Unsupervised Domain Adaptation with Unified Joint Distribution Alignment.
DASFAA (2)(2021)
摘要
Unsupervised domain adaptation aims at transferring knowledge from a labeled source domain to an unlabeled target domain. Recently, domain-adversarial learning has become an increasingly popular method to tackle this task, which bridges the source domain and target domain by adversarially learning domain-invariant representations. Despite the great success in domain-adversarial learning, these methods fail to achieve the invariance of representations at a class level, which may lead to incorrect distribution alignment. To address this problem, in this paper, we propose a method called domain adaptation with Unified Joint Distribution Alignment (UJDA) to perform both domain-level and class-level alignments simultaneously in a unified learning process. Instead of adopting the classical domain discriminator, two novel components named joint classifiers, which are provided with both domain information and label information in both domains, are adopted in UJDA. Single joint classifier plays the min-max game with the feature extractor by the joint adversarial loss to align the class-level alignment. Besides, two joint classifiers as a whole also play the min-max game with the feature extractor by the disagreement loss to achieve the domain-level alignment. Comprehensive experiments on two realworld datasets verify that our method outperforms several state-of-the-art domain adaptation methods.
更多查看译文
关键词
Transfer learning,Domain adaptation,Distribution alignment
AI 理解论文
溯源树
样例
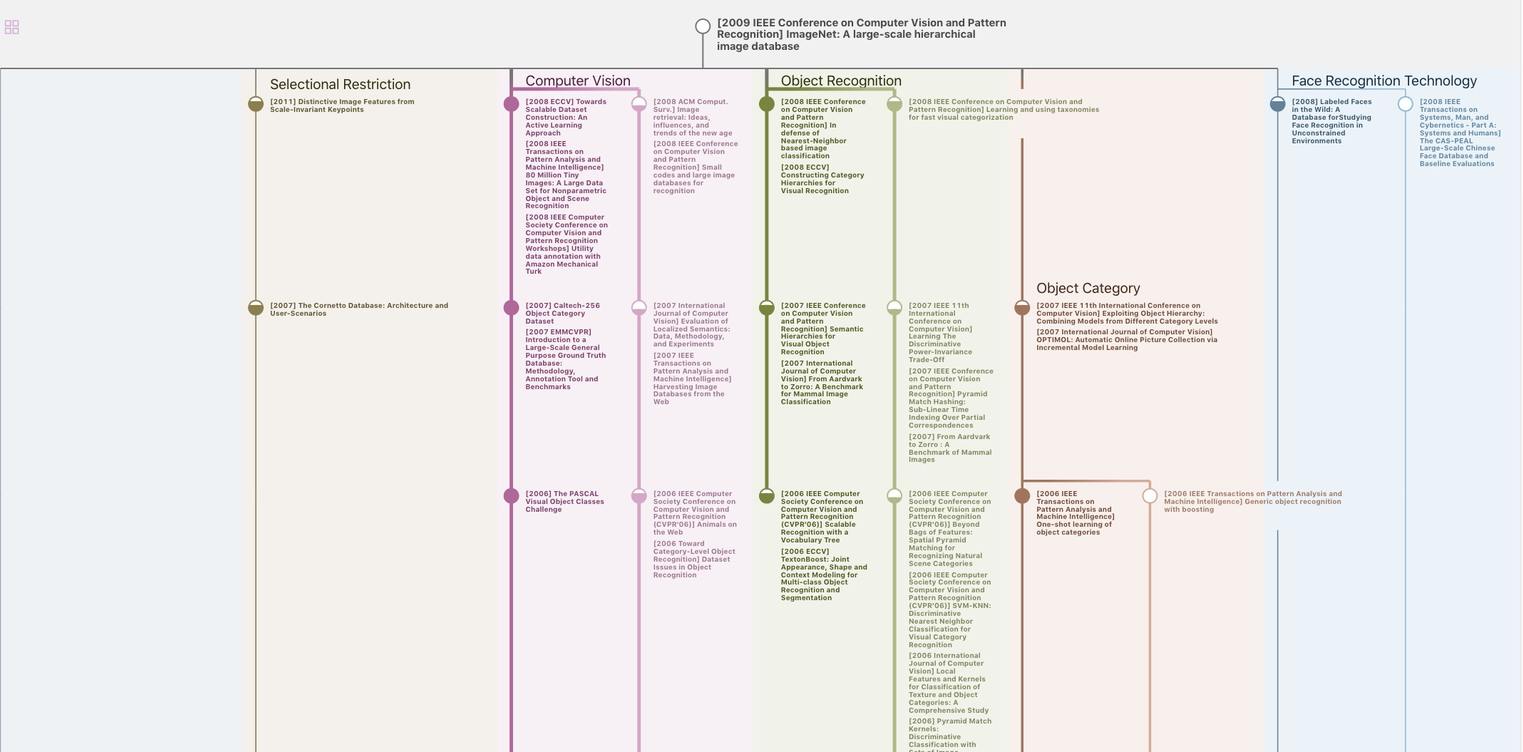
生成溯源树,研究论文发展脉络
Chat Paper
正在生成论文摘要