Distilling the Knowledge of Multiscale Densely Connected Deep Networks in Mechanical Intelligent Diagnosis
WIRELESS COMMUNICATIONS & MOBILE COMPUTING(2021)
摘要
At present, deep neural network (DNN) technology is often used in intelligent diagnosis research. However, the huge amount of calculation of DNN makes it difficult to apply in industrial practice. In this paper, an advanced multiscale dense connection deep network MSDC-NET is designed. A well-designed multiscale parallel branch module is used in the network. This module can greatly improve the acceptance domain of MSDC-NET, so as to learn useful information from input samples more effectively. Based on the inspiration of Densely Connected Convolutional Networks, MSDC-NET designed a similar dense connection technology, so that the model will not have the problem of gradient vanishing because of the deep network. The experimental data of MSDC-NET on MFPT, SEU, and Pu datasets show that our method has higher performance than other latest technologies. At the same time, we carried out knowledge distillation based on the high-precision classification level of MSDC-NET, which makes the diagnosis ability and robustness of the lightweight CNN model improve significantly.
更多查看译文
AI 理解论文
溯源树
样例
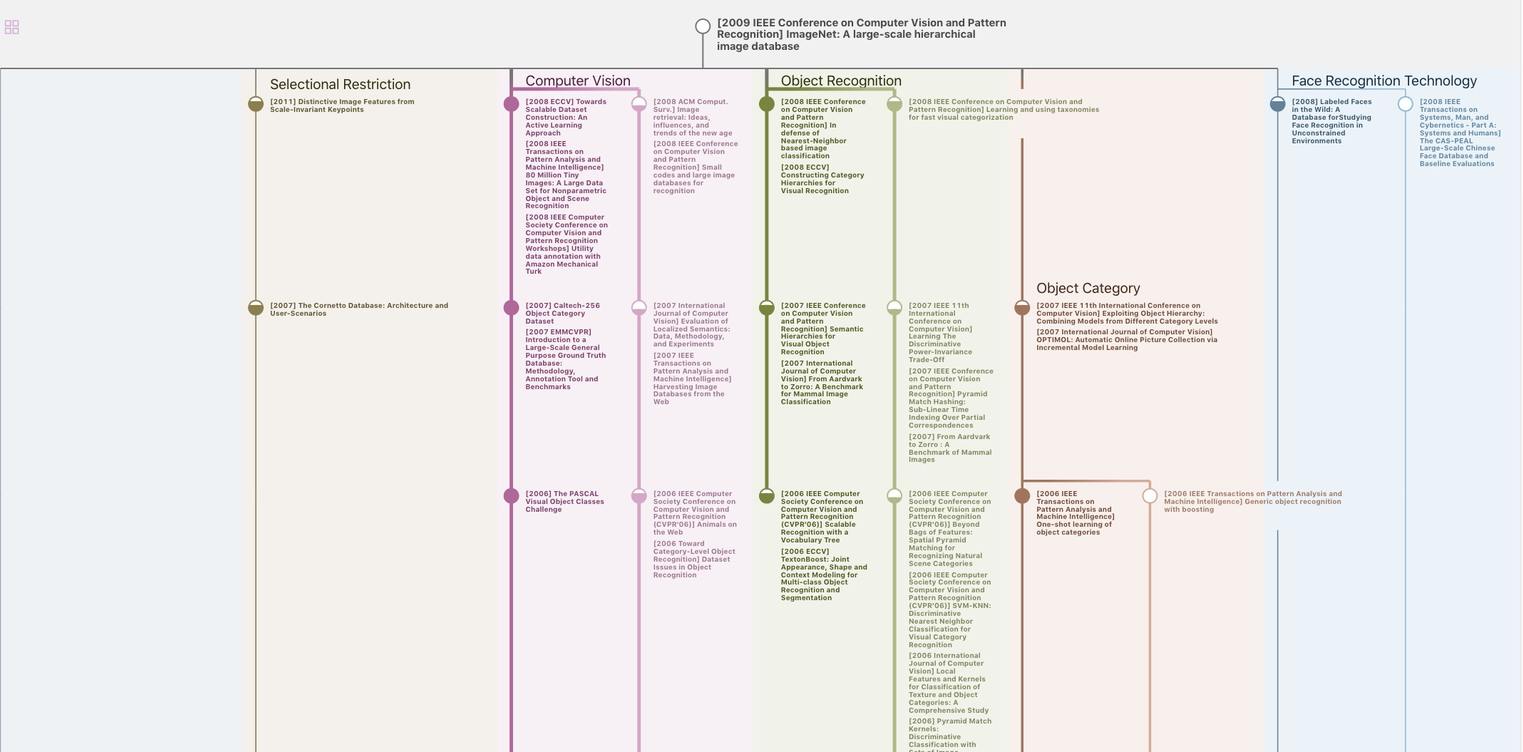
生成溯源树,研究论文发展脉络
Chat Paper
正在生成论文摘要