Quality monitoring for injection moulding process using a semi-supervised learning approach
IECON 2021 - 47TH ANNUAL CONFERENCE OF THE IEEE INDUSTRIAL ELECTRONICS SOCIETY(2021)
摘要
In mass production, supervised machine learning schemes may not be applicable directly in many cases due to insufficient labelled dataset, especially for failed samples. In this paper, we investigate the feasibility of a semi-supervised approach in a quality monitoring system for plastic injection moulding process using real-time manufacturing data. Due to randomly sampled quality inspection, the obtained dataset consists of only a limited amount of labelled data (8%) while a major portion of the dataset is with unlabelled production data (92%). The work starts with real-time data collection and feature extraction for raw data. Then self-learning and 2-views co-training are investigated for 2 MLP base classifiers. Totally six different approaches are studied to build the predictive quality monitoring model. Self-training and co-training approaches start with supervised training as the baseline. Then, two different pseudo labelling schemes are considered to evaluate their effectiveness to the model's performance. Also, the feature selection step is repeated for each iteration to ensure top useful features are included. This training loop continues until all unlabelled data samples are shifted to the labelled dataset or the prediction probability drop below an acceptable threshold. Finally, benchmarking is carried out for all 6 approaches in comparison with the baseline model and the validation results strongly support the use of the Co-trained_Top MLP model for the injection moulding quality monitoring application.
更多查看译文
关键词
Injection moulding, quality monitoring, defect detection, self-training, co-training, MLP
AI 理解论文
溯源树
样例
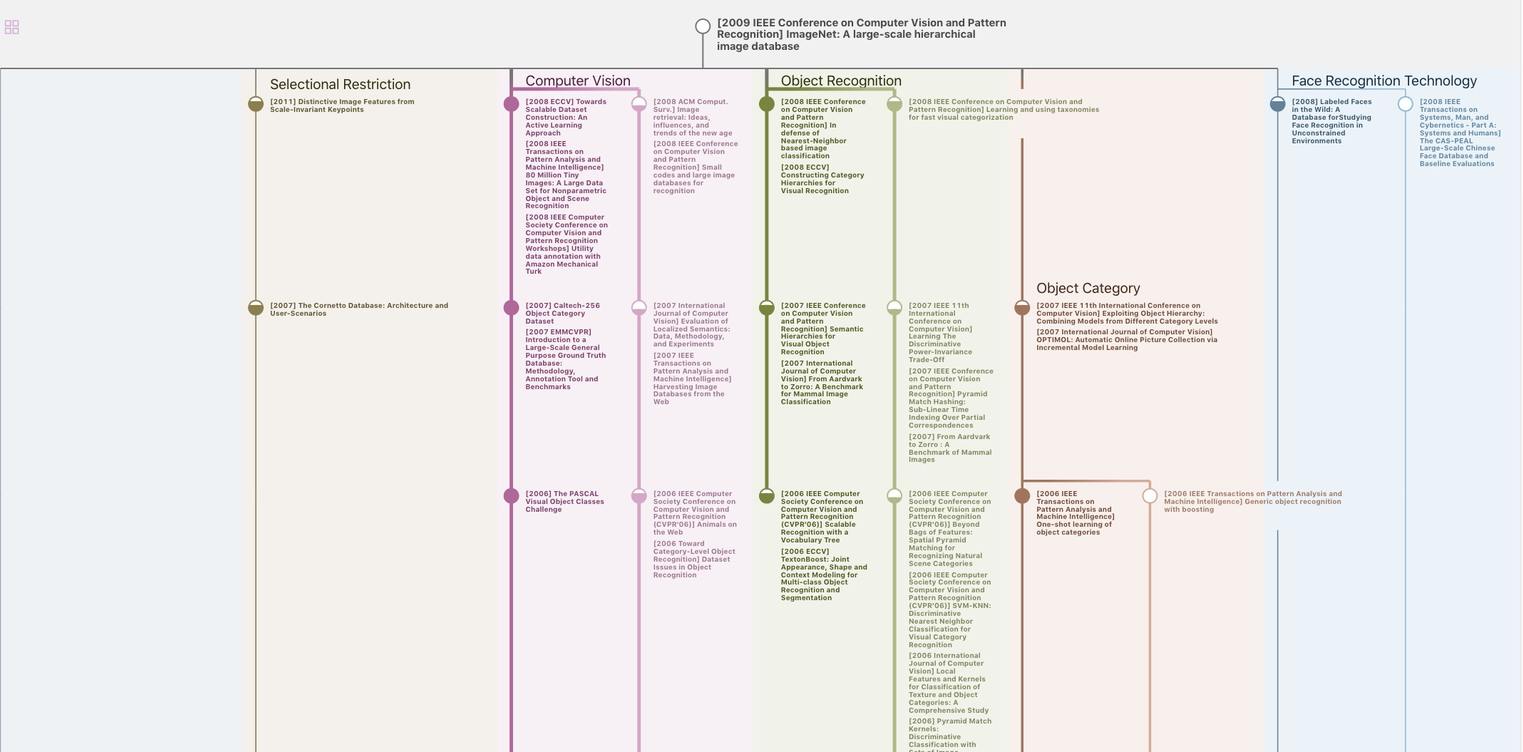
生成溯源树,研究论文发展脉络
Chat Paper
正在生成论文摘要