Wasserstein Coupled Graph Learning for Cross-Modal Retrieval.
ICCV(2021)
摘要
Graphs play an important role in cross-modal image-text understanding as they characterize the intrinsic structure which is robust and crucial for the measurement of cross-modal similarity. In this work, we propose a Wasserstein Coupled Graph Learning (WCGL) method to deal with the cross-modal retrieval task. First, graphs are constructed according to two input cross-modal samples separately, and passed through the corresponding graph encoders to extract robust features. Then, a Wasserstein coupled dictionary, containing multiple pairs of counterpart graph keys with each key corresponding to one modality, is constructed for further feature learning. Based on this dictionary, the input graphs can be transformed into the dictionary space to facilitate the similarity measurement through a Wasserstein Graph Embedding (WGE) process. The WGE could capture the graph correlation between the input and each corresponding key through optimal transport, and hence well characterize the inter-graph structural relationship. To further achieve discriminant graph learning, we specifically define a Wasserstein discriminant loss on the coupled graph keys to make the intra-class (counterpart) keys more compact and inter-class (non-counterpart) keys more dispersed, which further promotes the final cross-modal retrieval task. Experimental results demonstrate the effectiveness and state-of-the-art performance.
更多查看译文
关键词
Vision + language
AI 理解论文
溯源树
样例
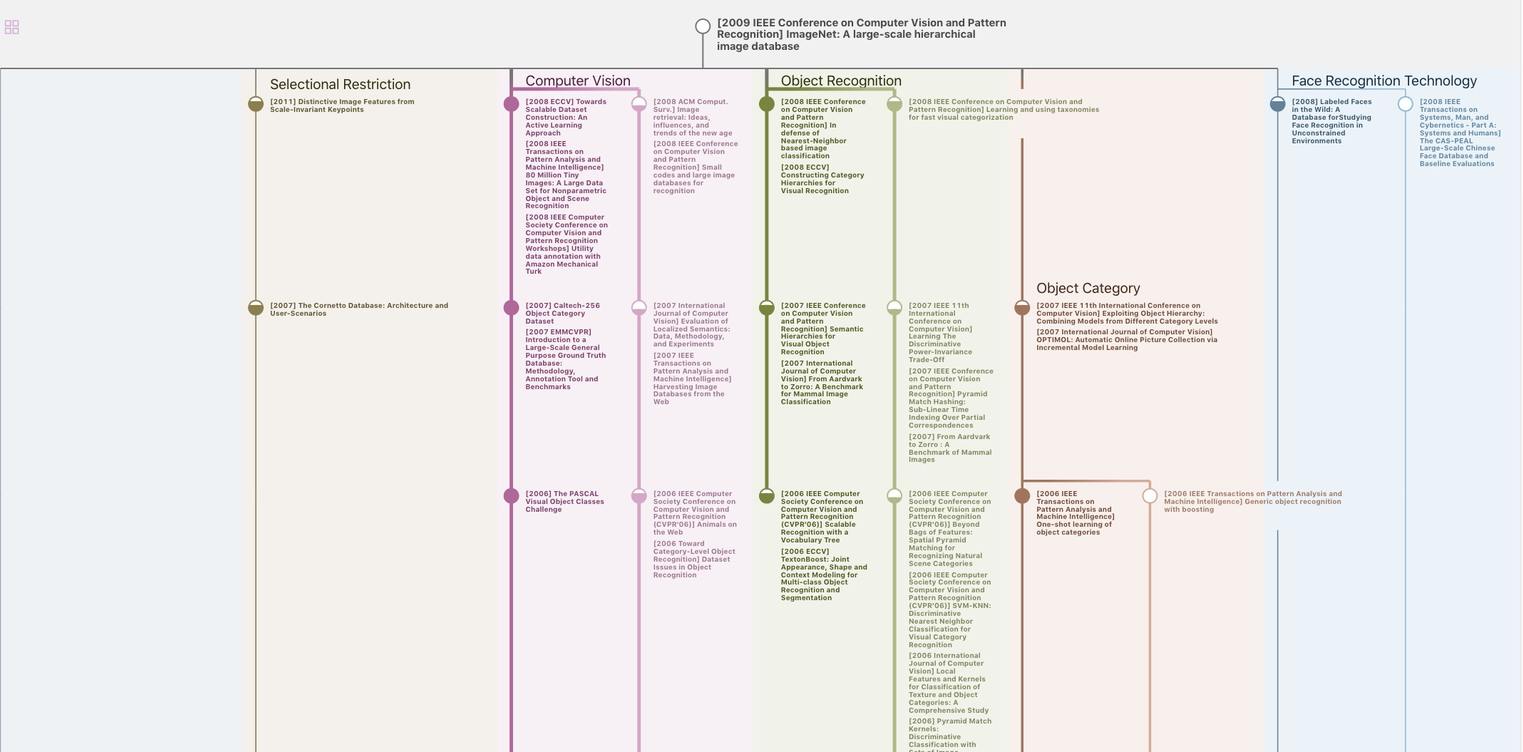
生成溯源树,研究论文发展脉络
Chat Paper
正在生成论文摘要