Deep Symmetric Network for Underexposed Image Enhancement with Recurrent Attentional Learning
ICCV(2021)
摘要
Underexposed image enhancement is of importance in many research domains. In this paper, we take this problem as image feature transformation between the underexposed image and its paired enhanced version, and we propose a deep symmetric network for the issue. Our symmetric network adapts invertible neural networks (INN) for bidirectional feature learning between images, and to ensure the mutual propagation invertible we specifically construct two pairs of encoder-decoder with the same pretrained parameters. This invertible mechanism with bidirectional feature transformations enable us to both avoid colour bias and recover the content effectively for image enhancement. In addition, we propose a new recurrent residual-attention module (RRAM), where the recurrent learning network is designed to gradually perform the desired colour adjustments. Ablation experiments are executed to show the role of each component of our new architecture. We conduct a large number of experiments on two datasets to demonstrate that our method achieves the state-of-the-art effect in underexposed image enhancement. Code is available at https://www.shaopinglu.net/proj-iccv21/ImageEnhancement.html.
更多查看译文
关键词
Image and video retrieval,Image and video synthesis,Machine learning architectures and formulations
AI 理解论文
溯源树
样例
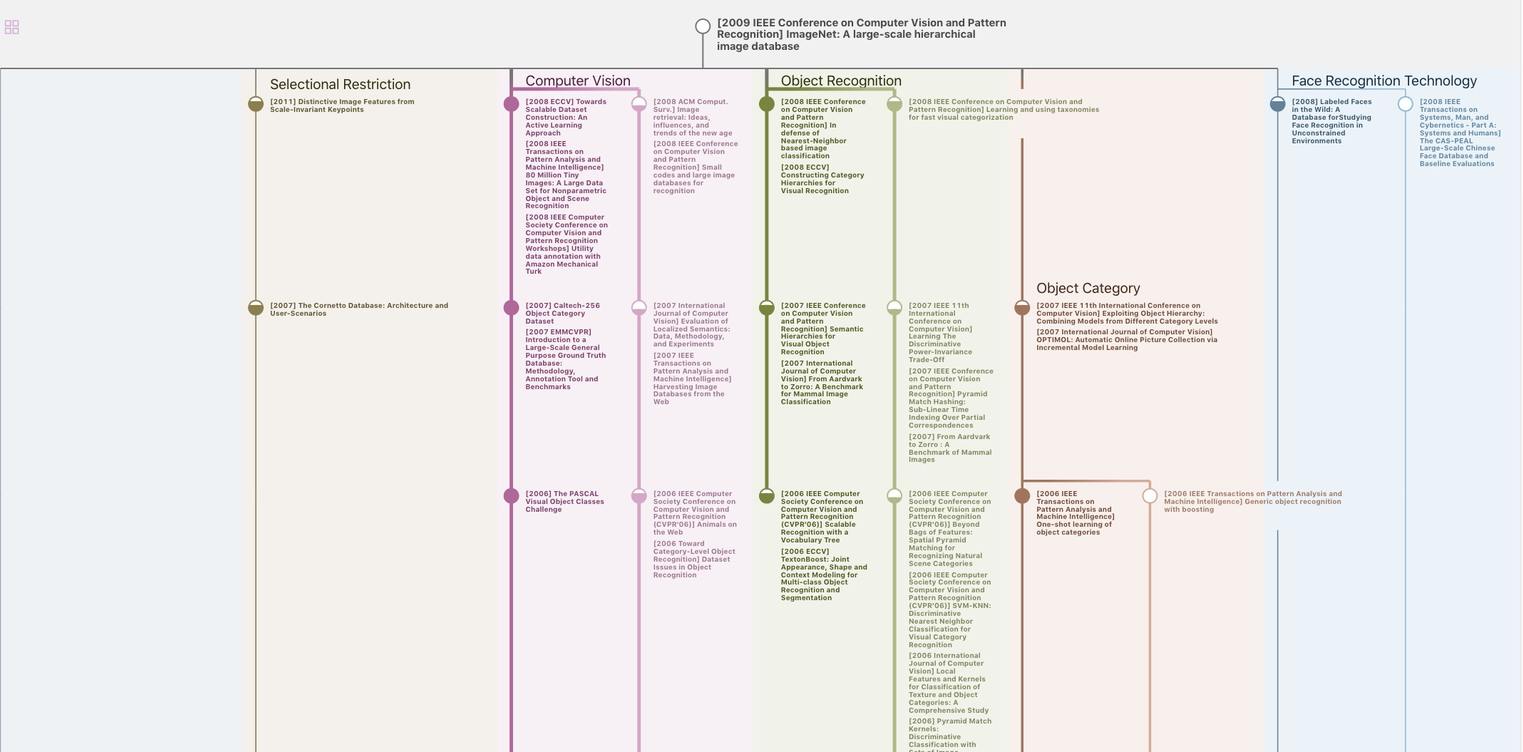
生成溯源树,研究论文发展脉络
Chat Paper
正在生成论文摘要