Automatic Classification of Lithofacies with Highly Imbalanced Dataset Using Multistage SVM Classifier
IECON 2021 - 47TH ANNUAL CONFERENCE OF THE IEEE INDUSTRIAL ELECTRONICS SOCIETY(2021)
摘要
The goal of lithofacies classification is to predict a facies profile at the well site by using the values of rock parameters obtained or computed in well log analysis. Knowledge of lithofacies profiles is required to map the depositional environment of the subsurfaces, from which hydrocarbon zones may be discovered. Statistical techniques give the most feasible categorization of lithological facies across the borehole by maximizing a model, which can predict the chance of a group of rock samples belonging to a specific class. The majority of existing algorithms categorize each sample in the well log separately and classify the different classes. However, mainly, these techniques failed for the complex environments, where the actual data is very imbalanced. In this work, this issue is addressed by using the multistage support vector machine (SVM) classification algorithm with modified regularization parameters for each class. In our case, minority classes are getting worse accuracy than majority classes, which is improved by applying the higher penalty parameter to the minority classes. The method is applied to the Krishna-Godavari basin dataset where seven lithofacies are identified. The entire statistical methodology allows us to transmit the ambiguity from data collected at the well site to the estimated facies profiles. The method's benefits are validated by the field data application results. This contribution provides a useful method for lithofacies classification and reservoir projection using SVM.
更多查看译文
关键词
Lithofacies, Multistage classification, Regularization Parameter, SVM, Well-Log interpretation
AI 理解论文
溯源树
样例
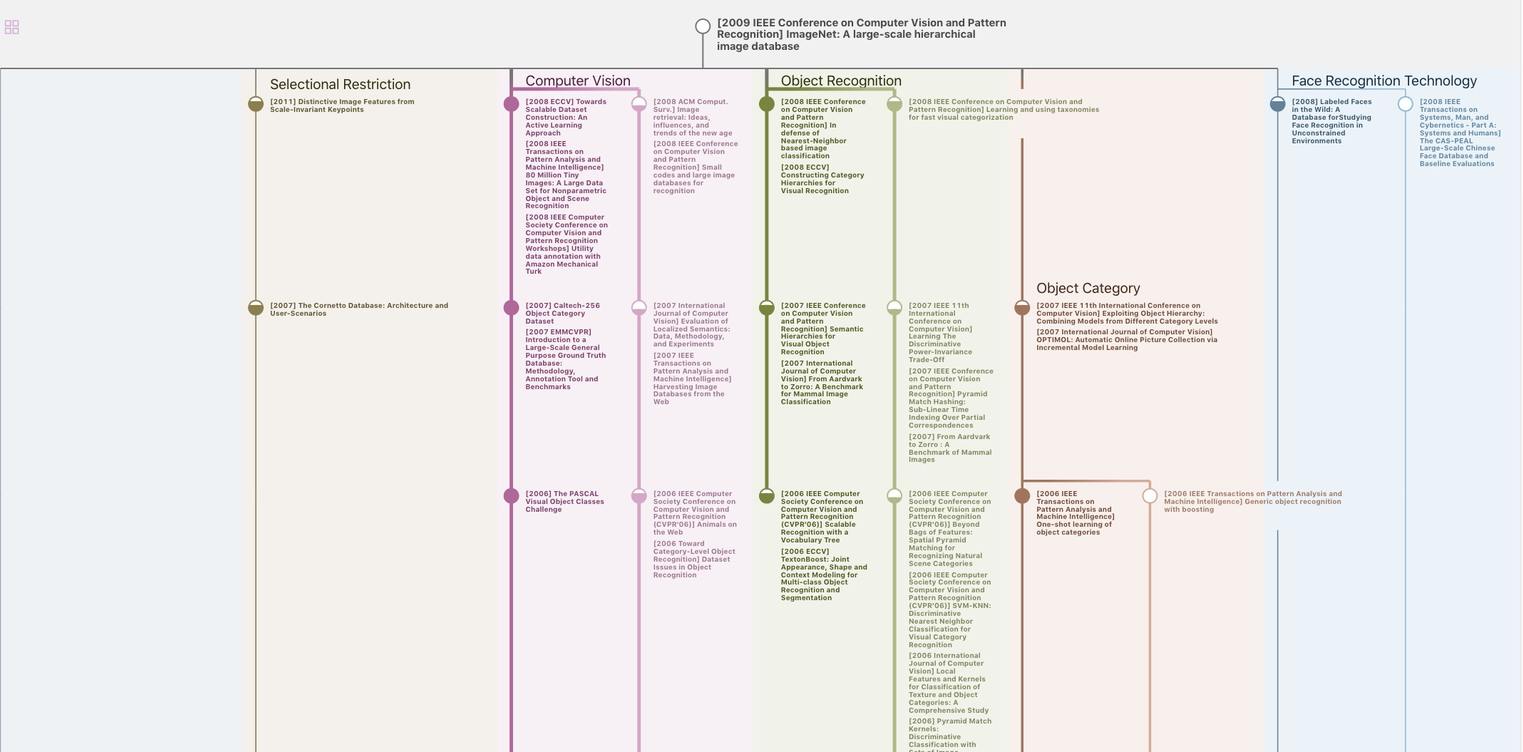
生成溯源树,研究论文发展脉络
Chat Paper
正在生成论文摘要