Towards Interpretability of Speech Pause in Dementia Detection Using Adversarial Learning.
IEEE International Conference on Acoustics, Speech, and Signal Processing (ICASSP)(2022)
摘要
Speech pause is an effective biomarker in dementia detection. Recent deep learning models have exploited speech pauses to achieve highly accurate dementia detection, but have not exploited the interpretability of speech pauses, i.e., what and how positions and lengths of speech pauses affect the result of dementia detection. In this paper, we will study the positions and lengths of dementia-sensitive pauses using adversarial learning approaches. Specifically, we first utilize an adversarial attack approach by adding the perturbation to the speech pauses of the testing samples, aiming to reduce the confidence levels of the detection model. Then, we apply an adversarial training approach to evaluate the impact of the perturbation in training samples on the detection model. We examine the interpretability from the perspectives of model accuracy, pause context, and pause length. We found that some pauses are more sensitive to dementia than other pauses from the model's perspective, e.g., speech pauses near to the verb "is". Increasing lengths of sensitive pauses or adding sensitive pauses leads the model inference to Alzheimer's Disease, while decreasing the lengths of sensitive pauses or deleting sensitive pauses leads to non-AD.
更多查看译文
关键词
Speech pauses,acoustic feature,dementia detection,interpretability,spontaneous speech
AI 理解论文
溯源树
样例
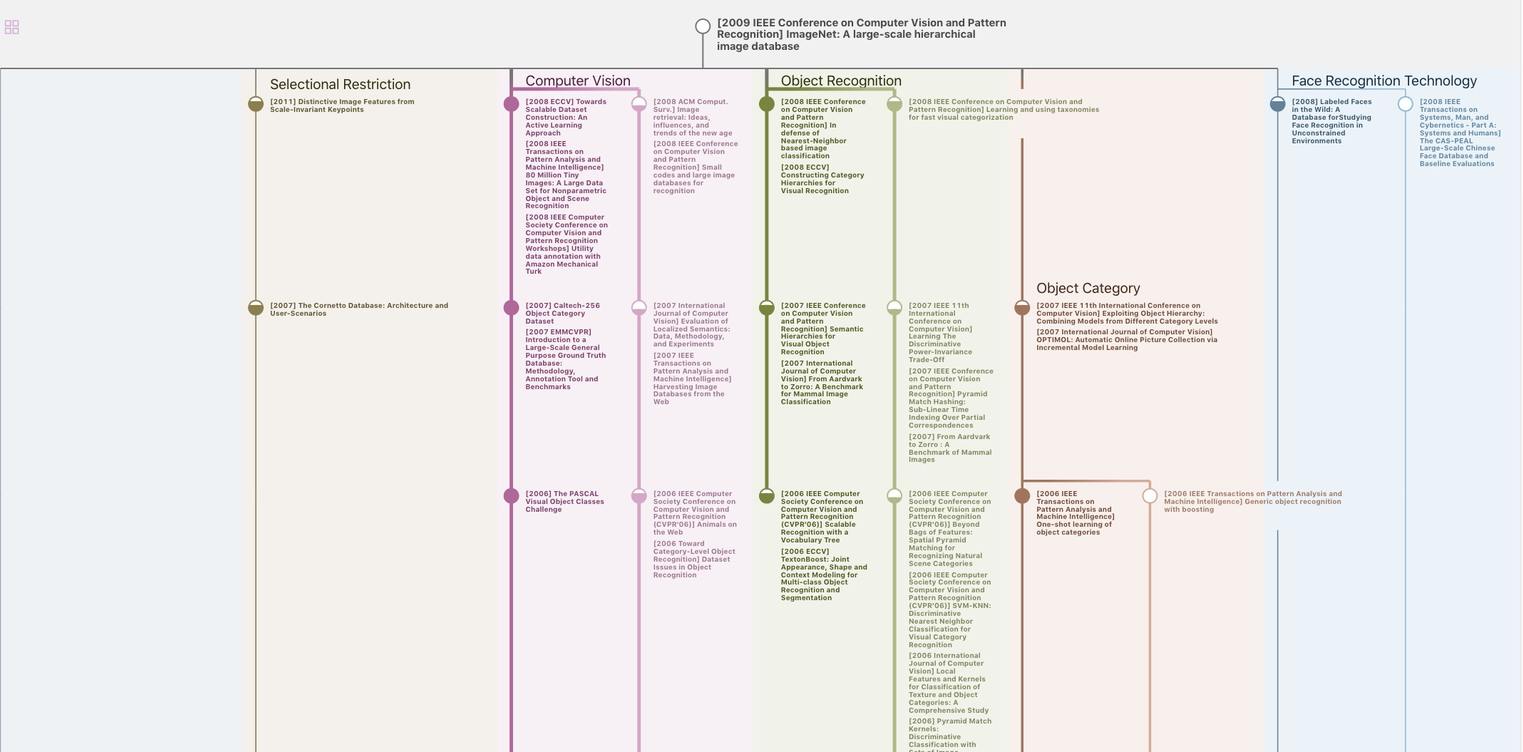
生成溯源树,研究论文发展脉络
Chat Paper
正在生成论文摘要