Self-Motivated Communication Agent for Real-World Vision-Dialog Navigation
ICCV(2021)
摘要
Vision-Dialog Navigation (VDN) requires an agent to ask questions and navigate following the human responses to find target objects. Conventional approaches are only allowed to ask questions at predefined locations, which are built upon expensive dialogue annotations, and inconvenience the real-word human-robot communication and cooperation. In this paper, we propose a Self-Motivated Communication Agent (SCoA) that learns whether and what to communicate with human adaptively to acquire instructive information for realizing dialogue annotation-free navigation and enhancing the transferability in real-world unseen environment. Specifically, we introduce a whether-to-ask (WeTA) policy, together with uncertainty of which action to choose, to indicate whether the agent should ask a question. Then, a what-to-ask (WaTA) policy is proposed, in which, along with the oracle’s answers, the agent learns to score question candidates so as to pick up the most informative one for navigation, and meanwhile mimic oracle’s answering. Thus, the agent can navigate in a self-Q&A manner even in real-world environment where the human assistance is often unavailable. Through joint optimization of communication and navigation in a unified imitation learning and reinforcement learning framework, SCoA asks a question if necessary and obtains a hint for guiding the agent to move towards the target with less communication cost. Experiments on seen and unseen environments demonstrate that SCoA shows not only superior performance over existing baselines without dialog annotations, but also competing results compared with rich dialog annotations based counterparts.
更多查看译文
关键词
Vision + language
AI 理解论文
溯源树
样例
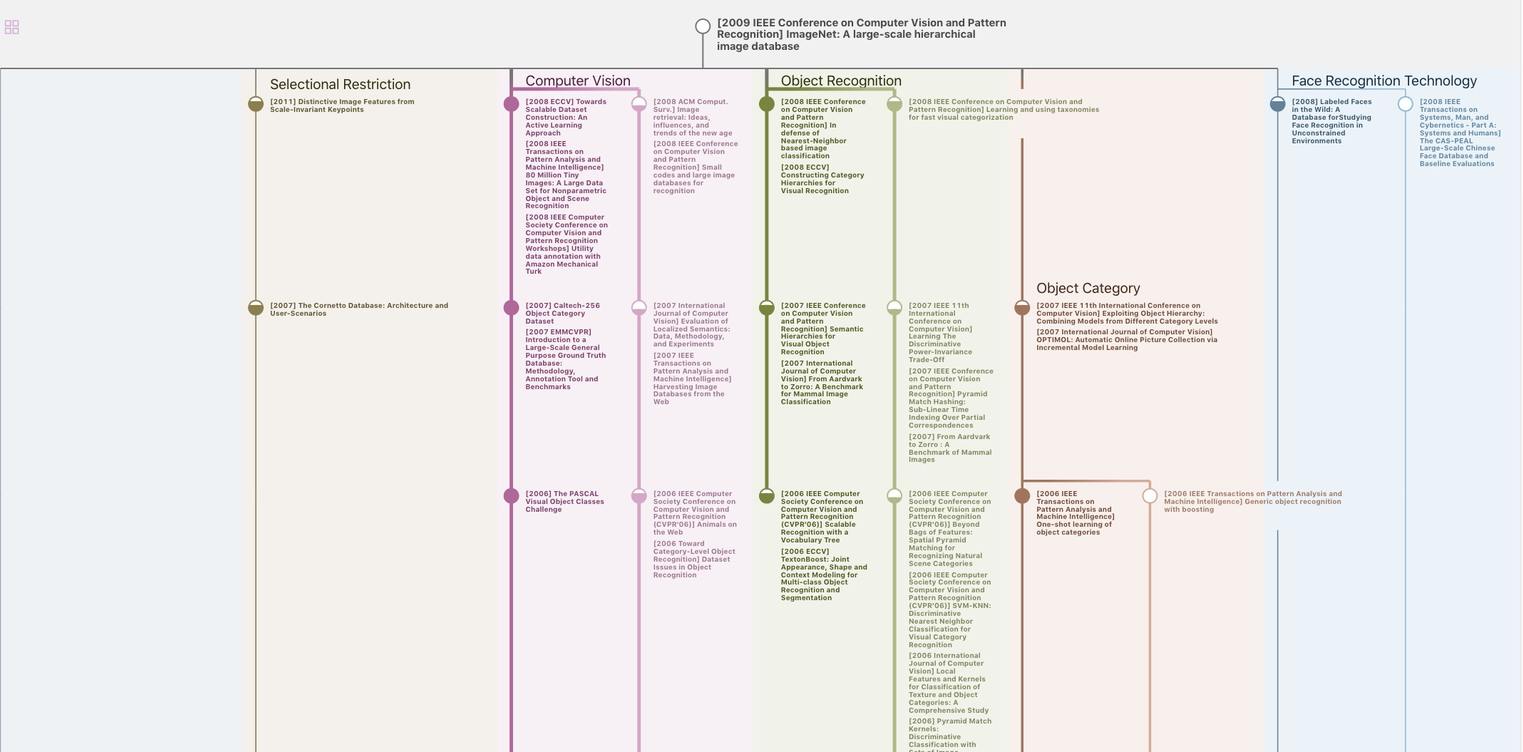
生成溯源树,研究论文发展脉络
Chat Paper
正在生成论文摘要