Sequence-to-Lattice Models for Fast Translation.
EMNLP(2021)
摘要
Non-autoregressive machine translation (NAT) approaches enable fast generation by utilizing parallelizable generative processes. The remaining bottleneck in these models is their decoder layers; unfortunately unlike in autoregressive models (Kasai et al., 2020), removing decoder layers from NAT models significantly degrades accuracy. This work proposes a sequence-to-lattice model that replaces the decoder with a search lattice. Our approach first constructs a candidate lattice using efficient lookup operations, generates lattice scores from a deep encoder, and finally finds the best path using dynamic programming. Experiments on three machine translation datasets show that our method is faster than past non-autoregressive generation approaches, and more accurate than naively reducing the number of decoder layers.
更多查看译文
关键词
fast translation,models,sequence-to-lattice
AI 理解论文
溯源树
样例
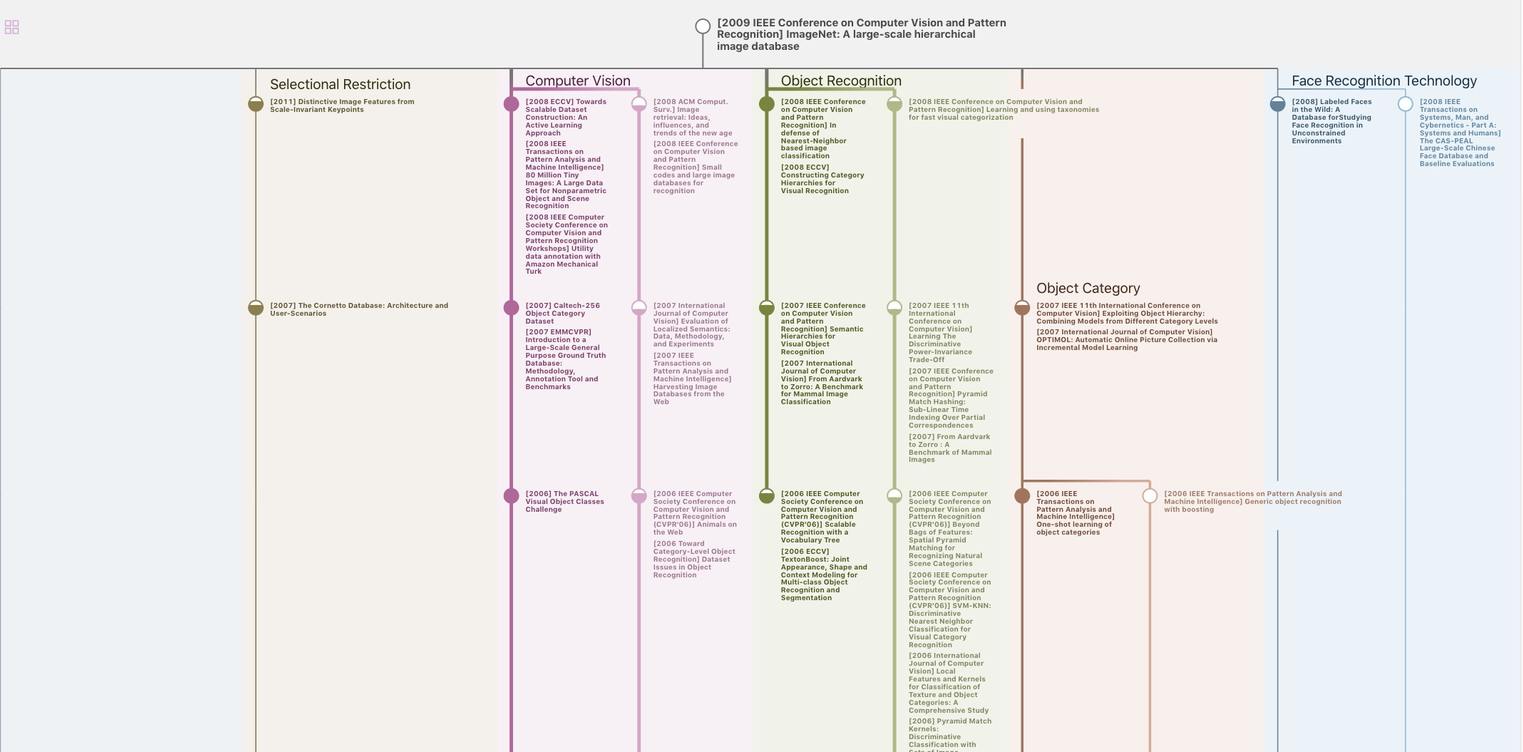
生成溯源树,研究论文发展脉络
Chat Paper
正在生成论文摘要