Will We Trust What We Don't Understand? Impact of Model Interpretability and Outcome Feedback on Trust in AI
arxiv(2021)
摘要
Despite AI's superhuman performance in a variety of domains, humans are often unwilling to adopt AI systems. The lack of interpretability inherent in many modern AI techniques is believed to be hurting their adoption, as users may not trust systems whose decision processes they do not understand. We investigate this proposition with a novel experiment in which we use an interactive prediction task to analyze the impact of interpretability and outcome feedback on trust in AI and on human performance in AI-assisted prediction tasks. We find that interpretability led to no robust improvements in trust, while outcome feedback had a significantly greater and more reliable effect. However, both factors had modest effects on participants' task performance. Our findings suggest that (1) factors receiving significant attention, such as interpretability, may be less effective at increasing trust than factors like outcome feedback, and (2) augmenting human performance via AI systems may not be a simple matter of increasing trust in AI, as increased trust is not always associated with equally sizable improvements in performance. These findings invite the research community to focus not only on methods for generating interpretations but also on techniques for ensuring that interpretations impact trust and performance in practice.
更多查看译文
关键词
model interpretability,trust,ai,feedback
AI 理解论文
溯源树
样例
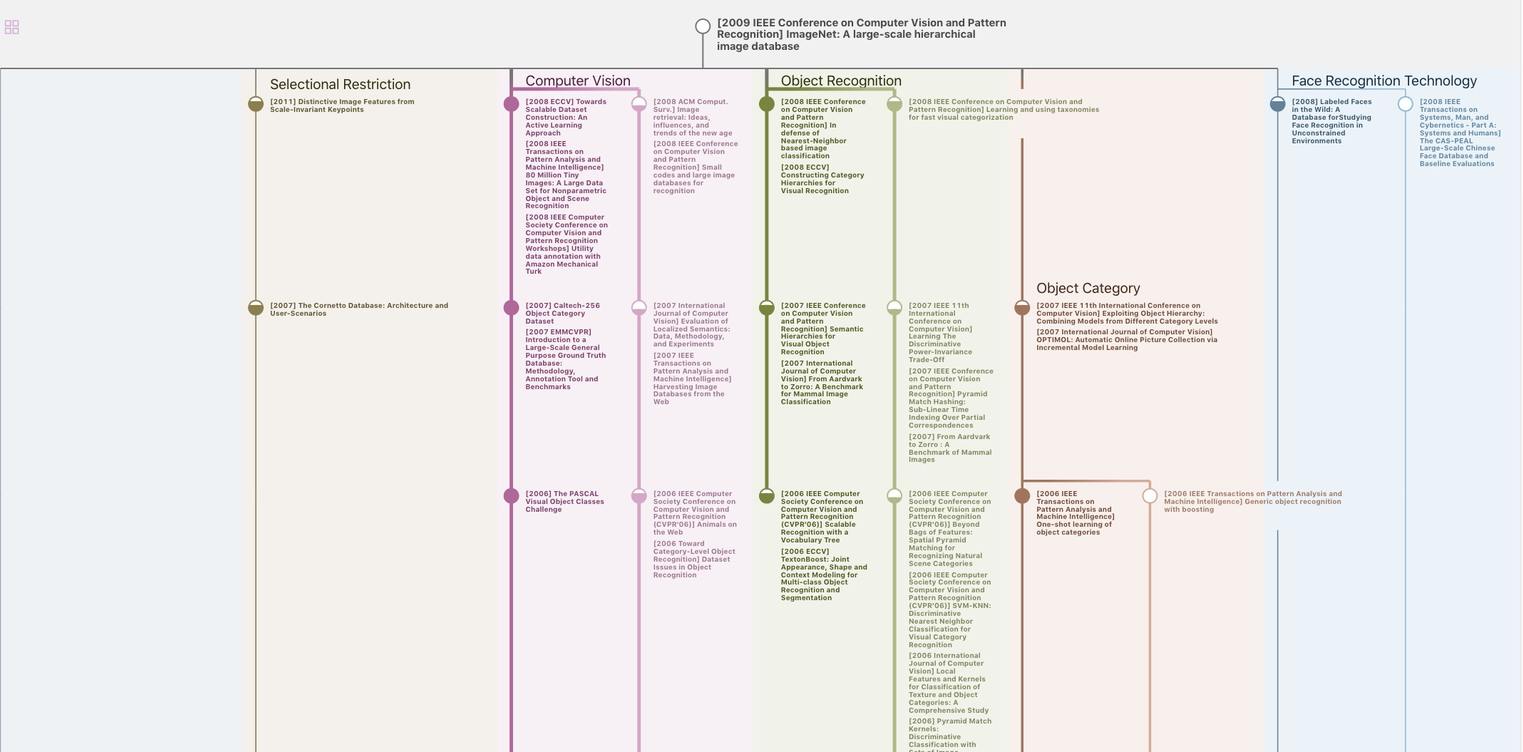
生成溯源树,研究论文发展脉络
Chat Paper
正在生成论文摘要