Optimal Reinforcement Learning with Asymmetric Updating in Volatile Environments: a Simulation Study
biorxiv(2021)
摘要
The ability to predict the future is essential for decision-making and interaction with the environment to avoid punishment and gain reward. Reinforcement learning algorithms provide a normative way for interactive learning, especially in volatile environments. The optimal strategy for the classic reinforcement learning model is to increase the learning rate as volatility increases. Inspired by optimistic bias in humans, an alternative reinforcement learning model has been developed by adding a punishment learning rate to the classic reinforcement learning model. In this study, we aim to 1) compare the performance of these two models in interaction with different environments, and 2) find optimal parameters for the models. Our simulations indicate that having two different learning rates for rewards and punishments increases performance in a volatile environment. Investigation of the optimal parameters shows that in almost all environments, having a higher reward learning rate compared to the punishment learning rate is beneficial for achieving higher performance which in this case is the accumulation of more rewards. Our results suggest that to achieve high performance, we need a shorter memory window for recent rewards and a longer memory window for punishments. This is consistent with optimistic bias in human behavior.
### Competing Interest Statement
The authors have declared no competing interest.
更多查看译文
关键词
volatile environments,optimal,learning,simulation study
AI 理解论文
溯源树
样例
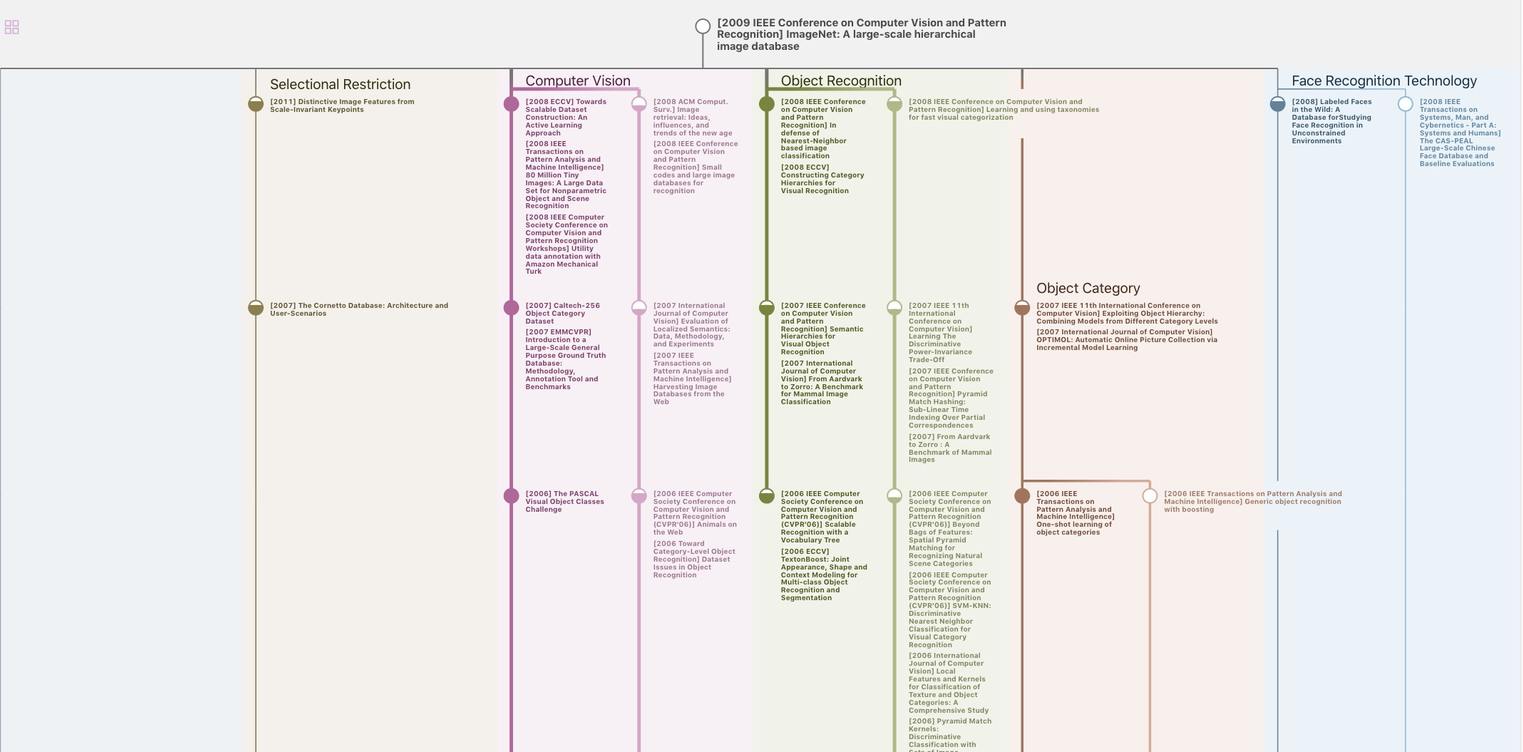
生成溯源树,研究论文发展脉络
Chat Paper
正在生成论文摘要