Sampling To Improve Predictions For Underrepresented Observations In Imbalanced Data
arxiv(2021)
摘要
Data imbalance is common in production data, where controlled production settings require data to fall within a narrow range of variation and data are collected with quality assessment in mind, rather than data analytic insights. This imbalance negatively impacts the predictive performance of models on underrepresented observations. We propose sampling to adjust for this imbalance with the goal of improving the performance of models trained on historical production data. We investigate the use of three sampling approaches to adjust for imbalance. The goal is to downsample the covariates in the training data and subsequently fit a regression model. We investigate how the predictive power of the model changes when using either the sampled or the original data for training. We apply our methods on a large biopharmaceutical manufacturing data set from an advanced simulation of penicillin production and find that fitting a model using the sampled data gives a small reduction in the overall predictive performance, but yields a systematically better performance on underrepresented observations. In addition, the results emphasize the need for alternative, fair, and balanced model evaluations.
更多查看译文
关键词
imbalanced data,underrepresented observations,predictions
AI 理解论文
溯源树
样例
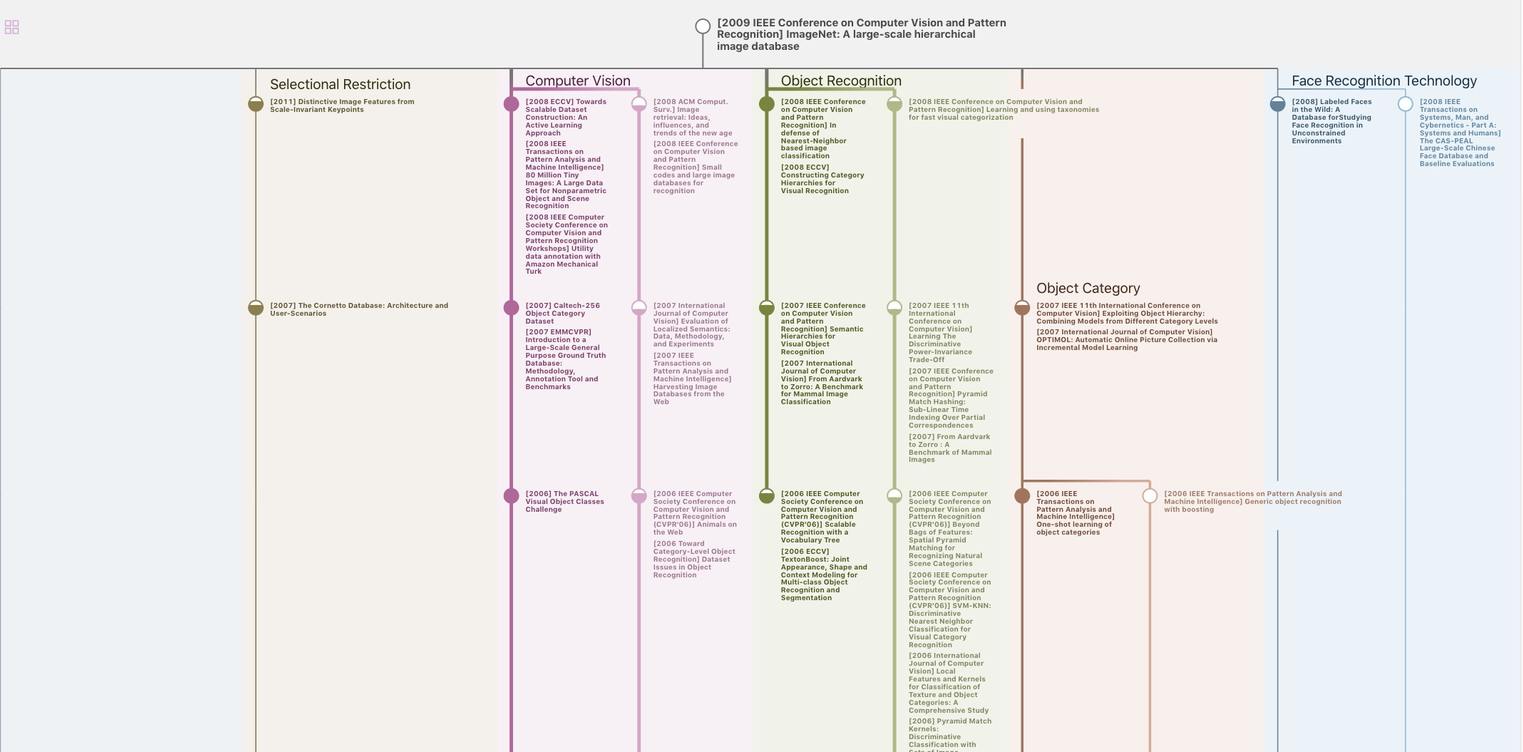
生成溯源树,研究论文发展脉络
Chat Paper
正在生成论文摘要