Stiffness Estimation in Single Degree of Freedom Mechanisms using Regression
IECON 2021 - 47TH ANNUAL CONFERENCE OF THE IEEE INDUSTRIAL ELECTRONICS SOCIETY(2021)
摘要
This paper leverages machine learning principles in combination with an exploratory procedure to estimate the unknown stiffness of a grasped object in a single degree of freedom gripper mechanism. The object stiffness is estimated using regression; sensor measurements of actuator position and actuator current are the regressor inputs, and object stiffness is the regressor output. The proposed approach reduces overall system complexity and cost compared to systems that use tactile sensors to estimate object stiffness. Several datasets are generated using a physics-based simulation model of the system. Regressors are trained on data produced by the simulation model, and their performance is analyzed under variations on simulation model parameters including stick-slip friction parameters; the influence of the actuation command signal used to direct the exploratory procedure is also considered. These regressors are then experimentally evaluated on a representative single degree of freedom mechanism, demonstrating worst-case stiffness estimation errors (based on datasheet spring stiffness values) of 3.4 %.
更多查看译文
关键词
gripper, stiffness, stick-slip friction, estimation, regression, machine learning
AI 理解论文
溯源树
样例
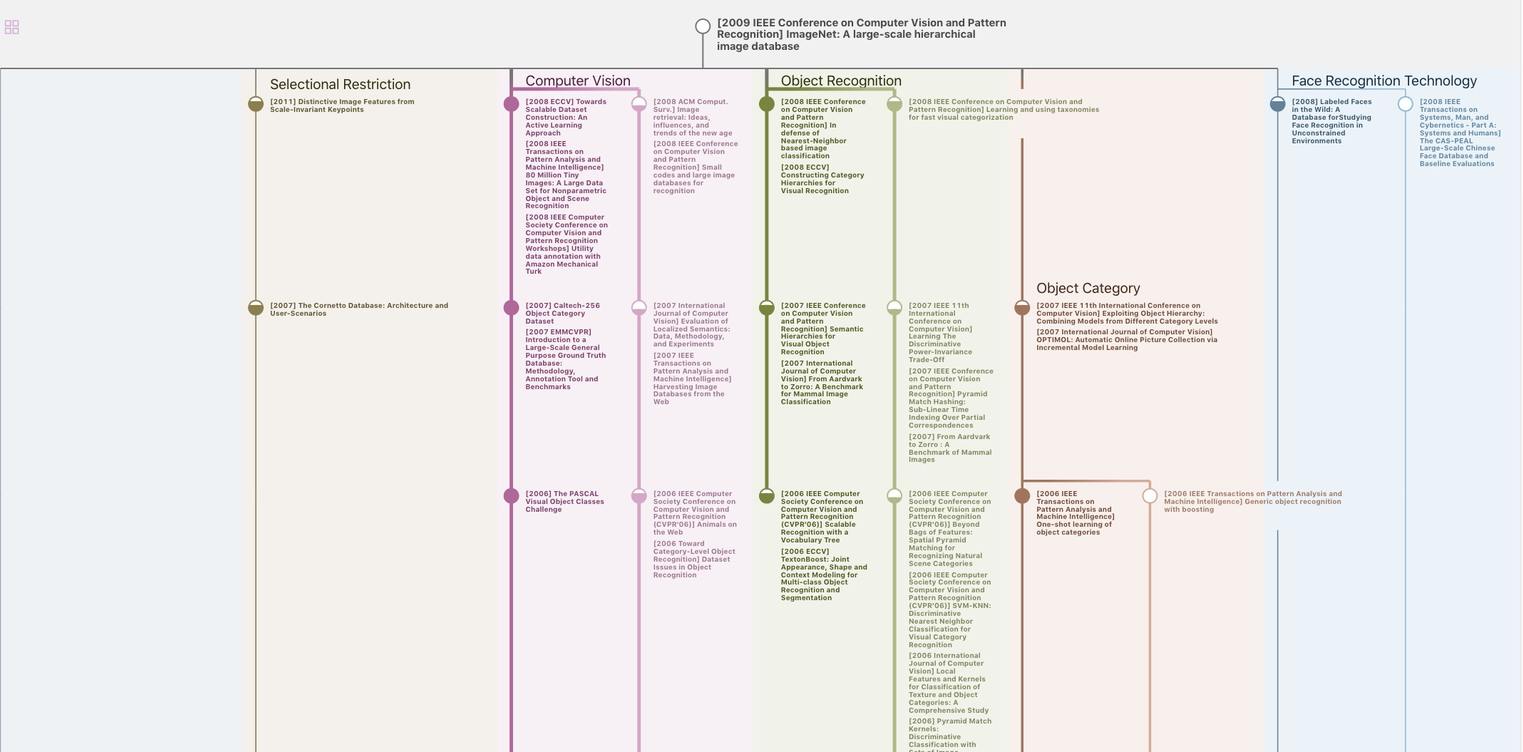
生成溯源树,研究论文发展脉络
Chat Paper
正在生成论文摘要