Evaluating Contrastive Learning on Wearable Timeseries for Downstream Clinical Outcomes
arxiv(2021)
摘要
Vast quantities of person-generated health data (wearables) are collected but the process of annotating to feed to machine learning models is impractical. This paper discusses ways in which self-supervised approaches that use contrastive losses, such as SimCLR and BYOL, previously applied to the vision domain, can be applied to high-dimensional health signals for downstream classification tasks of various diseases spanning sleep, heart, and metabolic conditions. To this end, we adapt the data augmentation step and the overall architecture to suit the temporal nature of the data (wearable traces) and evaluate on 5 downstream tasks by comparing other state-of-the-art methods including supervised learning and an adversarial unsupervised representation learning method. We show that SimCLR outperforms the adversarial method and a fully-supervised method in the majority of the downstream evaluation tasks, and that all self-supervised methods outperform the fully-supervised methods. This work provides a comprehensive benchmark for contrastive methods applied to the wearable time-series domain, showing the promise of task-agnostic representations for downstream clinical outcomes.
更多查看译文
关键词
wearable timeseries,contrastive learning,clinical outcomes
AI 理解论文
溯源树
样例
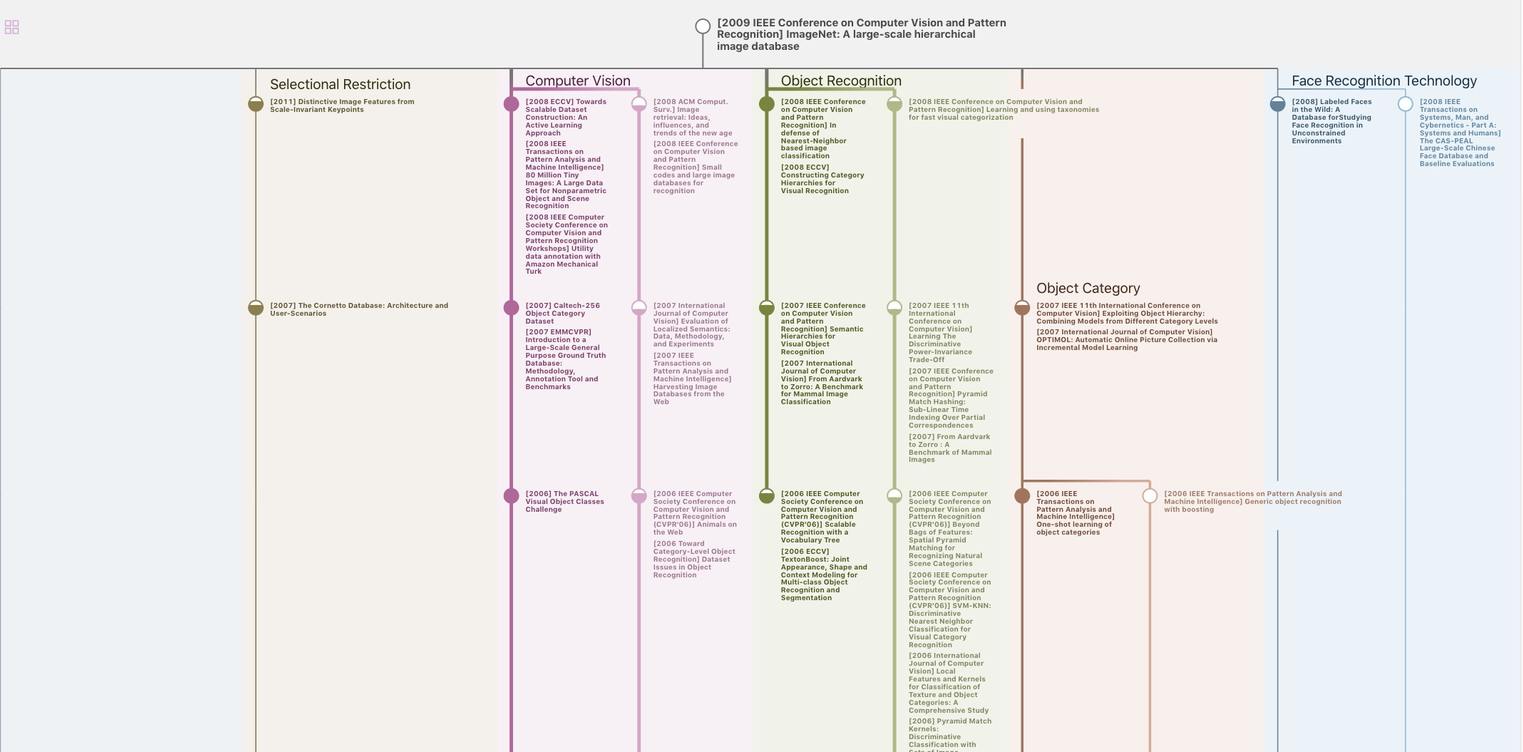
生成溯源树,研究论文发展脉络
Chat Paper
正在生成论文摘要