ARKitScenes: A Diverse Real-World Dataset For 3D Indoor Scene Understanding Using Mobile RGB-D Data
Annual Conference on Neural Information Processing Systems(2022)
摘要
Scene understanding is an active research area. Commercial depth sensors, such as Kinect, have enabled the release of several RGB-D datasets over the past few years which spawned novel methods in 3D scene understanding. More recently with the launch of the LiDAR sensor in Apple's iPads and iPhones, high quality RGB-D data is accessible to millions of people on a device they commonly use. This opens a whole new era in scene understanding for the Computer Vision community as well as app developers. The fundamental research in scene understanding together with the advances in machine learning can now impact people's everyday experiences. However, transforming these scene understanding methods to real-world experiences requires additional innovation and development. In this paper we introduce ARKitScenes. It is not only the first RGB-D dataset that is captured with a now widely available depth sensor, but to our best knowledge, it also is the largest indoor scene understanding data released. In addition to the raw and processed data from the mobile device, ARKitScenes includes high resolution depth maps captured using a stationary laser scanner, as well as manually labeled 3D oriented bounding boxes for a large taxonomy of furniture. We further analyze the usefulness of the data for two downstream tasks: 3D object detection and color-guided depth upsampling. We demonstrate that our dataset can help push the boundaries of existing state-of-the-art methods and it introduces new challenges that better represent real-world scenarios.
更多查看译文
关键词
3d indoor arkitscenes understanding,real-world real-world
AI 理解论文
溯源树
样例
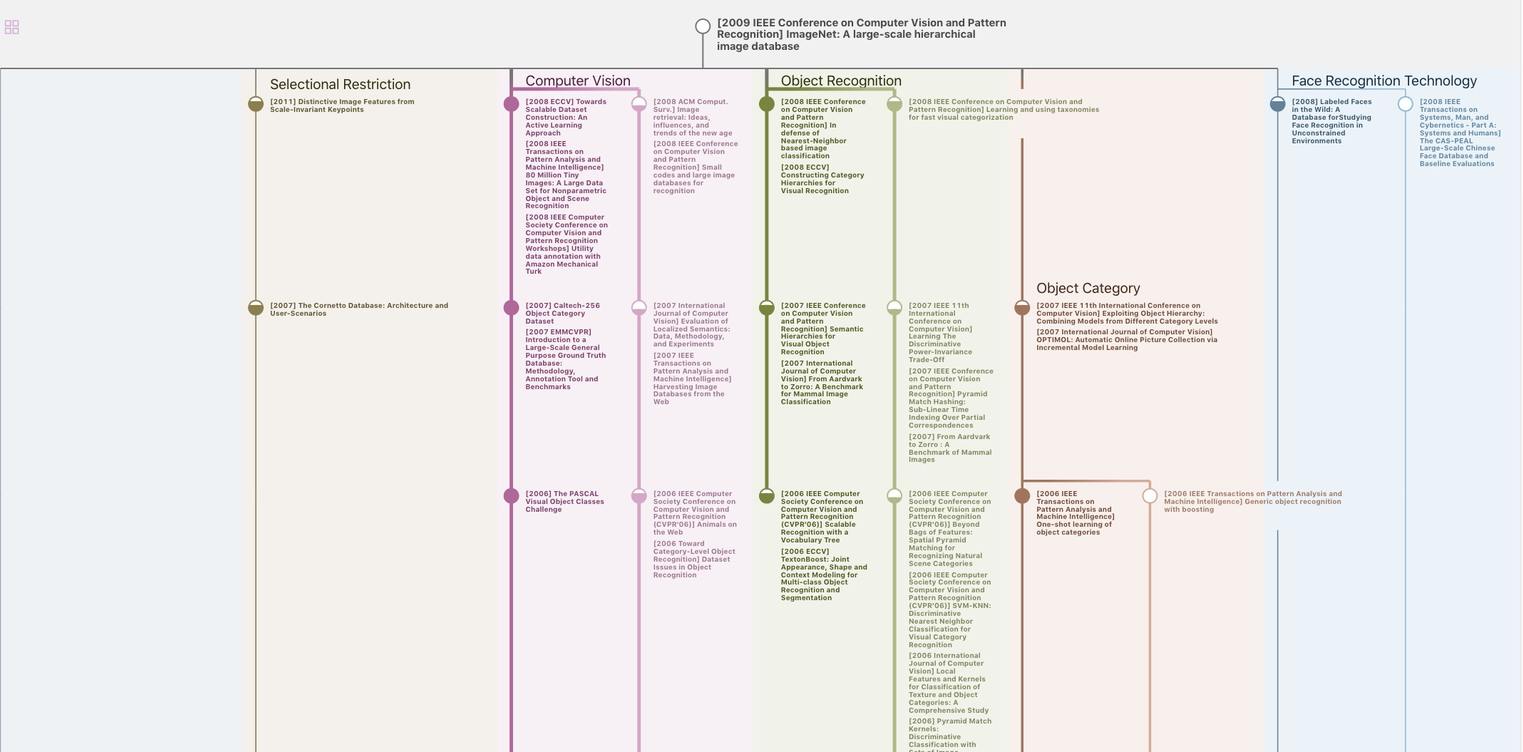
生成溯源树,研究论文发展脉络
Chat Paper
正在生成论文摘要