Beyond Global And Local Multi-Target Learning
INFORMATION SCIENCES(2021)
摘要
In multi-target prediction, an instance has to be classified along multiple target variables at the same time, where each target represents a category or numerical value. There are several strategies to tackle multi-target prediction problems: the local strategy learns a separate model for each target variable independently, while the global strategy learns a single model for all target variables together. Previous studies suggested that the global strategy should be preferred because (1) learning is more efficient, (2) the learned models are more compact, and (3) it overfits much less than the local strategy, as it is harder to overfit on several targets at the same time than on one target. However, it is not clear whether the global strategy exploits correlations between the targets optimally. In this paper, we investigate whether better results can be obtained by learning multiple multi-target models on several partitions of the targets. To answer this question, we first determined alternative partitions using an exhaustive search strategy and a strategy based on a genetic algorithm, and then compared the results of the global and local strategies against these. We used decision trees and random forests as base models. The results show that it is possible to outperform global and local approaches, but finding a good partition without incurring in overfitting remains a challenging task. Crown Copyright (c) 2021 Published by Elsevier Inc. All rights reserved.
更多查看译文
关键词
Multi-target regression, Multi-label classification, Predictive clustering trees, Random forests, Genetic algorithms
AI 理解论文
溯源树
样例
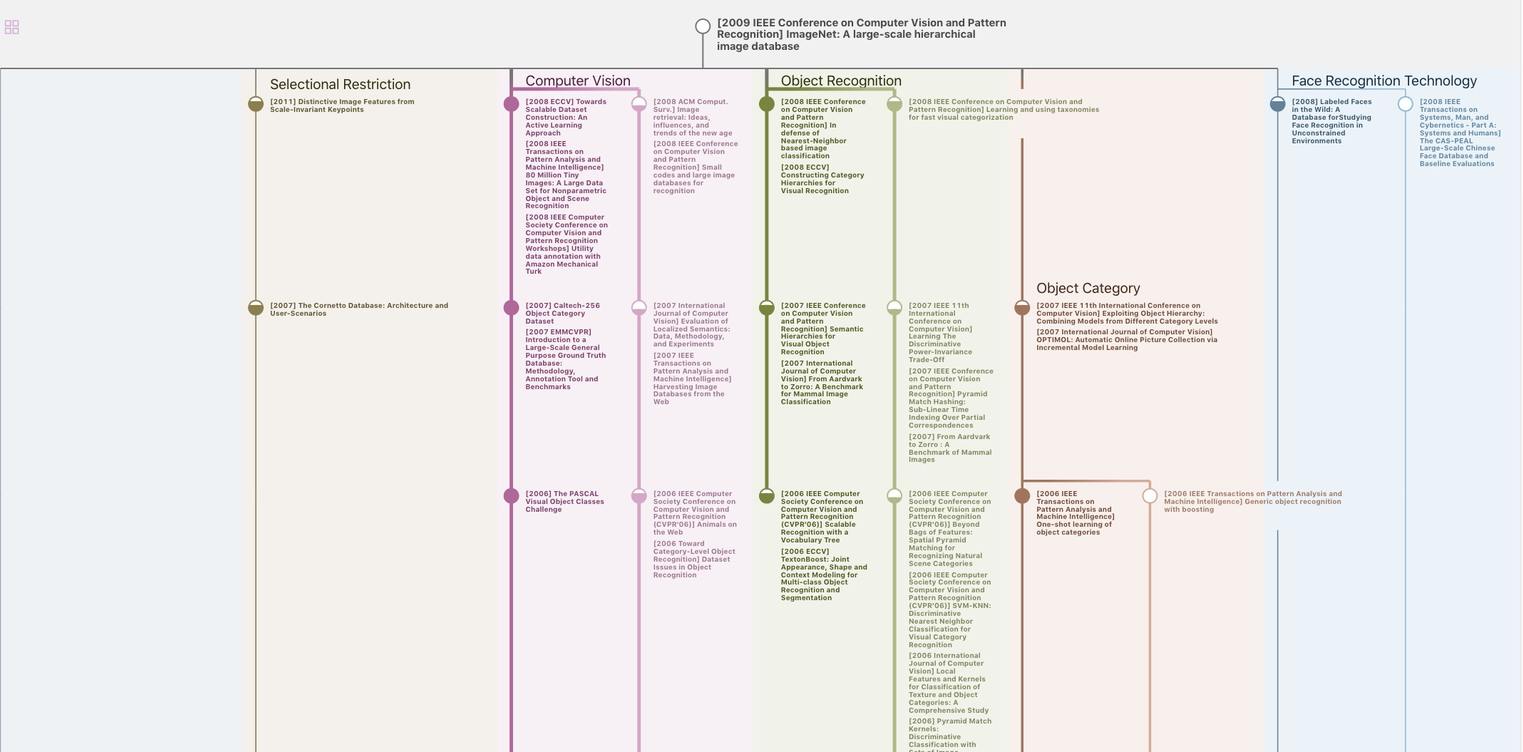
生成溯源树,研究论文发展脉络
Chat Paper
正在生成论文摘要