Optimising biomedical relationship extraction with BioBERT
biorxiv(2020)
摘要
Text mining is widely used within the life sciences as an evidence stream for inferring relationships between biological entities. In most cases, conventional string matching is used to identify cooccurrences of given entities within sentences. This limits the utility of text mining results, as they tend to contain significant noise due to weak inclusion criteria. We show that, in the indicative case of protein-protein interactions (PPIs), the majority of sentences containing cooccurrences (∽75%) do not describe any causal relationship. We further demonstrate the feasibility of fine tuning a strong domain-specific language model, BioBERT, to analyse sentences containing cooccurrences and accurately (F1 score: 88.95%) identify functional links between proteins. These strong results come in spite of the deep complexity of the language involved, which limits the accuracy even of expert curators. We establish guidelines for best practices in data creation to this end, including an examination of inter-annotator agreement, of semisupervision, and of rules based alternatives to manual curation, and explore the potential for downstream use of the model to accelerate curation of interactions in the SIGNOR database of causal protein interactions and the IntAct database of experimental evidence for physical protein interactions.
更多查看译文
关键词
biomedical relationship extraction
AI 理解论文
溯源树
样例
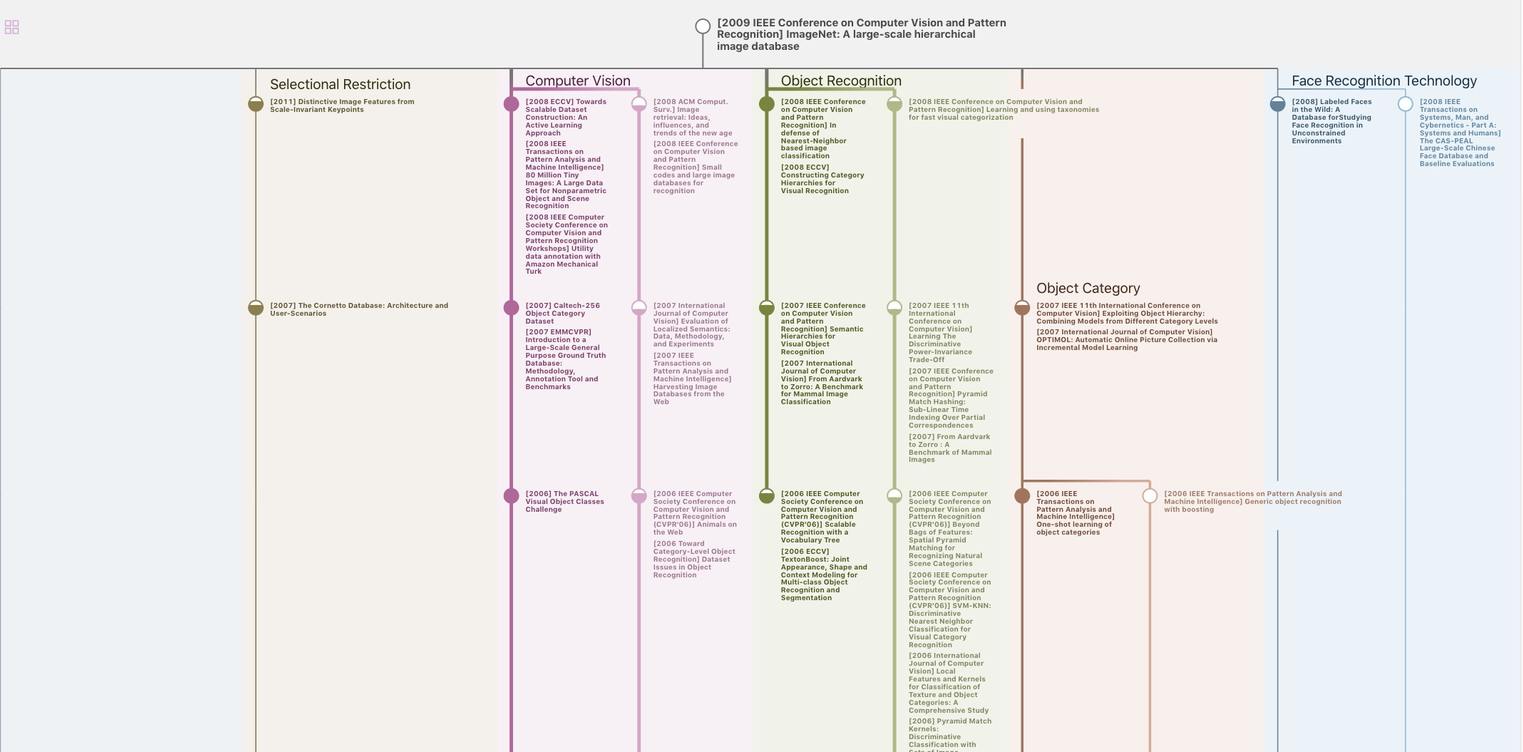
生成溯源树,研究论文发展脉络
Chat Paper
正在生成论文摘要