Addressing Class Overlap Under Imbalanced Distribution: An Improved Method And Two Metrics
SYMMETRY-BASEL(2021)
摘要
Class imbalance, as a phenomenon of asymmetry, has an adverse effect on the performance of most machine learning and overlap is another important factor that affects the classification performance of machine learning algorithms. This paper deals with the two factors simultaneously, addressing the class overlap under imbalanced distribution. In this paper, a theoretical analysis is firstly conducted on the existing class overlap metrics. Then, an improved method and the corresponding metrics to evaluate the class overlap under imbalance distributions are proposed based on the theoretical analysis. A well-known collection of the imbalanced datasets is used to compare the performance of different metrics and the performance is evaluated based on the Pearson correlation coefficient and the xi correlation coefficient. The experimental results demonstrate that the proposed class overlap metrics outperform other compared metrics for the imbalanced datasets and the Pearson correlation coefficient with the AUC metric of eight algorithms can be improved by 34.7488% in average.
更多查看译文
关键词
class overlap, class imbalance, theoretical analysis, machine learning
AI 理解论文
溯源树
样例
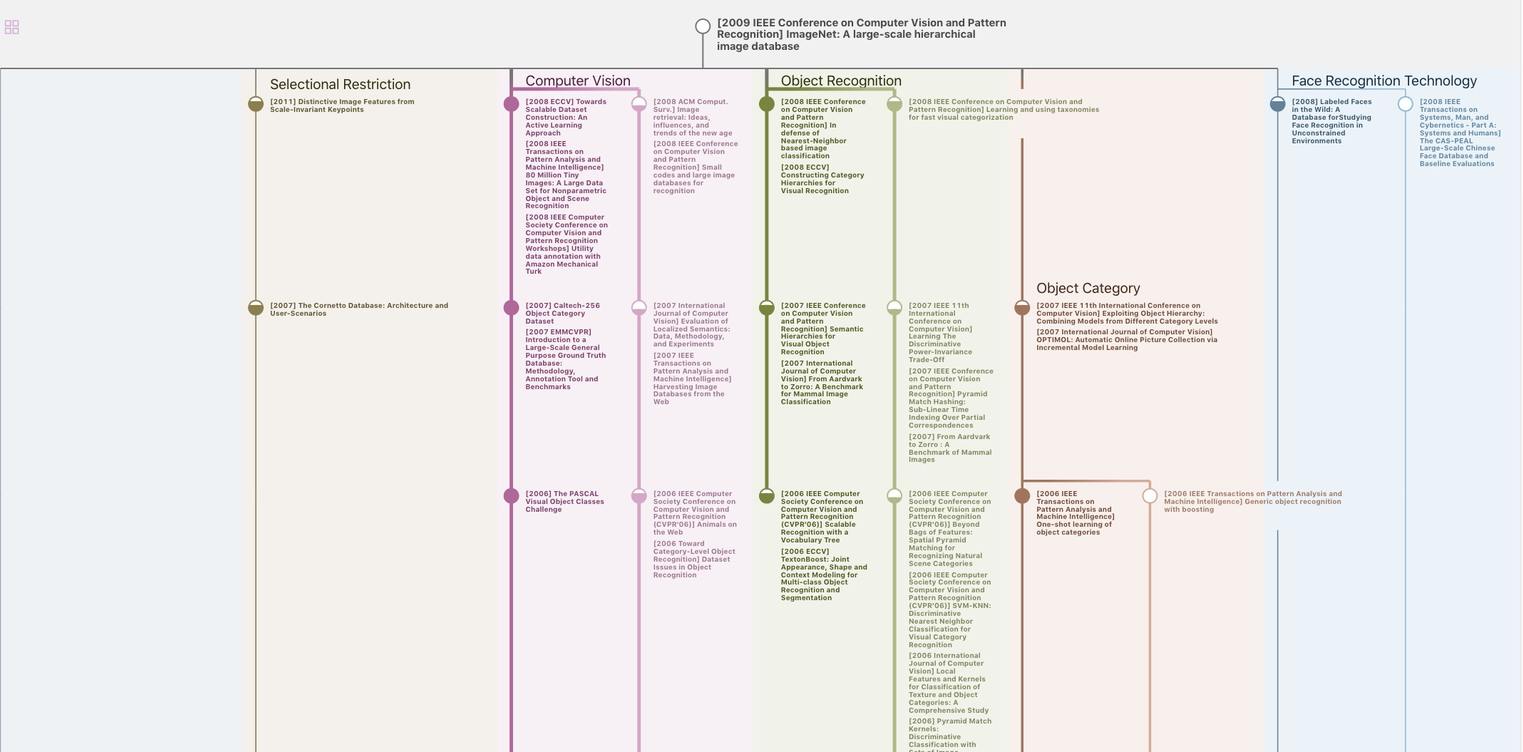
生成溯源树,研究论文发展脉络
Chat Paper
正在生成论文摘要