Dynamic Iterative Refinement for Efficient 3D Hand Pose Estimation
2022 IEEE WINTER CONFERENCE ON APPLICATIONS OF COMPUTER VISION (WACV 2022)(2022)
摘要
While hand pose estimation is a critical component of most interactive extended reality and gesture recognition systems, contemporary approaches are not optimized for computational and memory efficiency. In this paper; we propose a tiny deep neural network of which partial layers are recursively exploited for refining its previous estimations. During its iterative refinements, we employ learned gating criteria to decide whether to exit from the weight-sharing loop, allowing per-sample adaptation in our model. Our network is trained to be aware of the uncertainty in its current predictions to efficiently gate at each iteration, estimating variances after each loop for its keypoint estimates. Additionally, we investigate the effectiveness of end-to-end and progressive training protocols for our recursive structure on maximizing the model capacity. With the proposed setting, our method consistently outperforms state-of-the-art 2D/3D hand pose estimation approaches in terms of both accuracy and efficiency for widely used benchmarks.
更多查看译文
关键词
dynamic iterative refinement,hand,3d,estimation
AI 理解论文
溯源树
样例
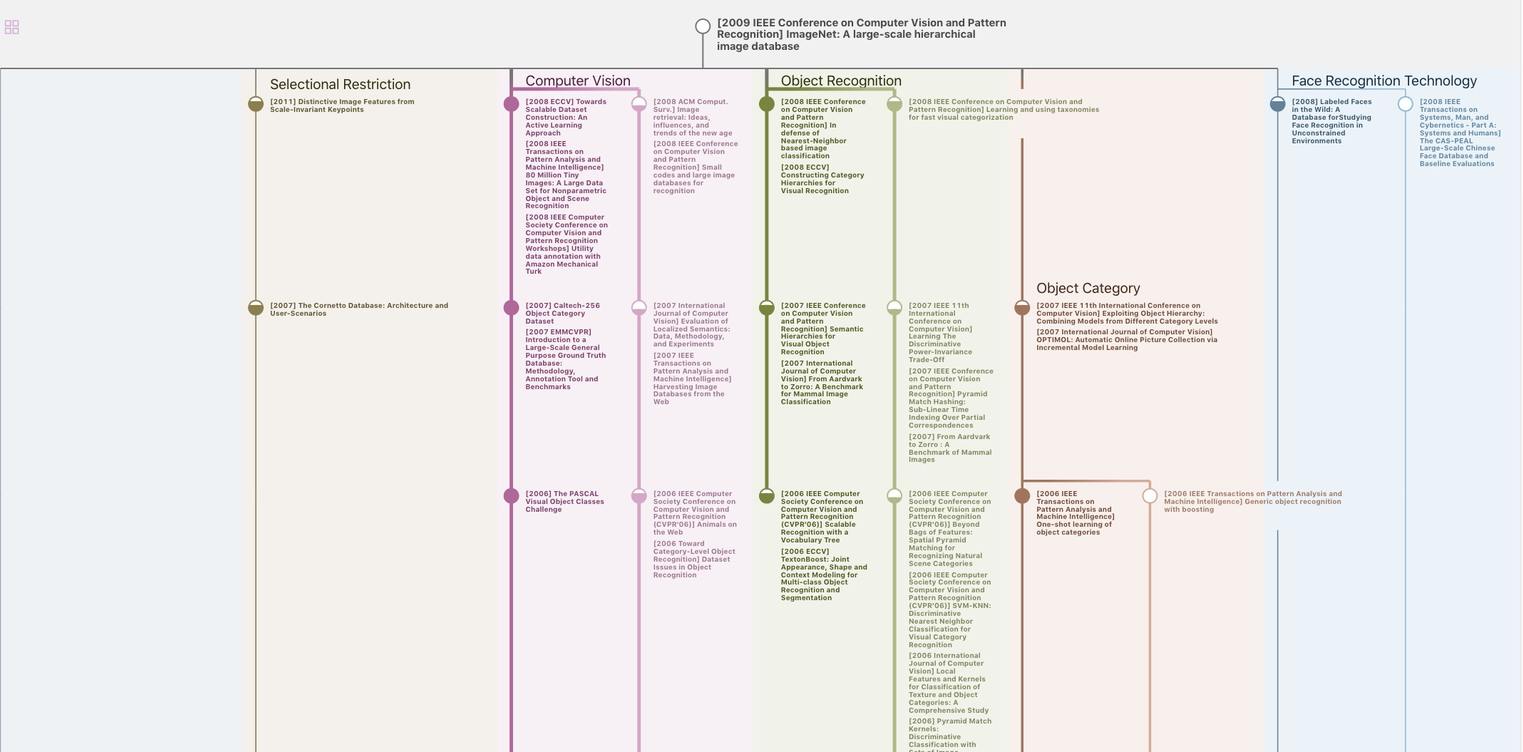
生成溯源树,研究论文发展脉络
Chat Paper
正在生成论文摘要