Covariate Shift in High-Dimensional Random Feature Regression
arxiv(2021)
摘要
A significant obstacle in the development of robust machine learning models is covariate shift, a form of distribution shift that occurs when the input distributions of the training and test sets differ while the conditional label distributions remain the same. Despite the prevalence of covariate shift in real-world applications, a theoretical understanding in the context of modern machine learning has remained lacking. In this work, we examine the exact high-dimensional asymptotics of random feature regression under covariate shift and present a precise characterization of the limiting test error, bias, and variance in this setting. Our results motivate a natural partial order over covariate shifts that provides a sufficient condition for determining when the shift will harm (or even help) test performance. We find that overparameterized models exhibit enhanced robustness to covariate shift, providing one of the first theoretical explanations for this intriguing phenomenon. Additionally, our analysis reveals an exact linear relationship between in-distribution and out-of-distribution generalization performance, offering an explanation for this surprising recent empirical observation.
更多查看译文
关键词
covariate shift,regression,high-dimensional
AI 理解论文
溯源树
样例
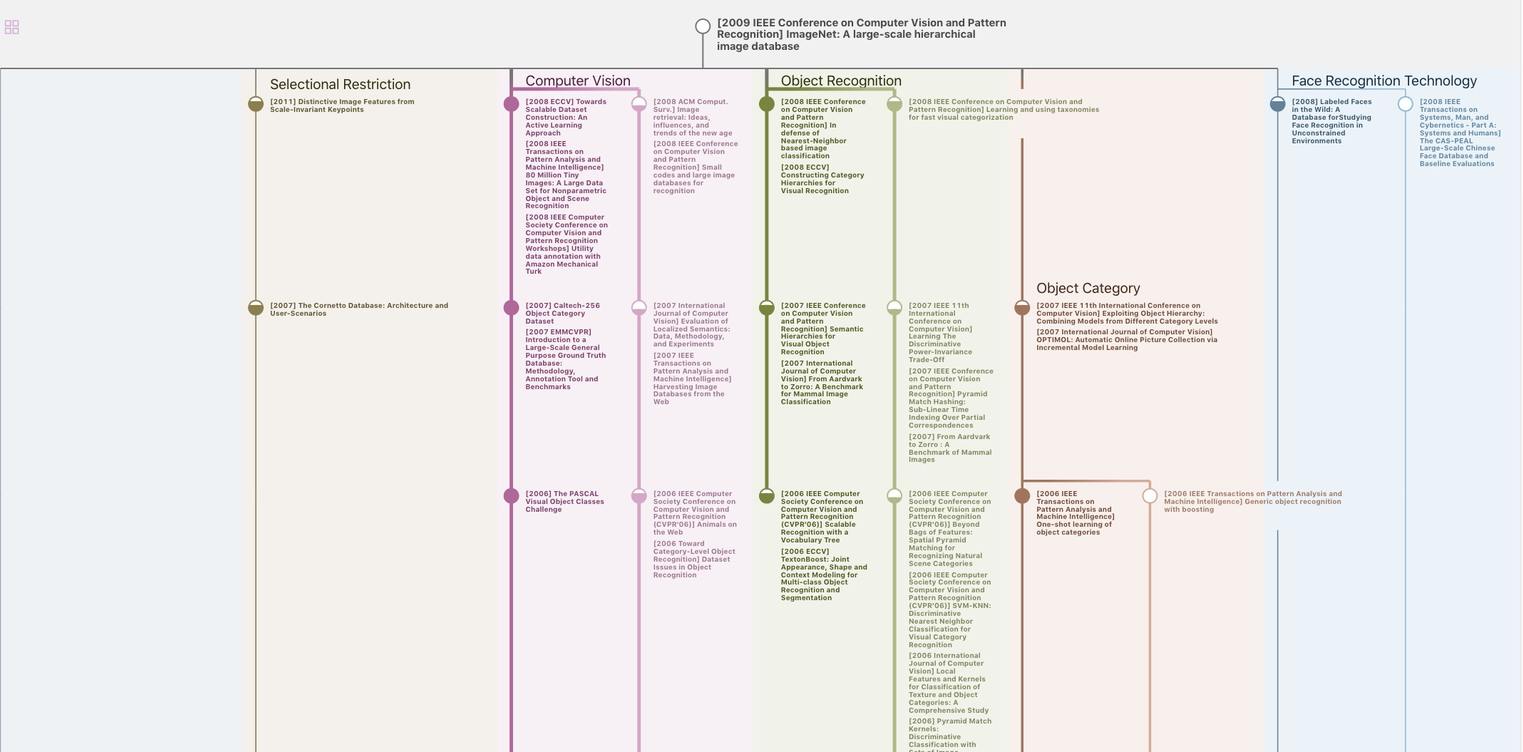
生成溯源树,研究论文发展脉络
Chat Paper
正在生成论文摘要