Efficient Prediction of Microplastic Counts from Mass Measurements
ACS ES&T WATER(2022)
摘要
Microplastics must be characterized and quantified to assess their impact. This is complicated by the time-consuming and error-prone nature of current quantification procedures. This study evaluates the use of machine learning to estimate the number of microplastic particles on the basis of aggregate particle weight measurements. Synthetic data sets are used to test the performance of linear regression, kernel ridge regression, and decision trees. Kernel ridge regression, which achieves the best performance, is tested on several experimental datasets. The numerical results show that the algorithm is better at predicting the counts of larger and more homogeneous samples and that contamination by organics does not significantly increase error. In mixed samples, prediction error is lower for heavier particles, with an error rate comparable to or better than that of traditional manual counting.
更多查看译文
关键词
microplastics,kernel ridge regression,prediction,machine learning
AI 理解论文
溯源树
样例
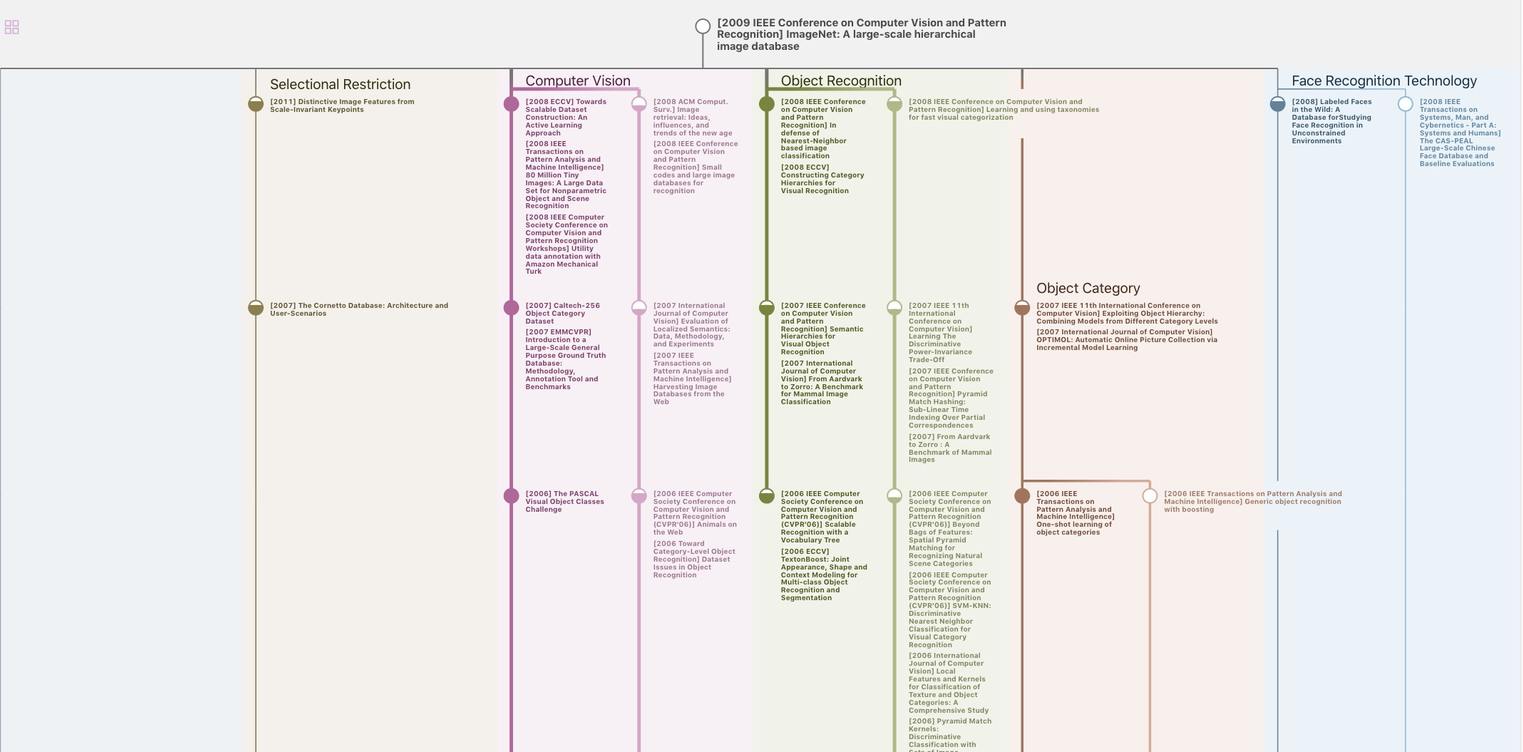
生成溯源树,研究论文发展脉络
Chat Paper
正在生成论文摘要