Open Vocabulary Object Detection with Pseudo Bounding-Box Labels
COMPUTER VISION, ECCV 2022, PT X(2022)
摘要
Despite great progress in object detection, most existing methods work only on a limited set of object categories, due to the tremendous human effort needed for instance-level bounding-box annotations of training data. To alleviate the problem, recent open vocabulary and zero-shot detection methods attempt to detect novel object categories beyond those seen during training. They achieve this goal by training on a pre-defined base categories to induce generalization to novel objects. However, their potential is still constrained by the small set of base categories available for training. To enlarge the set of base classes, we propose a method to automatically generate pseudo bounding-box annotations of diverse objects from large-scale image-caption pairs. Our method leverages the localization ability of pre-trained vision-language models to generate pseudo bounding-box labels and then directly uses them for training object detectors. Experimental results show that our method outperforms the state-of-the-art (SOTA) open vocabulary object detector by 8% AP on COCO novel categories, by 6.3% AP on PASCAL VOC, by 2.3% AP on Objects365 and by 2.8% AP on LVIS. Code is available: https://github.com/salesforce/PB-OVD.
更多查看译文
关键词
Open vocabulary detection,Pseudo bounding-box labels
AI 理解论文
溯源树
样例
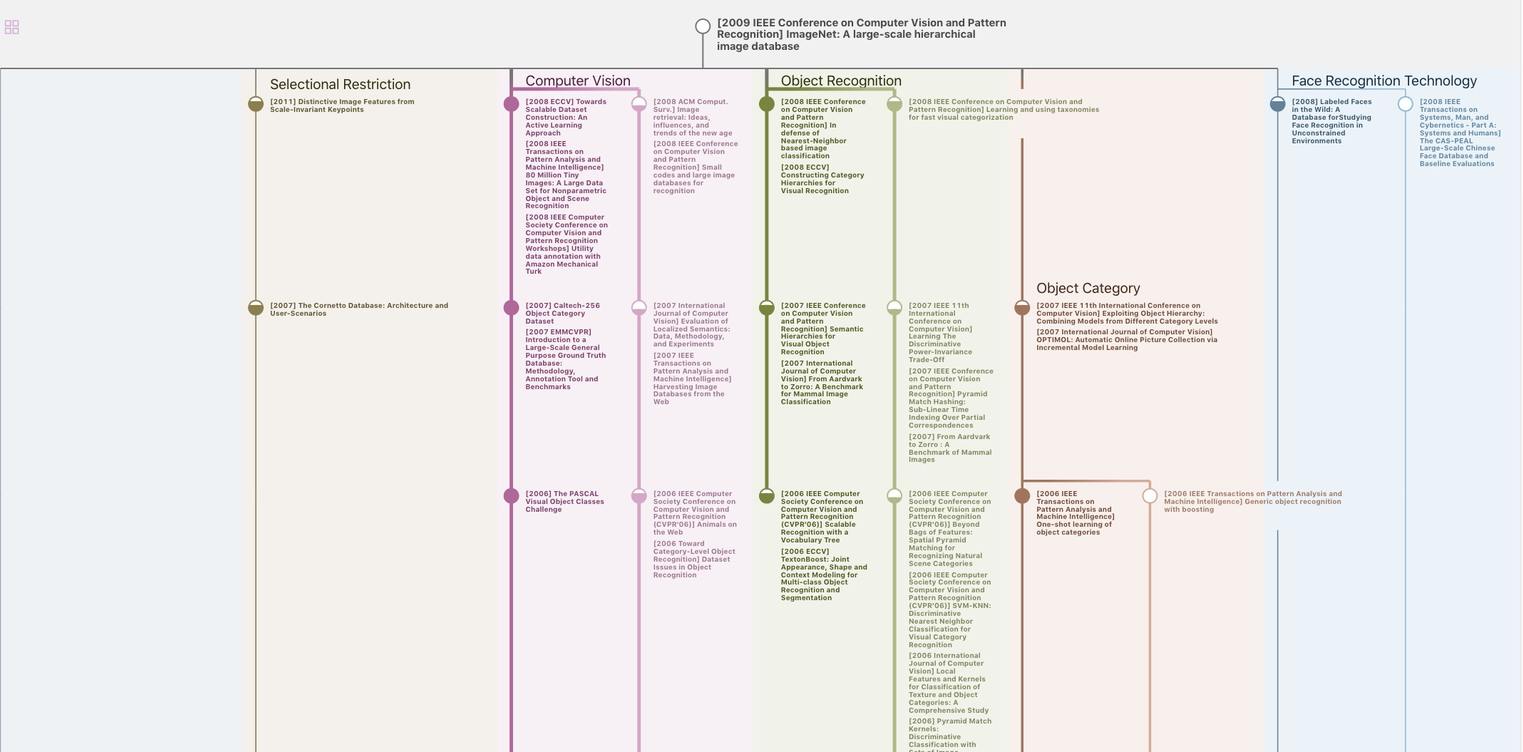
生成溯源树,研究论文发展脉络
Chat Paper
正在生成论文摘要