A Robust Deep Learning-Based Beamforming Design for RIS-Assisted Multiuser MISO Communications With Practical Constraints
IEEE Transactions on Cognitive Communications and Networking(2022)
摘要
Reconfigurable intelligent surface (RIS) has become a promising technology to improve wireless communication in recent years. It steers the incident signals to create a favorable propagation environment by controlling the reconfigurable passive elements with less hardware cost and lower power consumption. In this paper, we consider a RIS-aided multiuser multiple-input single-output downlink communication system. We aim to maximize the weighted sum-rate of all users by joint optimizing the active beamforming at the access point and the passive beamforming vector of the RIS elements. Unlike most existing works, we consider the more practical situation with the discrete phase shifts and imperfect channel state information (CSI). Specifically, for the situation that the discrete phase shifts and perfect CSI are considered, we first develop a deep quantization neural network (DQNN) to simultaneously design the active and passive beamforming while most reported works design them alternatively. Then, we propose an improved structure (I-DQNN) based on DQNN to simplify the parameters decision process when the control bits of each RIS element are greater than 1 bit. Finally, we extend the two proposed DQNN-based algorithms to the case that the discrete phase shifts and imperfect CSI are considered simultaneously. Our simulation results show that the two DQNN-based algorithms have better performance than traditional algorithms in the perfect CSI case, and are also more robust in the imperfect CSI case.
更多查看译文
关键词
Reconfigurable intelligent surface,joint beamforming,deep learning,quantization layer,discrete phase shifts,imperfect CSI
AI 理解论文
溯源树
样例
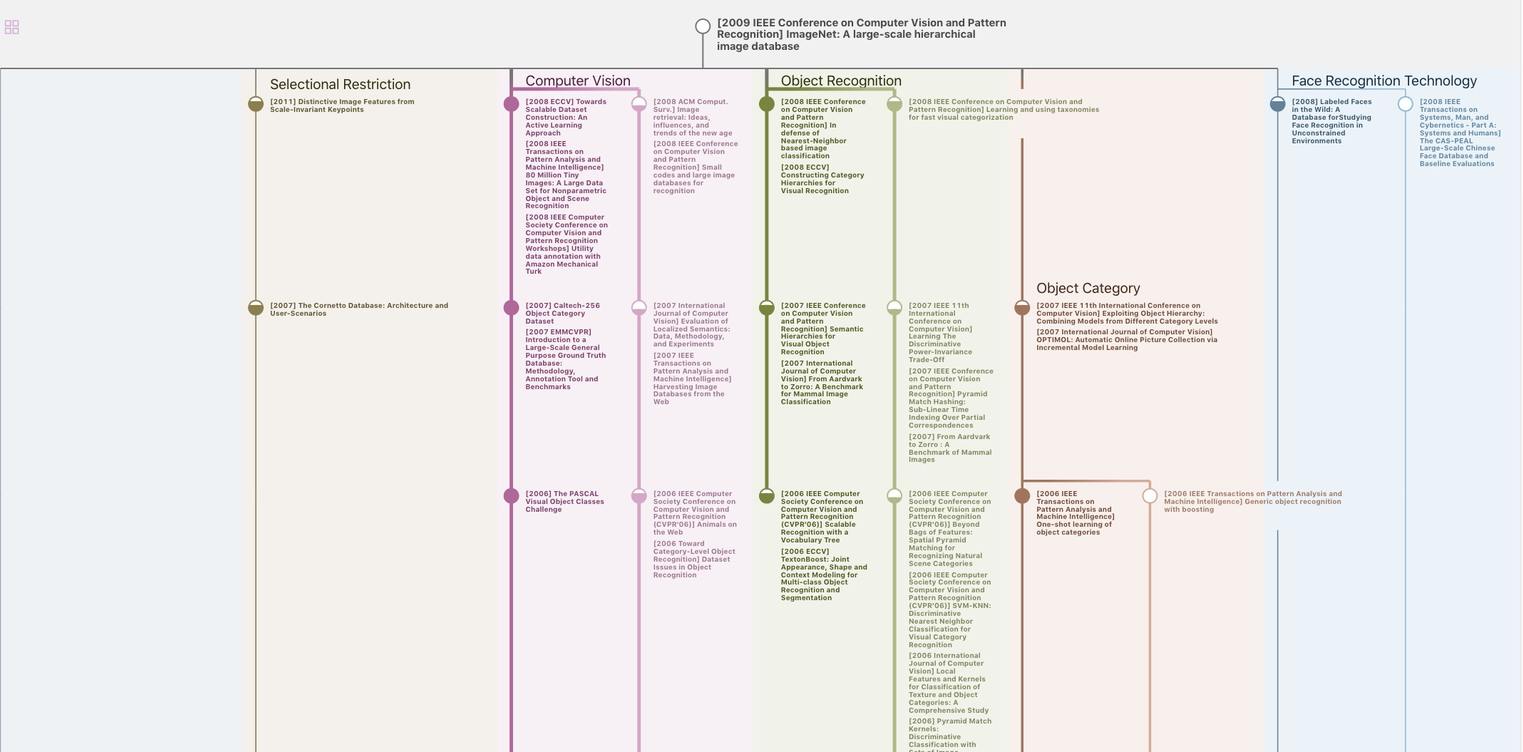
生成溯源树,研究论文发展脉络
Chat Paper
正在生成论文摘要