ORCCA: Optimal Randomized Canonical Correlation Analysis
IEEE transactions on neural networks and learning systems(2023)
摘要
Random features approach has been widely used for kernel approximation in large-scale machine learning. A number of recent studies have explored data-dependent sampling of features, modifying the stochastic oracle from which random features are sampled. While proposed techniques in this realm improve the approximation, their suitability is often verified on a single learning task. In this article, we propose a task-specific scoring rule for selecting random features, which can be employed for different applications with some adjustments. We restrict our attention to canonical correlation analysis (CCA) and provide a novel, principled guide for finding the score function maximizing the canonical correlations. We prove that this method, called optimal randomized CCA (ORCCA), can outperform (in expectation) the corresponding kernel CCA with a default kernel. Numerical experiments verify that ORCCA is significantly superior to other approximation techniques in the CCA task.
更多查看译文
关键词
Kernel,Correlation,Task analysis,Machine learning,Costs,Supervised learning,Computational efficiency,Canonical correlation analysis (CCA),kernel approximation,kernel methods,random features
AI 理解论文
溯源树
样例
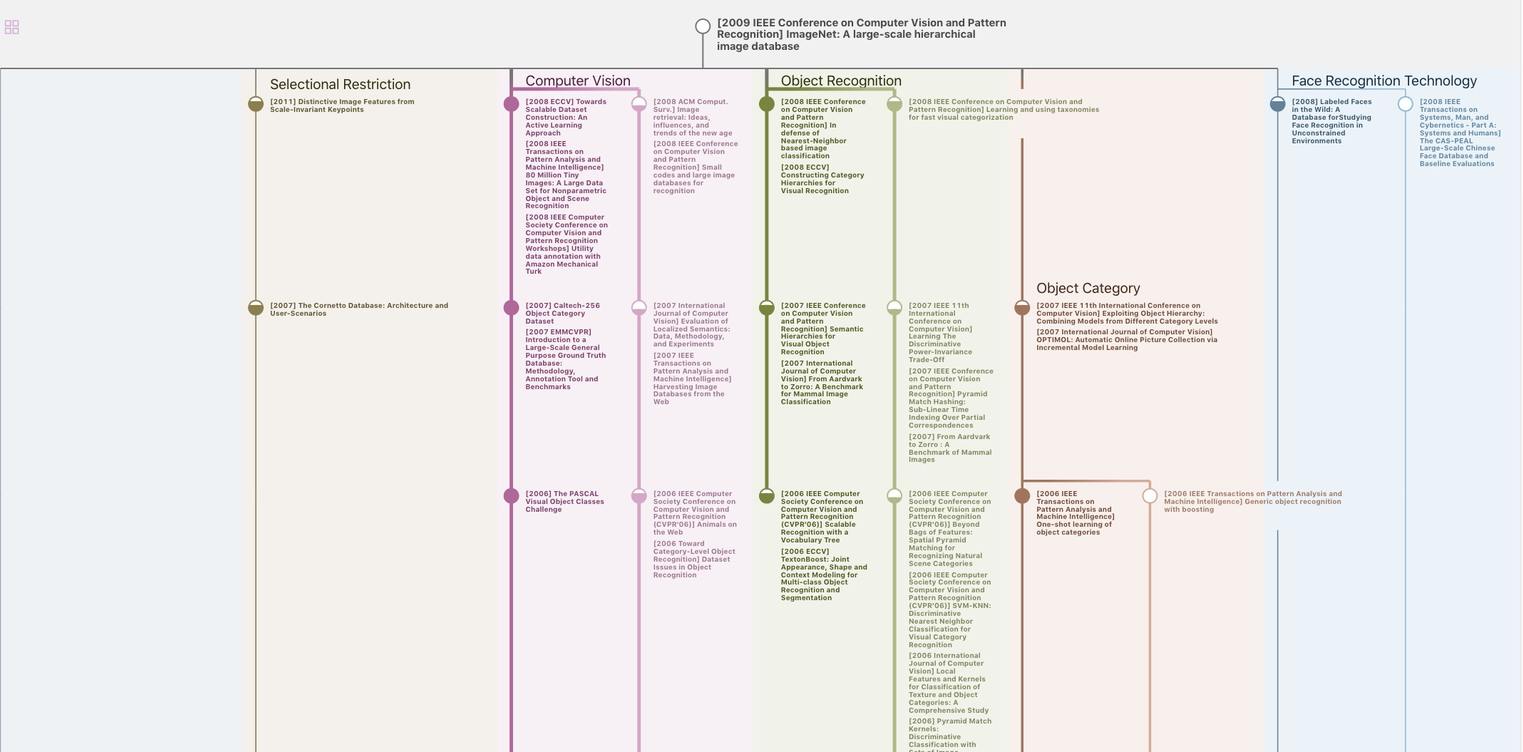
生成溯源树,研究论文发展脉络
Chat Paper
正在生成论文摘要