UAV swarm based radar signal sorting via multi-source data fusion: A deep transfer learning framework
Information Fusion(2022)
摘要
Traditional clustering algorithms can be applied for the pre-sorting step of radar signal sorting. It can effectively dilute the pulse stream and prevent the dense pulse stream from interfering pulse repetition interval (PRI) extraction. However, the pre-sorting deviation will cause interference and missing pulses during the main sorting process. To solve this problem, we deploy the unmanned aerial vehicle (UAV) swarm to monitor reconnaissance areas and put forward a novel deep transfer learning based signal sorting method. The UAV swarm can collect the pulses from different time and spatial domains, and interference and missing pulses in main sorting processing can be relieved dramatically. In our model, we pre-train our model with the data collected from multiple source areas, which corresponds to different areas detected by different parts of UAV swarms. Then we fine-tune our model with the data of the target area. The experimental results prove that the signal sorting accuracy of methods based on deep transfer learning, i.e., YOLO-MobileNet, F-RCNN and cascade RCNN, are higher than that of the baseline methods. In addition, the signal sorting accuracy of traditional methods based on deep learning can be greatly improved with the help of transfer learning.
更多查看译文
关键词
Signal sorting,Deep transfer learning,Data fusion,UAV swarm
AI 理解论文
溯源树
样例
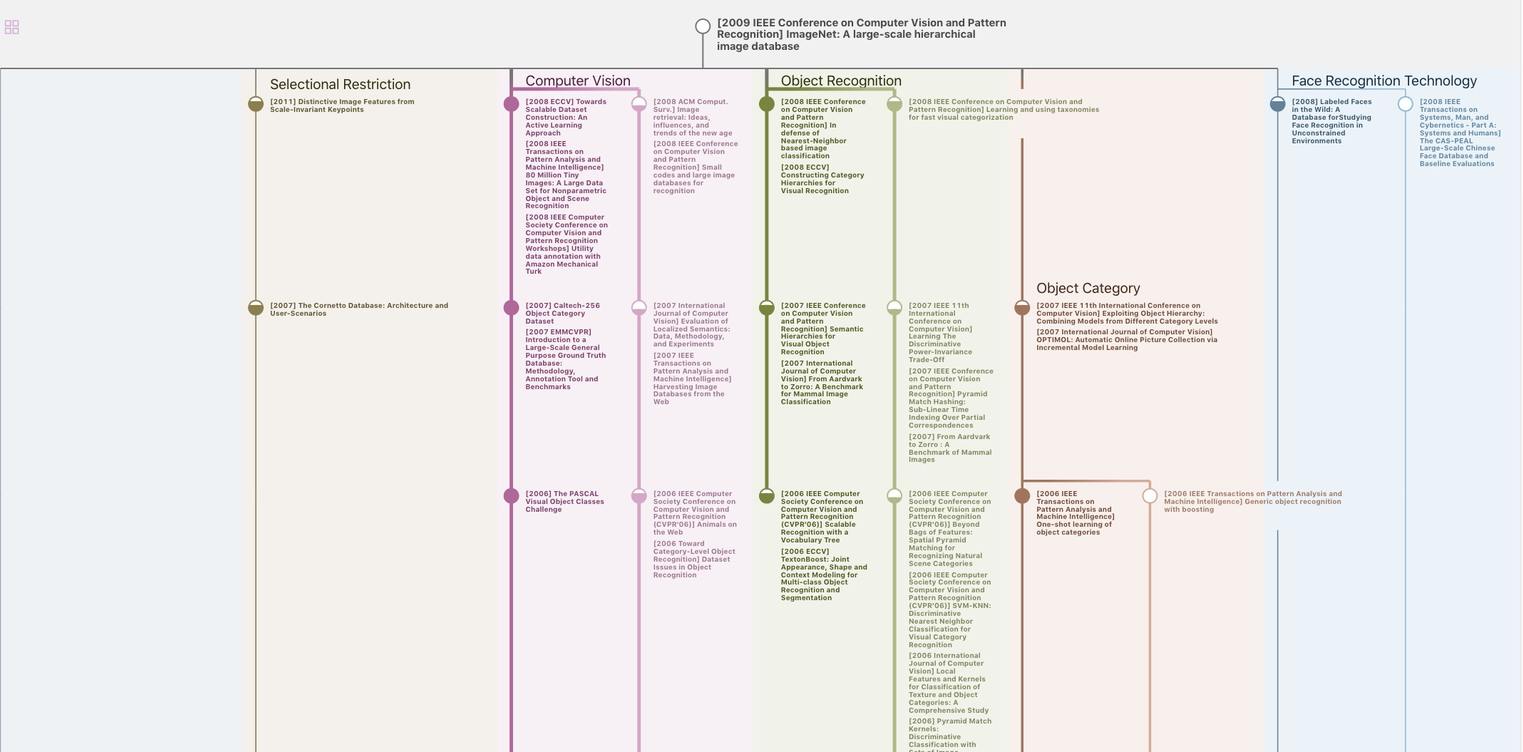
生成溯源树,研究论文发展脉络
Chat Paper
正在生成论文摘要