Generative Image Inpainting by Hybrid Contextual Attention Network.
MMM (1)(2021)
摘要
Image inpainting is a challenging task due to the loss of the image information. Recently, GAN-based approaches have shown promising performance in the field of image inpainting. For this task, a superior similarity measurement of extracted patches from known and missing regions is important. Existing approaches usually adopt cosine distance to measure this similarity for missing region reconstruction. However, from the semantic-level perspective, these methods often generate content with inconsistent color and disorder structure due to the ignorance of the magnitude distance of the attended patches. To resolve this problem, we propose a Hybrid Contextual Attention Network (HCA-Net) with a novel attention module called hybrid contextual attention module (HCAM). HCAM takes account of both cosine distance and Euclidean distance as the measurement of the extracted patches and gives a better prediction of missing features. Besides, a Spectral-Normalization patch discriminator and the cosine loss are added into the model for patch-level and pixel-level consistency enhancement. Extensive results on three public datasets (Paris Street View, Celeba-HQ, and Places2), have both validated that our approach significantly outperforms the state-of-the-art approaches.
更多查看译文
关键词
Image inpainting, HCA-Net, HCAM
AI 理解论文
溯源树
样例
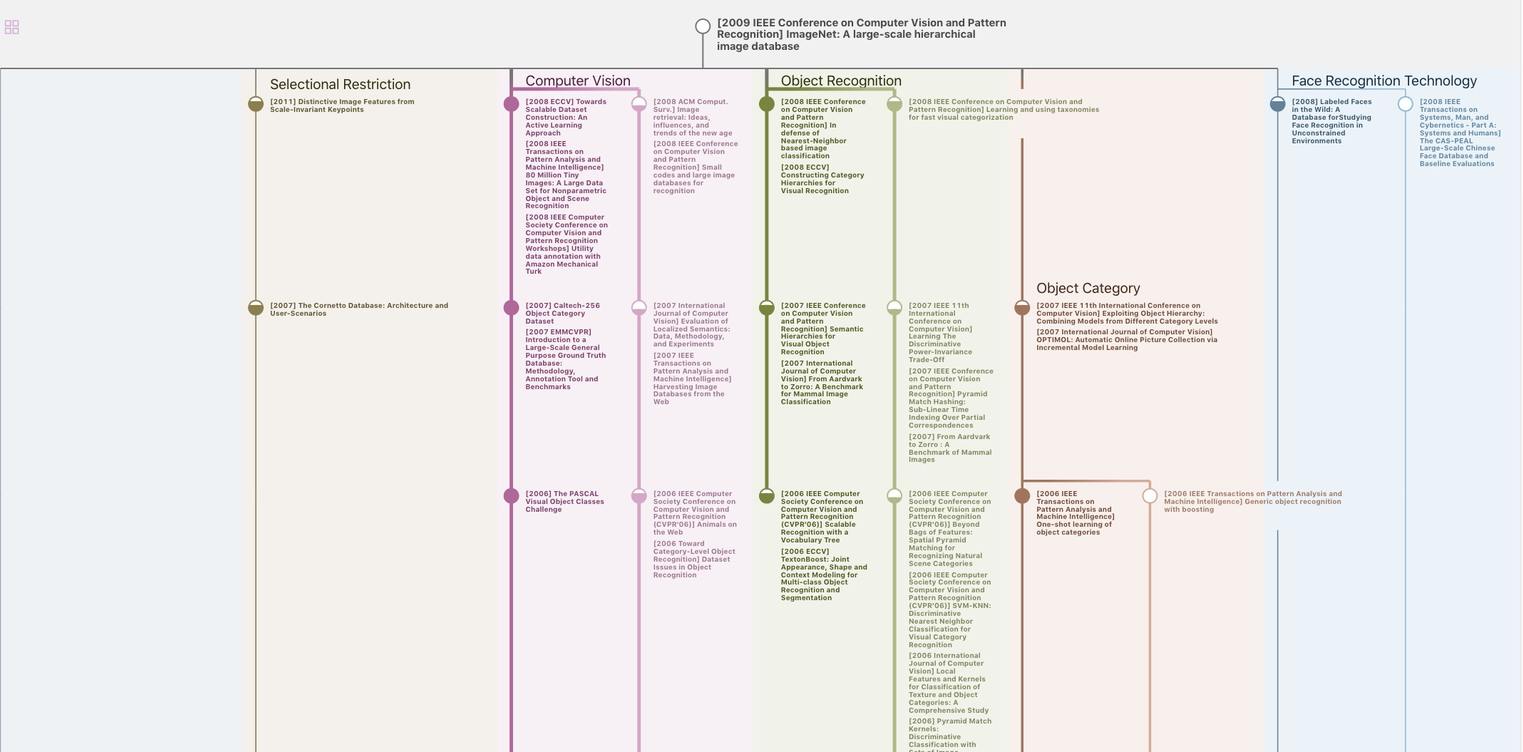
生成溯源树,研究论文发展脉络
Chat Paper
正在生成论文摘要