Interpretable Models via Pairwise Permutations Algorithm
MACHINE LEARNING AND PRINCIPLES AND PRACTICE OF KNOWLEDGE DISCOVERY IN DATABASES, ECML PKDD 2021, PT I(2021)
摘要
One of the most common pitfalls often found in high dimensional biological data sets are correlations between the features. This may lead to statistical and machine learning methodologies overvaluing or undervaluing these correlated predictors, while the truly relevant ones are ignored. In this paper, we will define a new method called pairwise permutation algorithm (PPA) with the aim of mitigating the correlation bias in feature importance values. Firstly, we provide a theoretical foundation, which builds upon previous work on permutation importance. PPA is then applied to a toy data set, where we demonstrate its ability to correct the correlation effect. We further test PPA on a microbiome shotgun dataset, to show that the PPA is already able to obtain biological relevant biomarkers.
更多查看译文
关键词
Permutation, Importance, Correlation, PPA, Diabetes
AI 理解论文
溯源树
样例
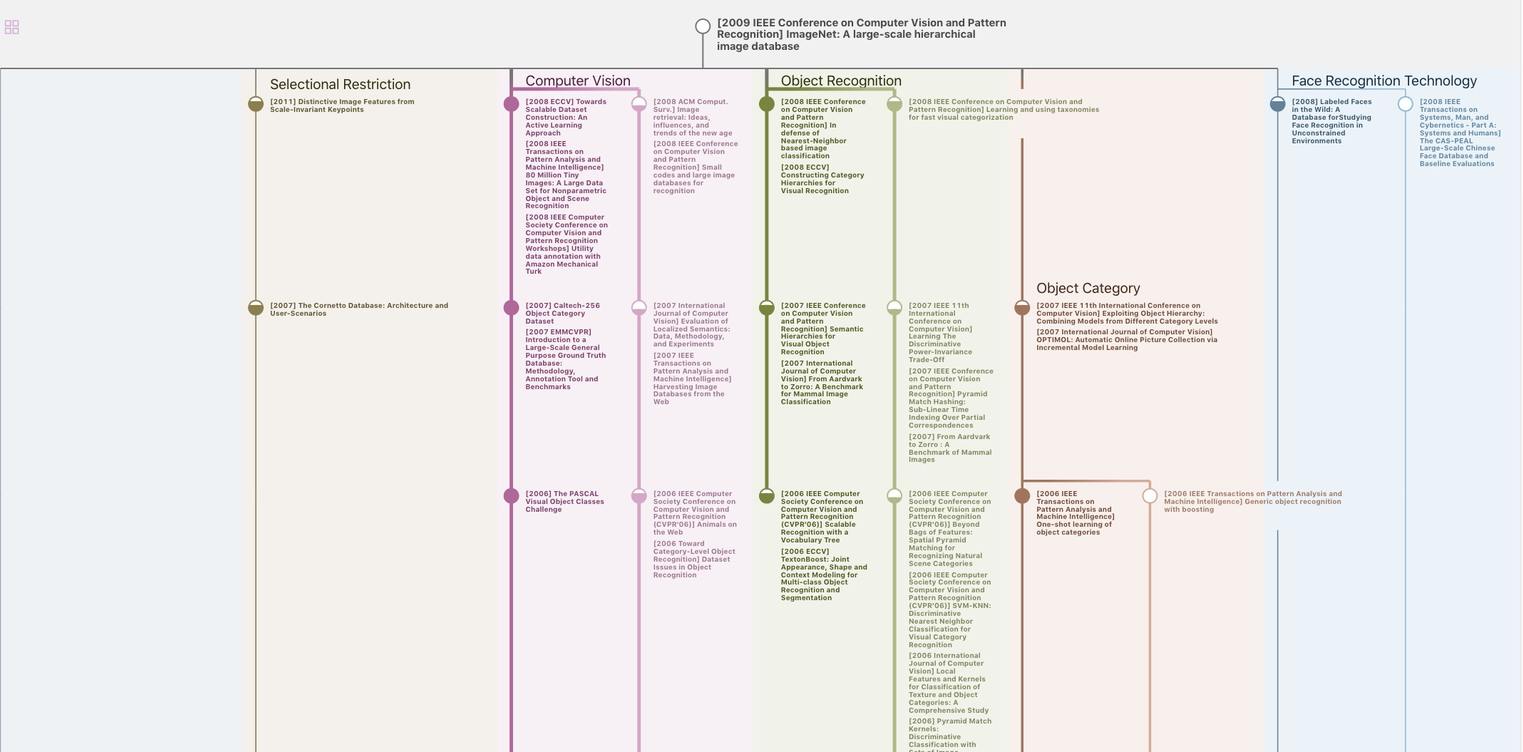
生成溯源树,研究论文发展脉络
Chat Paper
正在生成论文摘要