Improved Pan-Private Stream Density Estimation
arxiv(2021)
摘要
Differential privacy is a rigorous definition for privacy that guarantees that any analysis performed on a sensitive dataset leaks no information about the individuals whose data are contained therein. In this work, we develop new differentially private algorithms to analyze streaming data. Specifically, we consider the problem of estimating the density of a stream of users (or, more generally, elements), which expresses the fraction of all users that actually appear in the stream. We focus on one of the strongest privacy guarantees for the streaming model, namely user-level pan-privacy, which ensures that the privacy of any user is protected, even against an adversary that observes, on rare occasions, the internal state of the algorithm. Our proposed algorithms employ optimally all the allocated privacy budget, are specially tailored for the streaming model, and, hence, outperform both theoretically and experimentally the conventional sampling-based approach.
更多查看译文
关键词
stream,estimation,density,pan-private
AI 理解论文
溯源树
样例
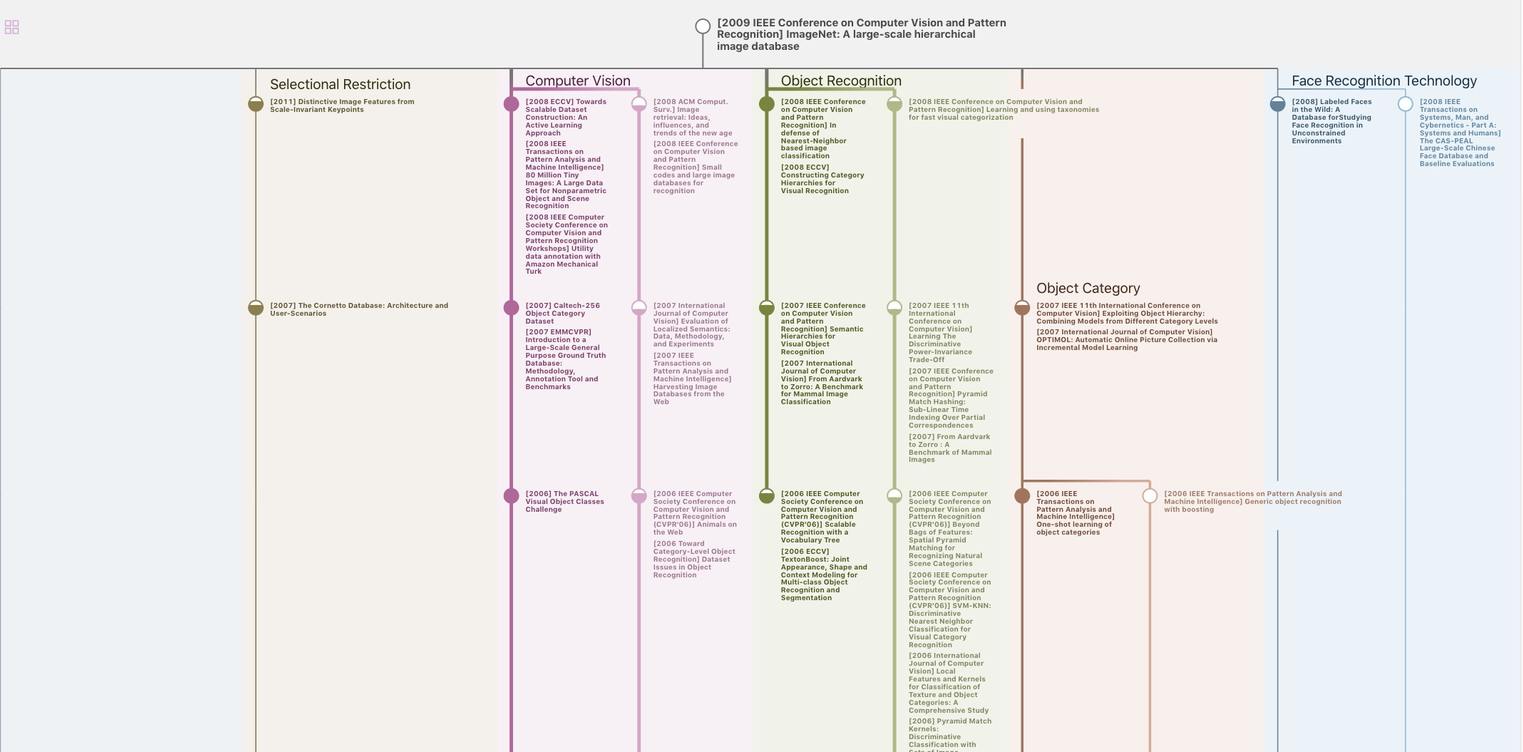
生成溯源树,研究论文发展脉络
Chat Paper
正在生成论文摘要