LatRAIVF: An Infrared and Visible Image Fusion Method Based on Latent Regression and Adversarial Training
IEEE TRANSACTIONS ON INSTRUMENTATION AND MEASUREMENT(2021)
摘要
In this article, we propose a novel method for infrared and visible image fusion based on latent regression and adversarial training, which is named as LatRAIVF. Compared to existing deep learning (DL)-based image fusion method that only focuses on the spatial information, we consider to utilize the information provided by high-level feature maps from latent space, which can guide the network to learn about semantically important feature information. The proposed method is based on the framework of conditional generative adversarial network (GAN), and two encoders are adopted to learn the respective semantic latent representations for the infrared and visible images, which are then combined by max-selection strategy and input into the decoder, with skip connections between the corresponding layers of the encoder and the decoder, to achieve the fused image. Apart from the adversarial process that enables the fused image to obtain more realistic details, we design two branches to constrain the generation of the image: a content loss to make the fused image close to the label image, and a latent regression loss to ensure the fused image with salient features from the infrared and visible images. Due to the lack of physical ground-truth fused images in public infrared and visible image datasets and the difficulties in defining desired fused image, we make use of existing RGB-D dataset to synthesize an infrared and visible image dataset with ground truths based on the widely used optical model for better network training. Comparison experiments show that the fused results of the proposed method can transfer meaningful features from the source image and provide good fusion quality.
更多查看译文
关键词
Deep learning (DL),generative adversarial networks (GANs),image fusion,infrared and visible image,latent space regression
AI 理解论文
溯源树
样例
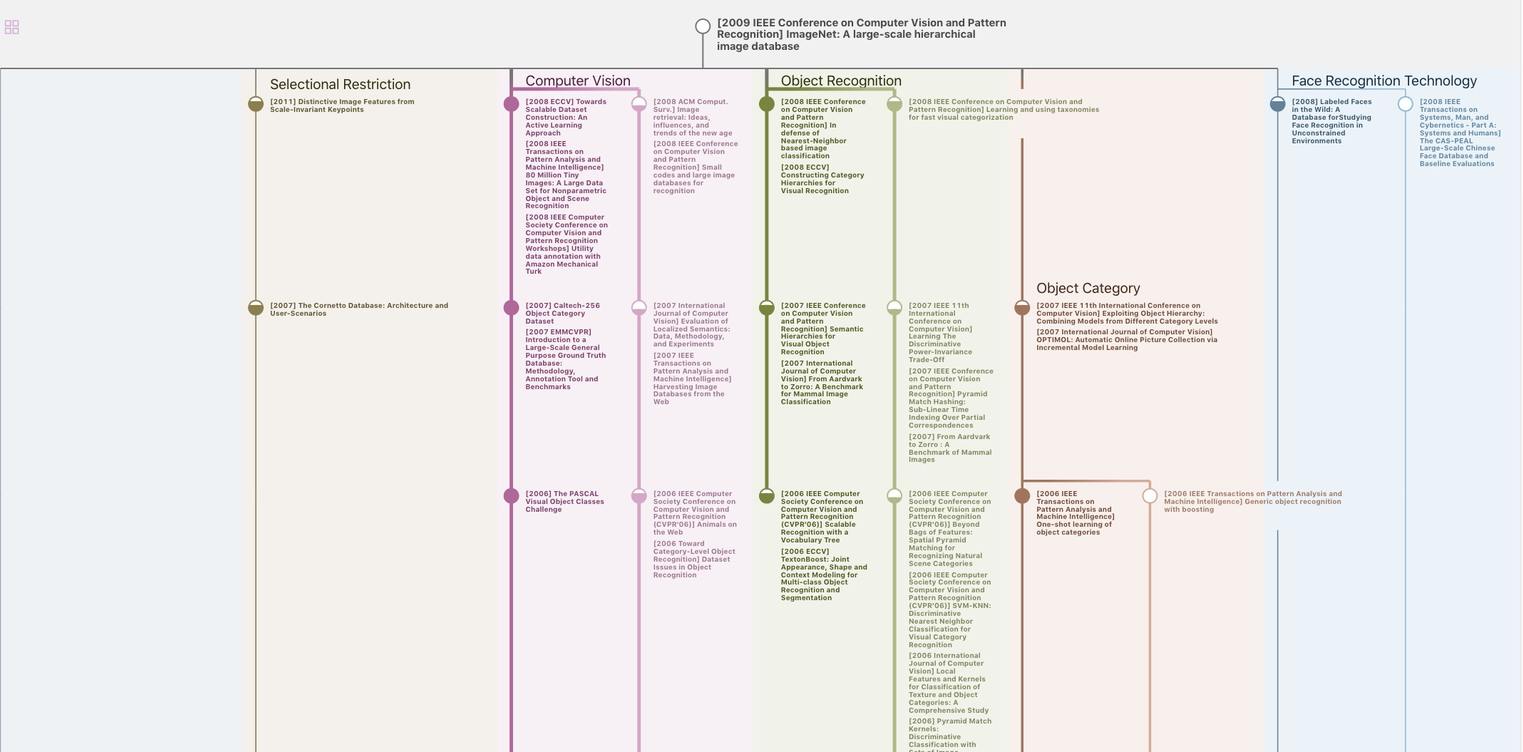
生成溯源树,研究论文发展脉络
Chat Paper
正在生成论文摘要