A New Gradient Free Local Search Mechanism For Constrained Multi-Objective Optimization Problems
SWARM AND EVOLUTIONARY COMPUTATION(2021)
摘要
Multi-objective evolutionary algorithms (MOEAs) are commonly applied to treat multi-objective optimization problems (MOPs) due to their global nature, robustness, and reliability. However, it is also well known that MOEAs need quite a few resources to compute a good approximation of the Pareto set/front. Even more, MOEAs may exhibit difficulties when dealing with highly constrained search spaces. A possible remedy is the hybridization of MOEAs with specialized local search operators; if the local search is based on mathematical programming techniques, gradient information is needed, resulting in relatively high computational cost in many problem instances. In this work, we propose an effective way of computing multi-objective descent directions seeking a compromise between feasibility and the decrement in the objective function values. Moreover, we can obtain this direction so that we do not have to explicitly compute the gradients but extract this information in the best fit manner out of the current population of the MOEA. We demonstrate two hybrid methods, each focused on certain types of problems. Numerical results on some benchmark problems support the benefits of the novel approach.
更多查看译文
关键词
Evolutionary computation, Multi-objective optimization, Descent directions, Constraint handling, Gradient-free local search
AI 理解论文
溯源树
样例
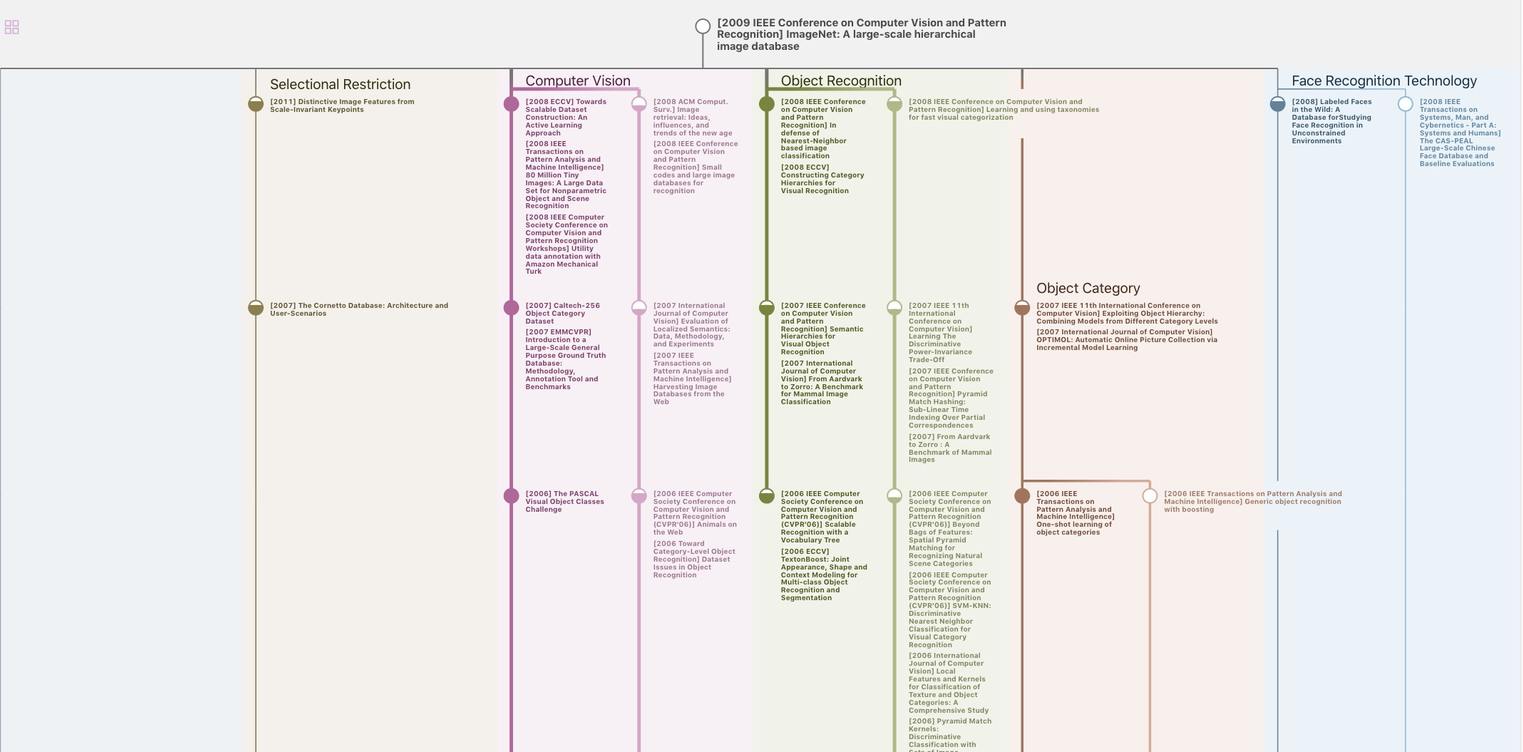
生成溯源树,研究论文发展脉络
Chat Paper
正在生成论文摘要