On the Performance of Planning Through Backpropagation.
BRACIS (2)(2020)
摘要
Planning problems with continuous state and action spaces are difficult to solve with existing planning techniques, specially when the state transition is defined by a high-dimension non-linear dynamics. Recently, a technique called Planning through Backpropagation (PtB) was introduced as an efficient and scalable alternative to traditional optimization-based methods for continuous planning problems. PtB leverages modern gradient descent algorithms and highly optimized automatic differentiation libraries to obtain approximate solutions. However, to date there have been no empirical evaluations comparing PtB with Linear-Quadratic (LQ) control problems. In this work, we compare PtB with an optimal algorithm from control theory called LQR, and its iterative version iLQR, when solving linear and non-linear continuous deterministic planning problems. The empirical results suggest that PtB can be an efficient alternative to optimizing non-linear continuous deterministic planning, being much easier to be implemented and stabilized than classical model-predictive control methods.
更多查看译文
关键词
Gradient based optimization, Deep learning, Continuous deterministic planning
AI 理解论文
溯源树
样例
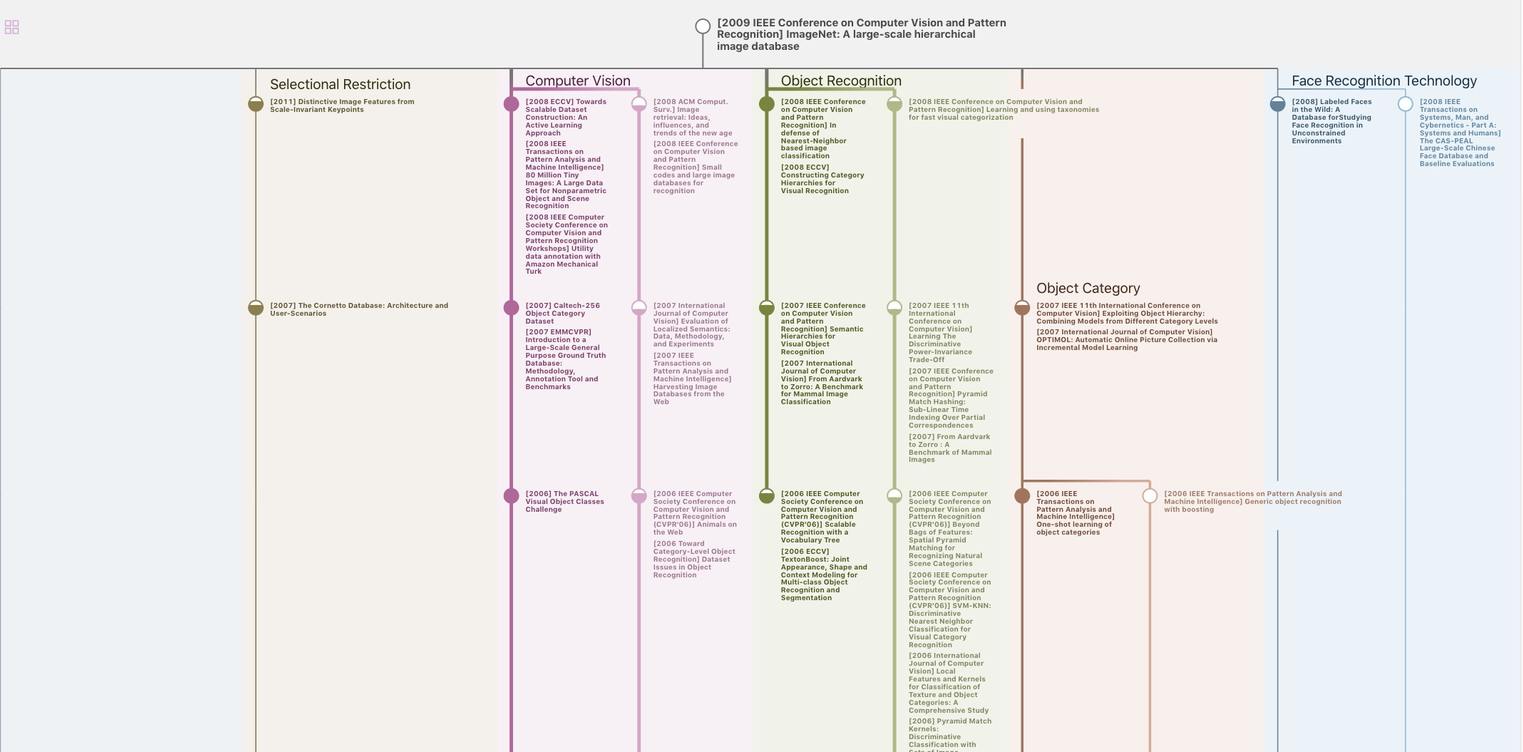
生成溯源树,研究论文发展脉络
Chat Paper
正在生成论文摘要