TARe: Task-Adaptive in-situ ReRAM Computing for Graph Learning
2021 58TH ACM/IEEE DESIGN AUTOMATION CONFERENCE (DAC)(2021)
摘要
ReRAM-based Computing-in-Memory (CiM) architecture has been considered an ideal solution to neural networks, by conducting in-situ matrix multiplications without moving the neural parameters from memory cells. However, we found that keeping the parameters static in ReRAM cells, i.e. weight-static processing, is not the sole choice to implement emerging graph neural networks (GNNs) that operate on the input of ultra large graphs. Therefore, we propose TARe, a Task-Adaptive CiM architecture that supports multiple different in-situ computing modes for Graph Learning. With the proposed novel hybrid in-situ computing architecture, TARe achieves 451.98x speedup on average over the baseline in SOTA GNN workloads.
更多查看译文
关键词
ReRAM, in-situ computing, graph learning, computing-in-memory, accelerator
AI 理解论文
溯源树
样例
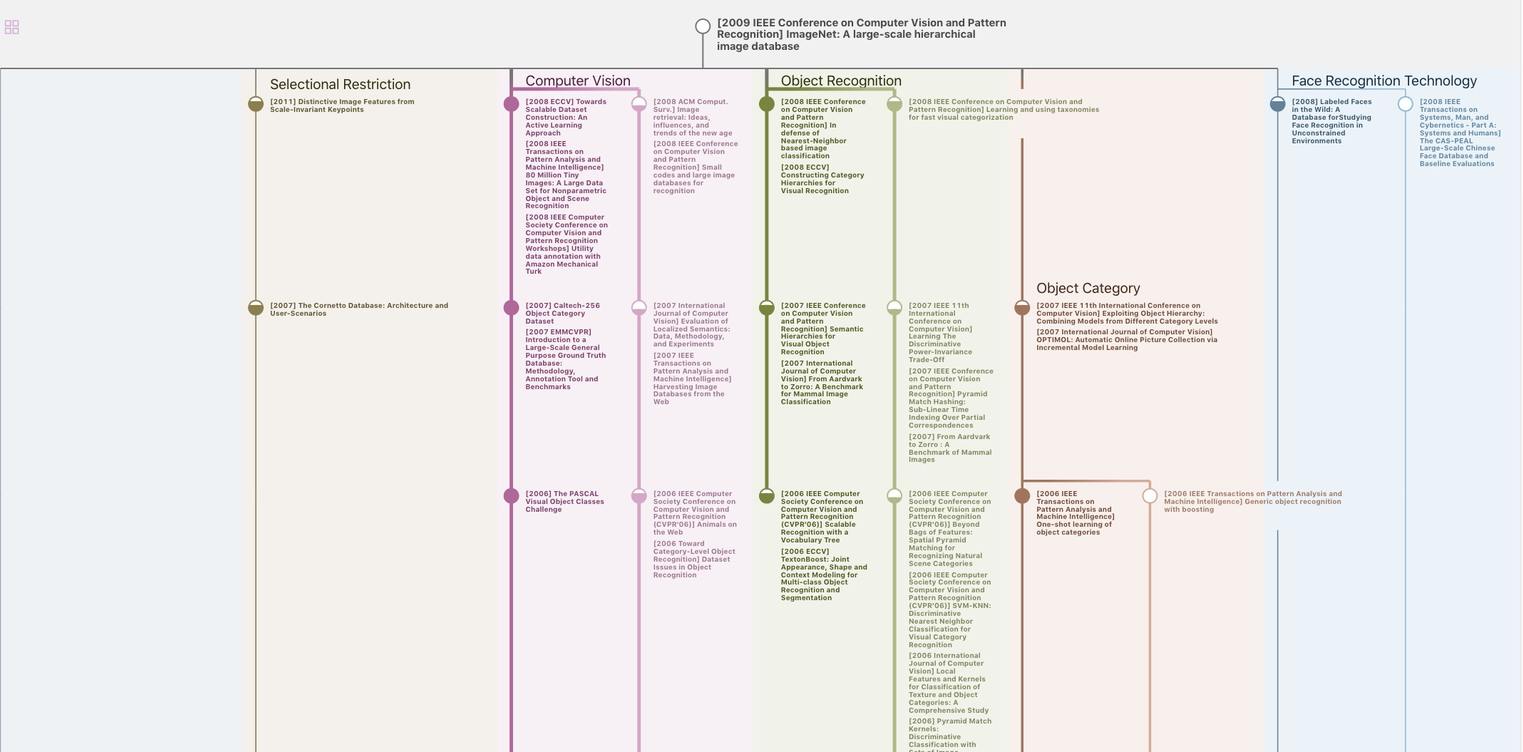
生成溯源树,研究论文发展脉络
Chat Paper
正在生成论文摘要