FILIP: Fine-grained Interactive Language-Image Pre-Training.
International Conference on Learning Representations (ICLR)(2022)
摘要
Unsupervised large-scale vision-language pre-training has shown promising advances on various downstream tasks. Existing methods often model the cross-modal interaction either via the similarity of the global feature of each modality which misses sufficient information, or finer-grained interactions using cross/self-attention upon visual and textual tokens. However, cross/self-attention suffers from inferior efficiency in both training and inference. In this paper, we introduce a large-scale Fine-grained Interactive Language-Image Pre-training (FILIP) to achieve finer-level alignment through a cross-modal late interaction mechanism, which uses a token-wise maximum similarity between visual and textual tokens to guide the contrastive objective. FILIP successfully leverages the finer-grained expressiveness between image patches and textual words by modifying only contrastive loss, while simultaneously gaining the ability to pre-compute image and text representations offline at inference, keeping both large-scale training and inference efficient. Furthermore, we construct a new large-scale image-text pair dataset called FILIP300M for pre-training. Experiments show that FILIP achieves state-of-the-art performance on multiple downstream vision-language tasks including zero-shot image classification and image-text retrieval. The visualization on word-patch alignment further shows that FILIP can learn meaningful fine-grained features with promising localization ability.
更多查看译文
关键词
Visual-language pretraining,Language-Image Pretraining,Multi-modality model
AI 理解论文
溯源树
样例
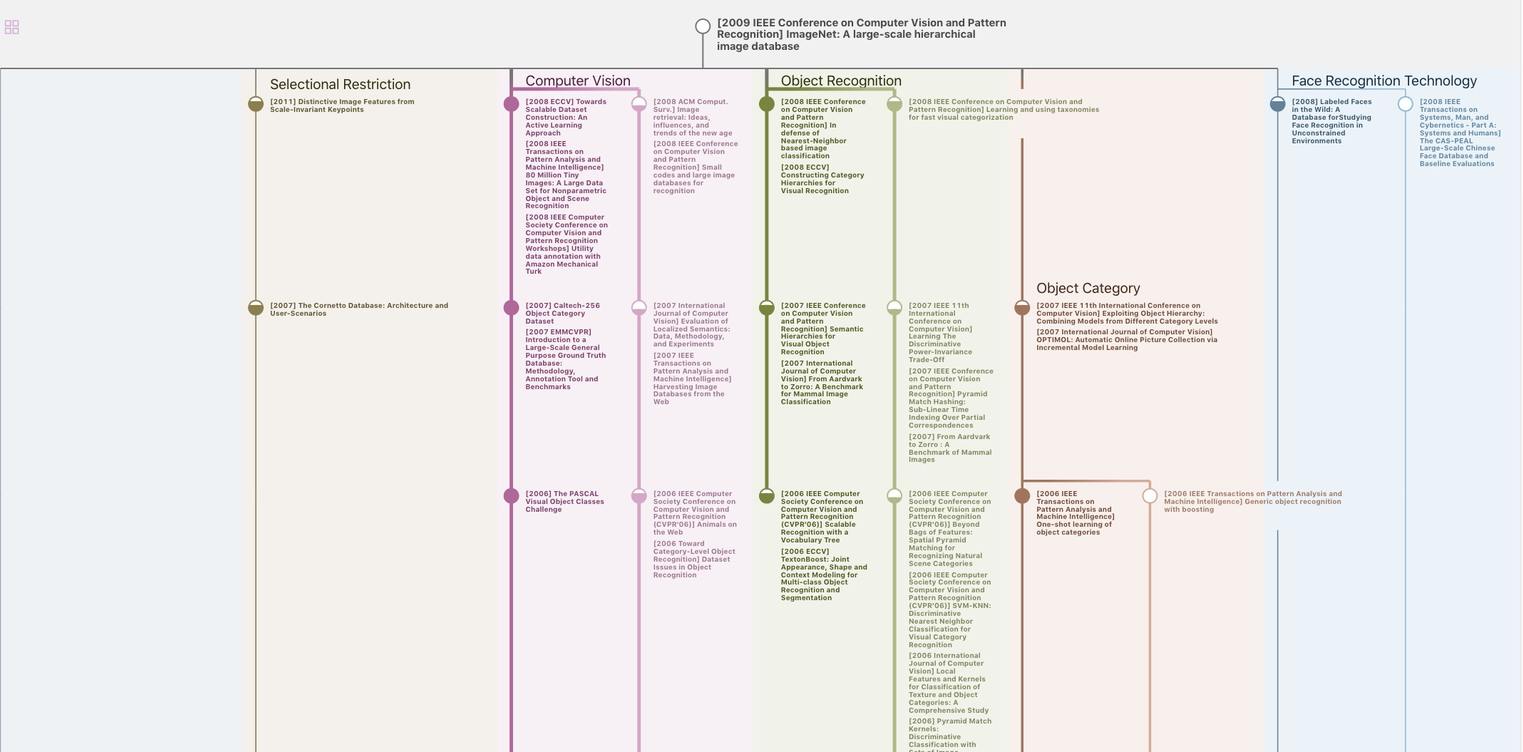
生成溯源树,研究论文发展脉络
Chat Paper
正在生成论文摘要