VLSI Structure-aware Placement for Convolutional Neural Network Accelerator Units
2021 58TH ACM/IEEE DESIGN AUTOMATION CONFERENCE (DAC)(2021)
摘要
AI-dedicated hardware designs are growing dramatically for various AI applications. These designs often contain highly connected circuit structures, reflecting the complicated structure in neural networks, such as convolutional layers and fully-connected layers. As a result, such dense interconnections incur severe congestion problems in physical design that cannot be solved by conventional placement methods. This paper proposes a novel placement framework for CNN accelerator units, which extracts kernels from the circuit and insert kernel-based regions to guide placement and minimize routing congestion. Experimental results show that our framework effectively reduces global routing congestion without wirelength degradation, significantly outperforming leading commercial tools.
更多查看译文
关键词
convolutional neural network accelerator units,AI-dedicated hardware designs,AI applications,highly connected circuit structures,neural networks,convolutional layers,fully-connected layers,dense interconnections,congestion problems,conventional placement methods,CNN accelerator units,global routing congestion,VLSI structure-aware placement,kernel-based regions
AI 理解论文
溯源树
样例
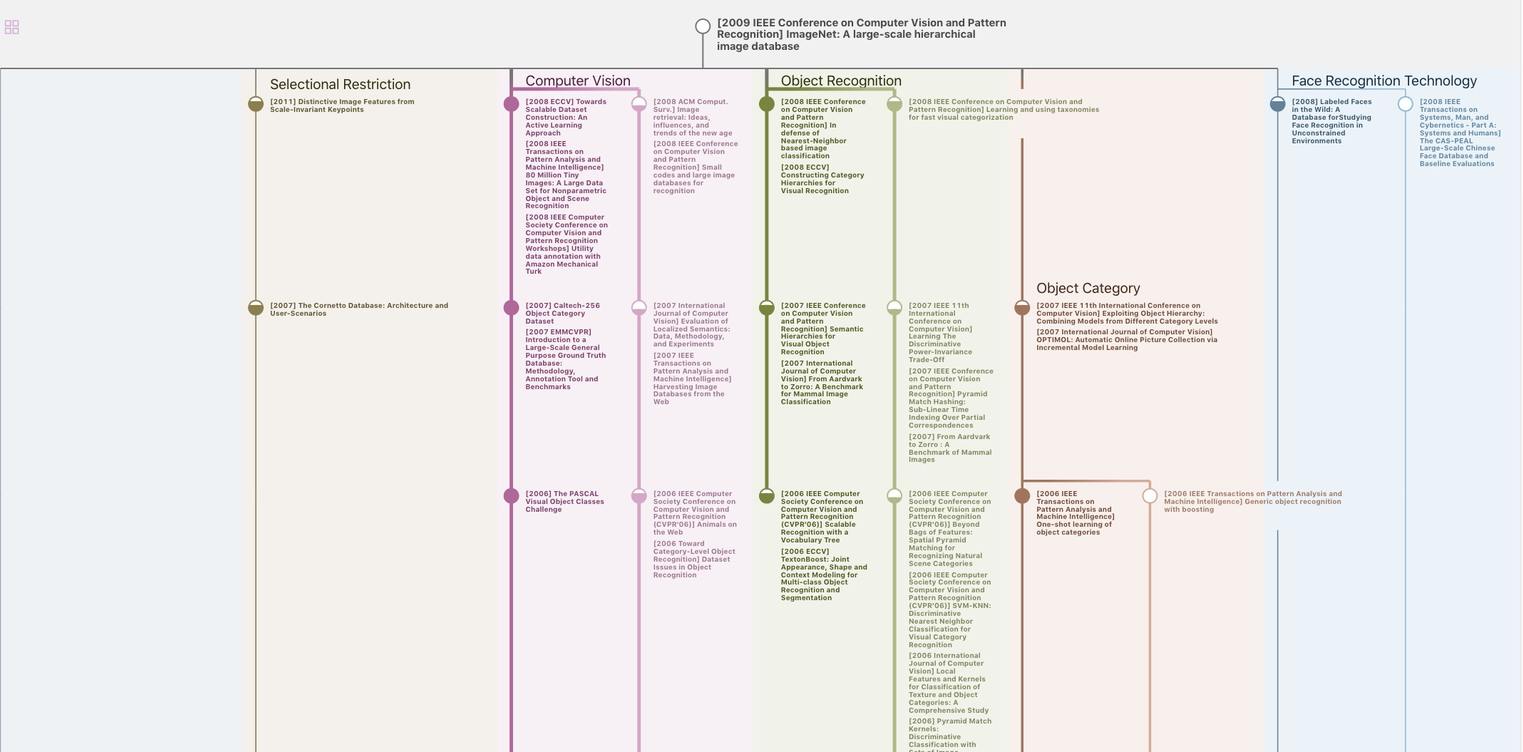
生成溯源树,研究论文发展脉络
Chat Paper
正在生成论文摘要