Evaluating the Impact of Algorithm Confidence Ratings on Human Decision Making in Visual Search
HCI (4)(2021)
摘要
As the ability to collect and store data grows, so does the need to efficiently analyze that data. As human-machine teams that use machine learning (ML) algorithms as a way to inform human decision-making grow in popularity it becomes increasingly critical to understand the optimal methods of implementing algorithm assisted search. In order to better understand how algorithm confidence values associated with object identification can influence participant accuracy and response times during a visual search task, we compared models that provided appropriate confidence, random confidence, and no confidence, as well as a model biased toward over confidence and a model biased toward under confidence. Results indicate that randomized confidence is likely harmful to performance while non-random confidence values are likely better than no confidence value for maintaining accuracy over time. Providing participants with appropriate confidence values did not seem to benefit performance any more than providing participants with under or over confident models.
更多查看译文
关键词
Visual search,Human-machine teaming,Cognition
AI 理解论文
溯源树
样例
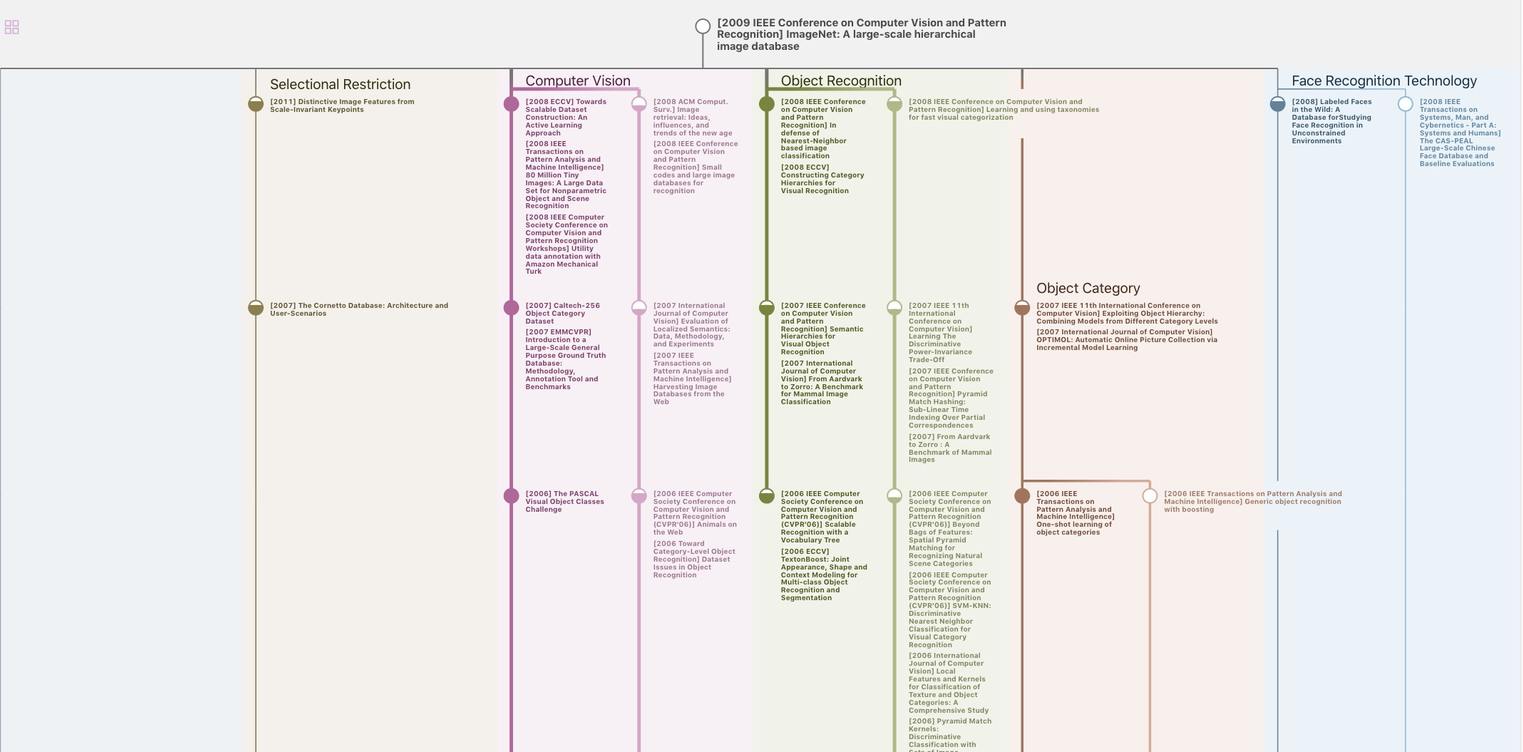
生成溯源树,研究论文发展脉络
Chat Paper
正在生成论文摘要