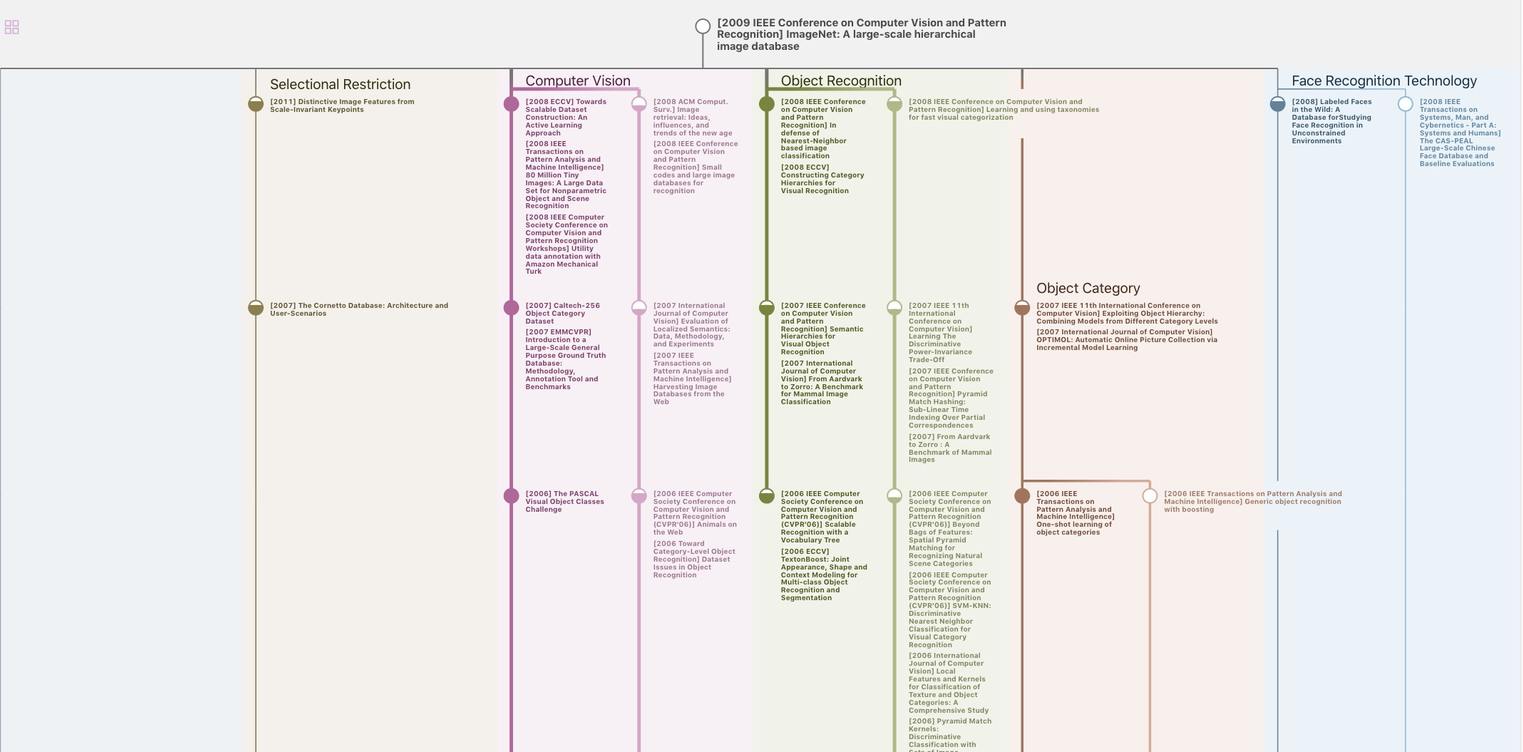
生成溯源树,研究论文发展脉络
Chat Paper
正在生成论文摘要