PAMMELA: Policy Administration Methodology using Machine Learning
SECRYPT : PROCEEDINGS OF THE 19TH INTERNATIONAL CONFERENCE ON SECURITY AND CRYPTOGRAPHY(2022)
摘要
In recent years, Attribute-Based Access Control (ABAC) has become quite popular and effective for enforcing access control in dynamic and collaborative environments. Implementation of ABAC requires the creation of a set of attribute-based rules which cumulatively form a policy. Designing an ABAC policy ab initio demands a substantial amount of effort from the system administrator. Moreover, organizational changes may necessitate the inclusion of new rules in an already deployed policy. In such a case, re-mining the entire ABAC policy requires a considerable amount of time and administrative effort. Instead, it is better to incrementally augment the policy. In this paper, we propose PAMMELA, a Policy Administration Methodology using Machine Learning to assist system administrators in creating new ABAC policies as well as augmenting existing policies. PAMMELA can generate a new policy for an organization by learning the rules of a policy currently enforced in a similar organization. For policy augmentation, new rules are inferred based on the knowledge gathered from the existing rules. A detailed experimental evaluation shows that the proposed approach is both efficient and effective.
更多查看译文
关键词
ABAC, Policy Administration, Policy Augmentation, Policy Adaptation, Supervised Learning
AI 理解论文
溯源树
样例
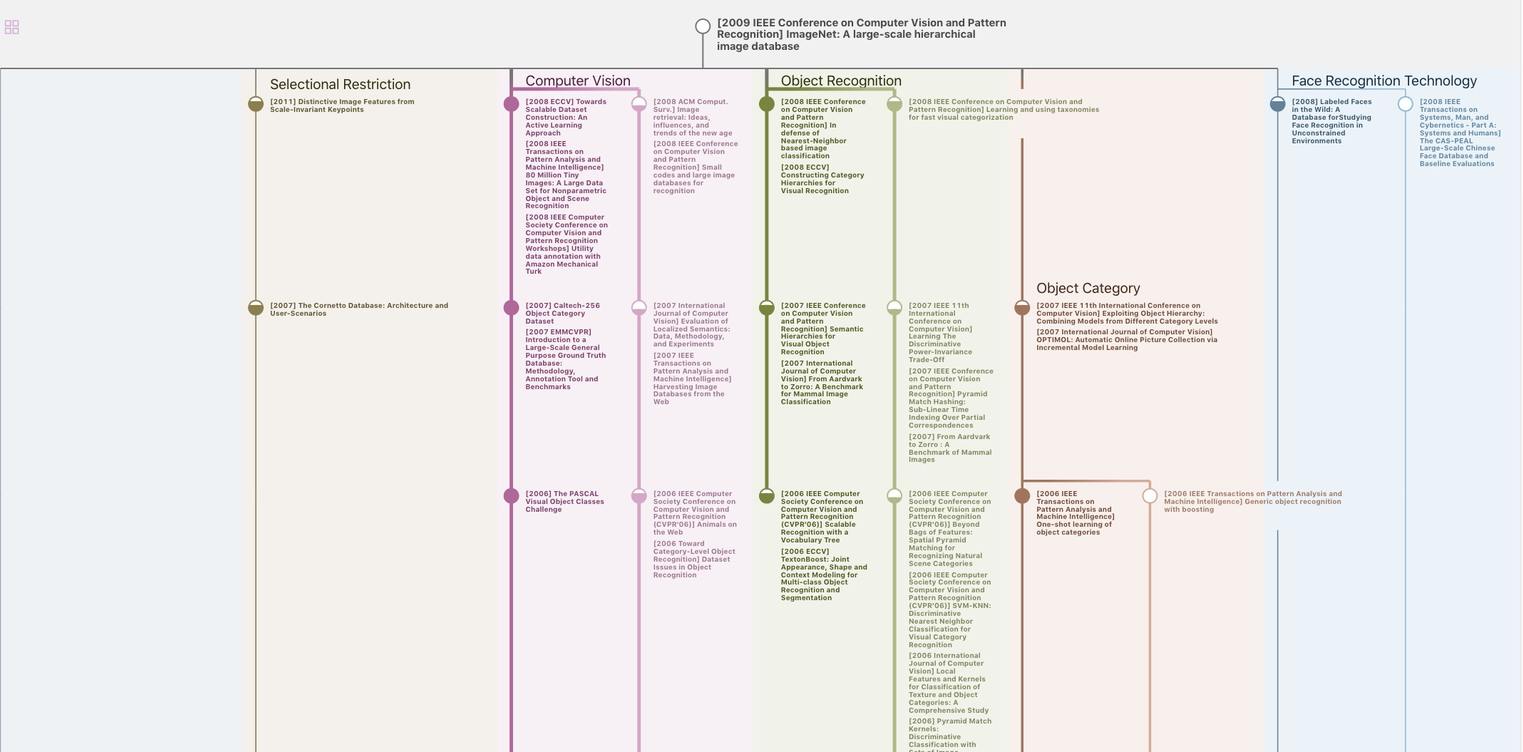
生成溯源树,研究论文发展脉络
Chat Paper
正在生成论文摘要